9. AI vs. Automation – Distinguishing and combining both
This post clarifies the key differences between AI and automation, illustrates how combining them can produce maximum impact, and offers practical use cases that SMEs can implement. By the end, you’ll understand the distinct roles of AI (the “brain”) and automation (the “hands”) and how, used together, they can revolutionize workflows across industries.
Q1: FOUNDATIONS OF AI IN SME MANAGEMENT - CHAPTER 1 (DAYS 1–31): CORE AI CONCEPTS & VALUE PROPOSITION
Gary Stoyanov PhD
1/9/20256 min read
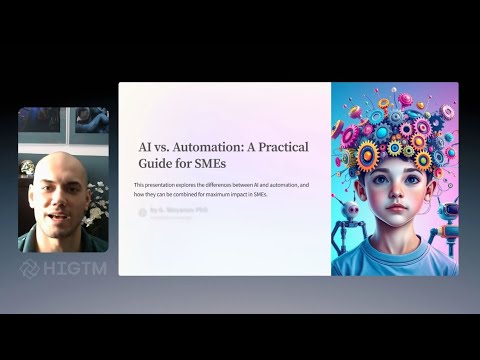
1. Defining AI & Automation
Before exploring synergies, it’s crucial to understand each concept independently. While automation centers on performing tasks without continuous human oversight, AI focuses on systems that learn, reason, and improve based on data.
1.1 Artificial Intelligence (AI)
Definition: AI refers to computer systems capable of learning, reasoning, or decision-making, often relying on algorithms and large datasets.
Core Activities:
Pattern Recognition: Identifying anomalies in transactions or trends in user behavior.
Prediction & Forecasting: Projecting future sales, maintenance requirements, or market shifts.
Adaptation Over Time: Refining outputs (recommendations, decisions) as new data arrives.
Examples:
Fraud Detection: Machine learning models pinpoint unusual spending patterns.
Demand Forecasting: Predicting seasonal surges for inventory management.
Chatbots: Understanding natural language inquiries and providing context-aware responses.
Key Distinction: AI systems don’t just execute a pre-set series of steps; they analyze patterns and adapt output based on data inputs. When properly trained, AI can go beyond basic rules, handling complex scenarios or even discovering new approaches no human explicitly programmed.
1.2 Automation
Definition: Automation leverages technology—scripts, robotics, or software workflows—to execute repetitive or rule-based tasks without requiring continuous human involvement.
Core Activities:
Rule Execution: Handling routine data entry, email filtering, or scripted responses.
Consistency & Speed: Carrying out tasks reliably with minimal errors.
Limited Flexibility: Does not inherently learn new methods; it follows established rules.
Examples:
Invoice Processing: Automated scripts send reminders or update payment statuses.
Email Responses: Pre-set triggers deliver relevant forms or replies to common queries.
Manufacturing Lines: Robotics handle repetitive assembly tasks with little variation.
Key Distinction: Automation excels at predictable tasks that follow a straightforward, repeatable sequence. If conditions change significantly or an unforeseen event occurs, automated systems typically need human intervention or reprogramming to cope.
2. Key Differences Between AI & Automation
While both AI and automation reduce manual workloads, each tackles challenges in distinct ways. Recognizing these differences helps you pick the right tool for your specific operational bottlenecks.
2.1 Complexity & Learning
Automation: Operates on fixed rules—what you program in is exactly what you get out. If conditions shift, you must manually update the logic or the entire workflow.
AI: Learns from data and refines its output. For example, a machine learning model can automatically adjust fraud detection patterns as criminals develop new tactics, without being explicitly reprogrammed each time.
Takeaway: If your process rarely changes (like emailing monthly invoices), automation suffices. For dynamic scenarios (like adapting marketing campaigns to trending topics), AI’s learning ability is indispensable.
2.2 Scope of Tasks
Automation: Suited to tasks with strict, repeatable sequences—like data entry or simple notifications—where variation is minimal.
AI: Addresses more complex, uncertain tasks—such as anomaly detection, advanced language interpretation, or pattern recognition—where data-driven reasoning matters.
Takeaway: Do your workflows differ day-to-day or require nuanced judgment? AI might be a fit. Are you dealing with high-volume, repetitive tasks? Automation is perfect.
2.3 Human Oversight
Automation: Runs autonomously once set up; but if unexpected situations arise, it lacks intelligence to adapt on its own.
AI: May operate independently but benefits from ongoing oversight, as models degrade or data drifts over time. Periodic retraining and performance checks keep AI fresh and relevant.
Takeaway: AI typically demands iterative maintenance—updating data sets, fine-tuning parameters—while automation can be a “set and run” approach, though it must be updated if the task’s logic changes.
3. Synergies: When AI Meets Automation
AI is often termed the “brain,” providing insight or prediction, while automation is the “hands,” executing routine tasks consistently. Combining them can magnify ROI—AI pinpoints anomalies or triggers, and automation carries out necessary follow-up actions.
3.1 Layering Intelligence onto Processes
Imagine AI as a decision-maker in workflows:
AI Identifies a Trigger: A predictive model flags potential equipment failure or an unusually large order.
Automation Executes Follow-Up: The system immediately schedules maintenance, orders replacement parts, or escalates the order approval to a manager—no human input needed unless anomalies persist.
Result: Problems get resolved swiftly, repetitive tasks vanish from human to-do lists, and resources shift to high-value projects like product innovation or client relationships.
3.2 Real-World Example: Predictive Maintenance + Automated Alerts
Predictive Maintenance: AI reviews sensor data (temperature, vibration) on critical machines to predict breakdowns.
Automated Alert System: Once the AI model predicts a fault, an automation workflow sends real-time notifications to technicians, auto-orders spare parts, or schedules downtime at non-peak hours.
SME Benefit: The synergy ensures minimal production disruption, more accurate parts management, and stronger equipment longevity—saving money and improving reliability.
4. Practical Use Cases for SMEs
Many SMEs incorrectly assume robust AI/automation combos are off-limits due to budget or skill constraints. In practice, cloud-based solutions, open-source tools, and pay-as-you-go platforms have lowered entry barriers, making integrated AI-automation setups feasible even for smaller organizations.
4.1 Customer Support
Automation: Chatbots responding to FAQs, auto-routing complex queries to human agents.
AI: Natural Language Processing (NLP) that understands user intent or sentiment, refining the chatbot’s responses over time.
Outcome: Reduced support ticket volume, faster response times, and a more personalized user experience—leading to happier customers and more efficient support teams.
4.2 Marketing & Sales
Automation: Automated lead nurturing emails triggered after specific user actions (like eBook downloads, webinar attendance).
AI: Lead scoring models that rank prospects based on their likelihood to convert, adjusting email sequences or frequency accordingly.
Outcome: Sales teams focus on high-potential leads, raising conversion rates while reducing wasted outreach efforts.
4.3 Inventory & Supply Chain
Automation: Auto-reordering products once stock levels fall below a preset threshold.
AI: Demand forecasting that dynamically updates reorder points based on real-time data—seasonal trends, promotions, or external factors.
Outcome: Fewer stockouts and less wasted capital in overstock, enabling more precise inventory management.
4.4 Quality Control
Automation: Fixed inspection steps for standardized checks—like verifying packaging labels or product weight.
AI: Computer vision analyzing products for defects (color variations, shape anomalies) with advanced pattern recognition.
Outcome: More consistent quality checks, immediate identification of subpar products, lower returns or warranty claims, and brand reputation gains.
5. Implementation Considerations for SMEs
Adopting AI and automation effectively requires planning, structured rollouts, and stakeholder buy-in. Many SMEs jump directly to technology procurement without assessing these foundational elements, leading to projects that never fulfill their promise.
5.1 Identify Clear Goals
Why: Projects with fuzzy objectives rarely yield measurable returns. Are you aiming to cut operational costs by 15% or halve customer wait times? Having specific goals shapes solution design.
How: Engage stakeholders (department heads, IT, finance) to define success metrics. If your main pain point is shipping delays, you might set a target to reduce average fulfillment time by 20%.
5.2 Data Readiness
Why: AI relies on data—clean, relevant, and adequately sized.
How: Conduct data audits. If you’re automating lead qualification, ensure CRM entries are consistent. If you’re deploying chatbots, gather a comprehensive dataset of past customer queries to train NLP models.
5.3 Scalable Infrastructure
Why: If an AI-automation pilot proves successful, your usage may grow.
How: Opt for cloud solutions that accommodate surges in data or activity, preventing heavy upfront hardware costs. Keep integration paths open, so new tools can slot in without massive reengineering.
5.4 Change Management
Why: Teams may fear job loss or be skeptical about new tech.
How: Communicate benefits clearly—highlight how these tools free staff from tedious tasks and offer new learning opportunities. Provide training and address concerns promptly. Early success stories or pilot wins can rally support.
5.5 ROI Tracking
Why: Showcasing ROI cements ongoing investment and expansion.
How: Define metrics early—like tasks automated per month, cost savings, increased sales conversion rates, or error reductions—and capture them systematically. Review monthly or quarterly to refine your approach, pivoting if some aspects underperform.
6. Conclusion: Harnessing the Power of AI + Automation
For many SMEs, time and resource constraints make technology adoption a challenging puzzle. Artificial Intelligence and automation each serve crucial roles, but it’s their synergy that accelerates growth:
Automation rapidly executes predictable workflows, providing speed, consistency, and reliability.
AI adds intelligence—learning and adapting to new patterns, handling complexity, and spotting hidden trends.
When SMEs integrate both in a strategic, staged manner—starting from well-defined pain points to show quick wins—the result is often compounded ROI: improved customer service, tighter operational efficiency, and data-driven agility. Whether it’s a chatbot triaging customer queries or predictive analytics guiding inventory replenishment, weaving AI intelligence into automated tasks yields outcomes greater than the sum of the parts.
At HIGTM, we help SMEs navigate each step, from clarifying objectives to verifying data readiness, ensuring that you invest in solutions aligned with your immediate goals and long-term vision.
By distinguishing AI’s “brain” from automation’s “hands,” you’ll optimize resources, strengthen team collaboration, and confidently move into an era where both technologies serve as the backbone of a modern, future-facing enterprise.
Turn AI into ROI — Win Faster with HIGTM.
Consult with us to discuss how to manage and grow your business operations with AI.
© 2025 HIGTM. All rights reserved.