88. AI & MLOps Trends Transforming Retail
For retail executives, the challenge is twofold: identify which AI trends will deliver the most value, and implement them effectively through robust MLOps practices. This article explores the most impactful trends in AI and MLOps for retail, with a focus on real-time decisioning, multimodal and generative AI, AI governance, and synthetic data. Each section provides data-driven insights and practical examples relevant to retailers, especially those with 50+ employees looking to scale these solutions. By understanding these trends, retail leaders can make informed decisions and position their companies at the forefront of innovation – with HI-GTM as a partner in navigating this journey.
Q1: FOUNDATIONS OF AI IN SME MANAGEMENT - CHAPTER 3 (DAYS 60–90): LAYING OPERATIONAL FOUNDATIONS
Gary Stoyanov PhD
3/29/202521 min read

1. Real-Time AI in Retail
Modern retail operates in real time. Customers expect instant responses – whether it’s accurate stock availability on a website or personalized recommendations as they shop. Real-time AI refers to systems that can ingest data and produce insights or actions immediately, with minimal latency. For retailers, this capability is becoming a game-changer in several areas:
Dynamic Pricing Optimization: AI-driven dynamic pricing allows retailers to adjust product prices on the fly based on live factors like demand, inventory levels, or competitor pricing. This strategy isn’t about surge pricing alone; it’s about micro-adjustments that optimize revenue and move inventory. According to SAP’s industry report, AI-based dynamic pricing can increase revenue by about 1.3% annually on average. For a retailer, that could mean millions in additional profit. Companies like Amazon famously reprice items multiple times a day using AI. Now, with cloud-based solutions (e.g., AWS Pricing Engine or Azure AI), even mid-sized retailers can implement real-time pricing models to stay competitive and responsive.
Hyper-Personalization in the Moment: Real-time recommendation engines analyze a shopper’s current behavior (clicks, views, cart additions) and cross-reference it with historical data to serve up the most relevant content or product instantly. The payoff is significant – recommendations powered by AI can drive a substantial portion of e-commerce sales and increase basket sizes. Retail giant Netflix (in entertainment) set the expectation for personalization, and in retail, Amazon’s “Customers who bought X also bought Y” is classic. Now, newer generative AI models can even personalize marketing messages or on-site search results in real time, creating a one-to-one experience for each customer. A Salesforce study cited in NVIDIA’s retail report found 92% of retailers are increasing investment in generative AI for better customer experiences, focusing on hyper-personalization.
Instant Inventory Intelligence: Keeping shelves stocked with the right products is a perennial challenge. AI-powered demand sensing uses real-time data – point-of-sale transactions, online trends, even local weather – to forecast short-term demand and trigger replenishment or re-allocation of stock. For example, if a sudden social media trend causes a spike in demand for a product, a real-time AI system can alert the retailer to redistribute inventory to affected stores or ramp up online stock. Consumers notice the difference: 67% of shoppers want real-time stock updates, and 31% have been frustrated by items being out of stock. By responding instantly to demand fluctuations, retailers not only prevent lost sales but also reduce overstock and waste. Solutions like Google Cloud’s Recommendations AI and Vertex AI can integrate inventory data to recommend stock transfers or highlight items at risk of selling out.
Fraud Detection and Security: Retail transactions (especially online) face fraud in real time, and AI is stepping up to counter it. Machine learning models monitor transactions as they happen and flag anomalies within milliseconds – far faster than human review. For instance, unusual purchasing patterns or a mismatch in shipping address triggers the AI to either halt the transaction or require additional verification. These systems must operate in real time to be effective. PayPal and other payment processors leverage such AI, and services from AWS (Fraud Detector) or Microsoft Dynamics 365 Fraud Protection are available for retailers to integrate. Stopping fraudulent orders in real time saves retailers money and protects genuine customers from hassles.
Implementing real-time AI requires robust infrastructure. This is where partners like NVIDIA (for high-performance GPUs that make split-second computations possible) and cloud providers (for scalable, low-latency data processing) come in. Importantly, MLOps practices are needed to support real-time systems – models must be continuously monitored and retrained as data drifts over time. In a real-time setting, a model’s failure or slowdown can impact sales immediately, so MLOps capabilities like automated monitoring, alerts, and fallback mechanisms are critical. Retailers are increasingly adopting hybrid deployment approaches (cloud + edge) for real-time AI: critical systems can run at the store level or on edge devices to avoid internet latency, with cloud as backup or for heavy computations.
For retail executives, the message is clear: speeding up the decision cycle through real-time AI can deliver tangible ROI. Whether it’s slightly boosting margins on each sale or preventing a customer from seeing an out-of-stock item, these incremental improvements scale up. The key is focusing on use cases where immediacy matters and ensuring the tech is reliable. With HI-GTM’s expertise, a retailer can identify the highest-impact real-time AI opportunities (like dynamic pricing for fast-moving goods or instant personalized offers at checkout) and build the MLOps scaffolding to deploy them successfully.
2. Multimodal Models and Generative AI in Retail
Today’s AI systems are no longer limited to just one type of data or output. Multimodal models – which can process and generate text, images, audio, and more – are unlocking creative and practical applications in retail. Alongside them, generative AI (AI that creates content) is enabling new ways to engage customers and design products. Major tech companies have been at the forefront of this: OpenAI’s GPT-4 can handle text and images, Google’s research on multimodal AI (like PaLM and Muse models) is enabling rich media understanding, and Hugging Face’s open-source models make multimodal tech accessible to smaller players. Here are the top multimodal and generative trends making waves in retail:
Visual Search and Shopping: Consumers increasingly want to search for products using images – “Show me similar items” via a photo. Multimodal AI makes this possible. An AI model can take an image input (say a customer snaps a picture of a dress they liked) and then search a product catalog for visually similar items, even accounting for style and color. This blends computer vision and recommendation systems. Google Lens and Pinterest Lens popularized visual search for consumers, and now retailers are integrating this capability into their apps and websites. For example, IKEA’s Place app lets users visualize furniture in their room (using AR, which is another form of multimodal experience combining spatial data and product images). Retailers adopting visual search tools have seen higher conversion rates, as customers find what they really want faster. The technology relies on models that understand both images and product metadata – a multimodal understanding that is far more advanced than a text-only search engine.
AI-Generated Product Content: Generative AI is transforming how product descriptions, ads, and even images are created. Traditionally, writing unique descriptions for thousands of products or generating lifestyle photos is labor-intensive. Now AI can assist or automate much of this. OpenAI’s GPT series can draft product descriptions or marketing copy that a human editor can fine-tune – saving time while maintaining brand voice. On the visual side, models like DALL·E 3 or Stable Diffusion (accessible via Hugging Face or Azure’s OpenAI Service) can generate product images or background art. We’re seeing retailers use these tools to create endless variations of marketing visuals (for example, placing a product in different holiday-themed scenes) without hiring photographers for each variation. One high-impact use case is A/B testing ad creatives: generative AI can produce multiple versions of an ad, and the retailer’s marketing team can quickly test which one resonates most, refining the style in a data-driven way. The result is more engaging content that can drive click-through and sales – all produced at a fraction of traditional cost and time.
Multilingual and Multimodal Customer Service: Retail is global, and customer service queries come in various forms – emails, chat messages, voice calls, even photos (“The item I received has this defect, see attached image”). AI that can handle multiple languages and input types provides superior service. For instance, multilingual chatbots powered by generative AI can understand a customer’s question in French or Spanish and respond in kind. NVIDIA’s survey highlights that retailers are adopting branded virtual assistants and multilingual chatbots to enhance service.
Now, consider a customer sending a picture of a damaged item: a multimodal AI could analyze the image to identify the issue and trigger the appropriate response (refund or replacement) without human intervention. Microsoft’s Kosmos-1 and similar emerging models are specifically aiming to combine vision, text, and even audio understanding. The benefit to retailers is faster, more accurate customer support. It can reduce return processing time, improve customer satisfaction, and free up human agents to handle truly complex cases.
Design and Merchandising Innovation: Generative AI is also an ideation partner. It can create new product designs (patterns for fashion, flavor combinations for foods, layouts for store displays) that human designers might not have imagined, serving as a creative brainstorming tool. For example, an apparel retailer can use generative models to produce dozens of new pattern ideas for a season’s collection, then have designers curate and refine the best ones. In merchandising, generative AI can simulate store layouts or shelf arrangements in VR based on different themes or traffic flows, letting the team “test” concepts digitally. This is analogous to running many experiments quickly. Some big retailers are exploring AI-generated virtual models to showcase clothing without needing a photo shoot – the AI can put the clothing on synthetic but realistic models of different body types, poses, and even generate short catwalk videos. This not only saves cost but also ensures inclusivity (AI can easily generate models of various ethnicities and sizes to reflect a diverse customer base).
The common thread in these applications is the blending of multiple data types. To implement them, a robust data foundation and integration is required. Retailers need to combine image databases (product photos, in-store footage), text databases (product specs, customer reviews), and audio (call center logs, if voice is considered) to train or fine-tune these models. Hugging Face’s Transformers library and model hub have made it easier to get started by providing pre-trained multimodal models that can be adapted to specific retail tasks.
However, deploying such models in production demands a strong MLOps framework. These models are often large (think hundreds of millions of parameters like GPT-4 or Vision Transformers), so optimizing them for inference (using techniques from NVIDIA like TensorRT or using AWS Inferentia chips, etc.) is crucial for performance and cost. Monitoring is equally important – for instance, if a generative model starts producing off-brand or incorrect content, there needs to be a review and update process (this ties into AI governance, discussed later).
In summary, multimodal and generative AI unlocks exciting possibilities for customer engagement and operational creativity in retail. Early adopters are creating more intuitive shopping experiences – customers can search how they think (with words or images), and they get richer content that drives buying confidence. The key is to start with a clear use case: e.g., implement an image-based search for your catalog, or use GPT to automate product copy for a specific category. HI-GTM advises focusing on one high-impact application at a time, proving the ROI, and then scaling up – all while ensuring your MLOps pipeline can handle the complexity of these advanced models.
3. AI Governance and Responsible AI in Retail
As retailers integrate AI deeper into their operations, AI governance becomes a critical theme. AI systems can affect pricing, hiring, customer interaction, and more – so ensuring these systems operate fairly, transparently, and in line with company values is paramount. Retailers also deal with sensitive customer data (purchase histories, personal preferences, payment information), which raises the stakes for privacy and compliance. High-profile tech companies have learned the hard lessons of AI risks, and they are now providing tools and frameworks to help businesses govern AI use. For retail executives, establishing a robust AI governance practice isn’t just about avoiding negative outcomes – it’s about building trust with customers and stakeholders, which ultimately protects the brand and enables sustainable AI innovation.
Here are key aspects of AI governance in the retail context:
Data Privacy and Compliance: Retailers must navigate regulations like GDPR, CCPA, and PCI DSS (for payment data) while using AI. A top concern for retail AI programs is data privacy – cited by 39% of retailers as their #1 concern with generative AI adoption. AI governance starts with how data is collected, stored, and used. For example, if you’re using customer transaction data to train a recommendation algorithm, governance policies should dictate encryption, access controls, and anonymization where possible. Many retailers are turning to privacy-preserving techniques like data masking or federated learning (where raw data stays on-device and only aggregated models are shared). Synthetic data (as discussed in the next section) is another tool, as it allows training AI on artificial data that mirrors real patterns without exposing actual customer details. Compliance officers and AI teams need to work hand-in-hand – governance frameworks like the EU’s upcoming AI Act will likely classify some retail AI use cases (e.g. credit scoring for store cards or fraud detection) as high-risk, demanding extra oversight.
Bias and Fairness in Algorithms: An AI model is only as good as the data and assumptions behind it. In retail, this might manifest in a product recommendation system inadvertently underserving a segment of customers (perhaps not showing premium products to certain demographics due to learned biases in data), or an AI hiring tool for retail staff disfavoring certain groups (a lesson from Amazon, which had to scrap an AI recruiting tool that showed gender bias). Governance means proactively testing models for bias and fairness. Tools like Amazon SageMaker Clarify provide bias detection metrics out-of-the-box, and companies like IBM have open-source toolkits (AI Fairness 360) for this purpose. A governance framework would establish that before any AI model is deployed, it undergoes fairness testing across key attributes (gender, age, location, etc., as appropriate). If biases are found, the model must be adjusted or retrained with more balanced data. For retail, fairness can also mean algorithmic transparency – for instance, if an AI sets prices, can the company explain to regulators or customers why a price was higher or lower for a certain region? Having that explainability is crucial to avoid reputational harm or legal issues.
Brand Reputation and AI Behavior: Generative AI, in particular, poses a risk that the AI might produce content that is off-brand or offensive. Imagine a chatbot responding to a customer with a joke that goes wrong, or a generative ad text that inadvertently uses language inconsistent with the brand’s voice. Governance here involves setting “guardrails” for AI behavior. NVIDIA’s open-source NeMo Guardrails toolkit is one example that helps developers define rules for conversational AI (e.g., topics to avoid, style guidelines).
Microsoft and OpenAI also enforce content moderation filters on their AI APIs to prevent toxic or inappropriate outputs. Retailers should define their own guardrails: a fashion retailer’s AI stylist bot, for example, should be positive and never body-shame or make sensitive comments, even inadvertently. Testing AI systems extensively for edge cases is part of governance. Some retailers assemble diverse internal teams to “red-team” the AI (stress test with tricky inputs) before wide release.
Accountability and Human Oversight: Who is responsible when an AI makes a mistake? AI governance establishes clear lines: there should be a human in the loop or on call for critical AI decisions. For example, if an automated system flags a huge bulk order as fraud and plans to cancel it, governance might dictate that a human review orders above a certain dollar threshold to avoid false positives that anger big customers. Many retailers start AI projects with a augmented intelligence mindset – AI provides recommendations, but humans approve. As confidence grows, some decisions automate, but even then there should be monitoring. Model monitoring and drift detection (part of MLOps) feed into governance by alerting when a model’s performance degrades or its predictions start to skew. If a model that was fair at launch becomes biased after 6 months due to shifting data, governance policies should trigger retraining or pulling the model from production until fixed. Retailers also benefit from forming an AI Ethics Committee or task force, including stakeholders from IT, legal, customer experience, and even customer representatives if possible. This group reviews new AI use cases and policies, ensuring alignment with company values and societal norms.
Vendor and Partner Governance: Using third-party AI services (from cloud providers or startups) is common – e.g., using an external API for image recognition in store. Governance means holding these vendors to your standards. Retailers should vet how an AI service handles their data (Is it used to train others’ models? How is it stored?), what bias mitigations the vendor has, and what audit rights the retailer has. Contracts should include clauses about data ownership and privacy. The good news is major vendors understand this need: Microsoft, Google, and AWS all have responsible AI programs and often share documentation on how their models are trained and what data was used (for instance, OpenAI publishes model cards that detail known limitations of models like GPT-4). As a retailer, insist on transparency from partners – it’s part of your governance due diligence.
In practical terms, implementing AI governance in retail can start with a clear responsible AI framework. This is a set of principles (like fairness, privacy, transparency, accountability) and procedures (like bias testing, regular audits, incident response plans). For example, a principle might be “Our AI will never discriminate based on protected characteristics,” and the procedure is “Perform quarterly reviews of recommendation outcomes by demographic segment.” Major consulting firms and tech companies often provide templates for such frameworks – but they must be tailored to each organization. HI-GTM, for instance, works with clients to embed governance from day one of an AI project, rather than treating it as an afterthought. This includes training the team on ethical AI and establishing checkpoints in the project plan for governance review.
It’s worth noting that good governance isn’t a blocker to innovation – it’s an enabler. Companies with strong AI governance find that they actually innovate faster because they clear the path of potential minefields early. They build customer trust (customers are more willing to share data and try new AI-driven services if they trust the brand’s use of AI) and they avoid costly backtracking. In retail, trust is everything. AI governance ensures that while we leverage cutting-edge tech like real-time personalization and generative AI, we do so in a way that strengthens the brand promise of treating customers fairly and respectfully.
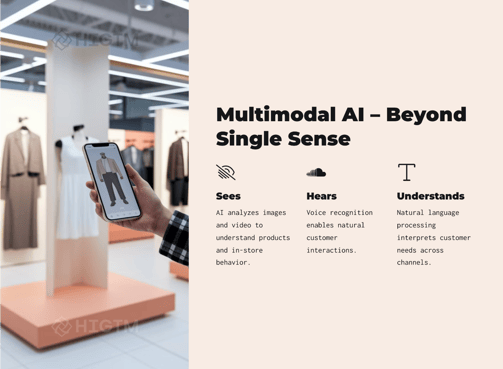
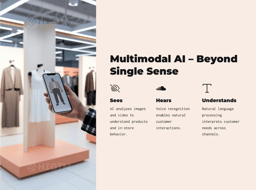
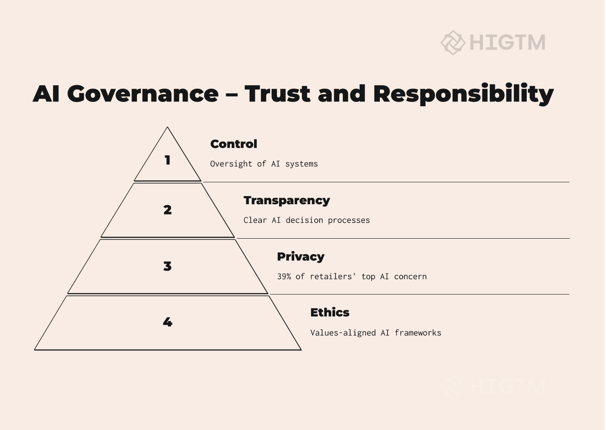
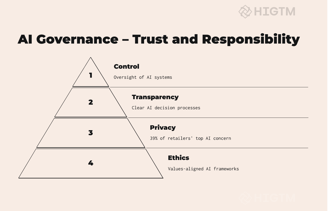
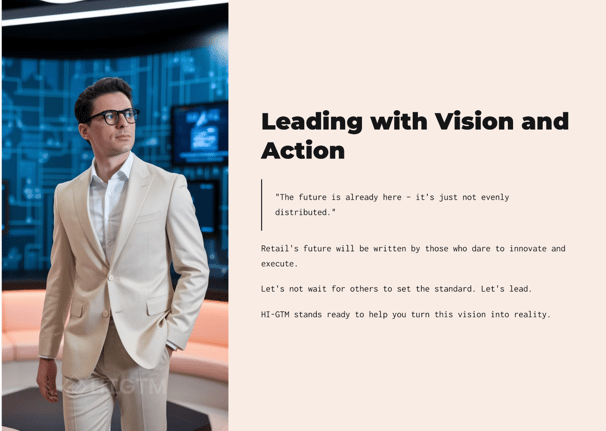
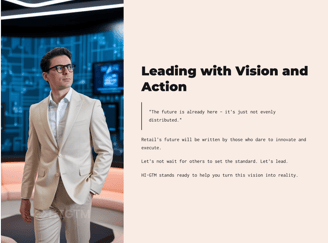
4. Synthetic Data: Amplifying Retail AI with Artificial Data
Data fuels AI, but retailers often struggle with obtaining enough quality data for every scenario, all while respecting privacy and security. Synthetic data has emerged as a high-impact trend to address this challenge. Synthetic data is artificially generated data that mimics the patterns of real data without being tied to actual individuals or events. Thanks to generative models and simulations, synthetic data can take many forms – customer profiles, transaction records, product images, store foot traffic patterns, and more. In retail, synthetic data offers a way to innovate faster, test more thoroughly, and protect customer information. It’s a perfect example of how generative AI (one of our key trend themes) is not just creating content, but also creating data for AI.
Here are some of the most valuable uses of synthetic data in retail:
Augmenting Training Data for AI Models: Often, training an AI model (say, for demand forecasting or image recognition) requires more data than a retailer has on hand. Synthetic data can fill these gaps. For instance, to train a computer vision model that identifies products on store shelves, you might need tens of thousands of images of shelves under various conditions (different lighting, angles, product arrangements). Rather than manually collecting that, retailers can use a simulator – like NVIDIA’s Omniverse Replicator or Unity’s synthetic data tools – to generate photorealistic images of store shelves with randomized variations. This synthetic image dataset can be as large as needed and comes with automatic labels (since in simulation you know which product is where). By training vision models on a mix of real and synthetic images, companies have achieved higher accuracy in tasks like shelf scanning and checkout-free store technology. Synthetic data is also used for scenario generation in forecasting: if you have only a few years of sales data, you could use generative models to create additional plausible sales sequences under various hypothetical events (e.g., what if a new competitor opens next door?) to train more robust forecasting models.
Preserving Privacy with Synthetic Customer Data: Retailers hold sensitive customer data – purchase histories, loyalty program details, demographics. Sharing or using this data in development can raise privacy issues. Synthetic data provides a privacy-friendly alternative. Tools (offered by startups like Mostly AI or older ones like IBM Data Fabric) can learn the statistical patterns of the real customer data and then generate fake customer records that look and behave like the real ones without referring to actual people. For example, a synthetic dataset might retain that 5% of customers in a region buy luxury brands exclusively and typically in Q4, without copying any one person’s exact record. Data scientists can use this synthetic customer data to build recommendation algorithms or customer segmentation models without accessing the raw customer PII (Personally Identifiable Information). This greatly reduces compliance risk. If the synthetic data generation is done well, models trained on it perform similarly to those trained on real data. Some retailers even share synthetic transaction datasets with partners for joint analysis or benchmarking – something they could never do with real data due to privacy restrictions.
Testing and QA for Systems: Beyond model training, synthetic data is a boon for testing IT systems. Consider a retailer implementing a new point-of-sale system or e-commerce platform. Before going live, they need to test with lots of transactions, including edge cases like returns, coupon stackings, or unusual shopping cart combinations. Generating synthetic transaction logs that cover years’ worth of activity can help QA teams ensure the system handles everything correctly. Similarly, for load testing a website, synthetic user traffic data can simulate peak shopping days. Synthetic datasets can also help in scenario planning: want to know how your supply chain system handles 10x online orders (like a Black Friday times ten)? Generate the orders and see how the algorithms and infrastructure cope. This kind of stress test with artificial data prepares retailers for real-world extremes.
New Product Development and Merchandising Experiments: When launching a new product or entering a new market, historical data is limited. Synthetic data can model how customers might react. For example, if a retailer plans to introduce a completely new category, they can generate synthetic customer profiles and purchase behaviors based on analogous products or market research, then use those as input to their inventory and marketing models. It’s a bit like a Monte Carlo simulation – you create many hypothetical “customers” and see what demand might look like under each scenario. This doesn’t replace real pilot programs, but it helps narrow down strategies. In merchandising, synthetic data can assist in space planning: e.g., simulate virtual shoppers moving through a store (using agent-based modeling) to see where bottlenecks might occur or which aisles get attention. These “synthetic shoppers” follow probabilistic rules that mirror real human behavior. Running thousands of virtual shopping trips can yield insights on optimal store layouts or where to place promotional displays.
The rise of synthetic data in retail is closely tied to advances in generative models (GANs, variational autoencoders, transformer-based generators) and simulation environments. NVIDIA, for instance, has invested in simulation for robotics and retail, recognizing that synthetic data can accelerate AI development when real data is expensive or sensitive. Their Retail AI workflows often include synthetic data generation components.
However, synthetic data is not a silver bullet. Quality matters: poorly generated data can mislead models. For example, if synthetic customer data doesn’t correctly capture seasonality (holidays, weather impacts), a model trained on it might perform badly in real life. Therefore, an MLOps approach is important when using synthetic data. It includes validating synthetic data against real hold-out data – do models trained on synthetic generalize to real? If not, refine the generation process. Another aspect is governance: synthetic data reduces privacy risk but doesn’t eliminate the need for caution. There’s a chance (if not properly done) that synthetic data could accidentally memorize and leak real records, or introduce bias (if the generation learns biases in the source). Techniques like differential privacy and careful evaluation are part of governance for synthetic data usage.
From a strategic standpoint, retail executives should view synthetic data as a force multiplier. It can shorten development cycles (data collection no longer a bottleneck) and open up new possibilities to use AI where it was previously not feasible due to data scarcity. A survey noted that 46% of retailers are exploring synthetic data generation as a use case for AI – that’s nearly half the industry seeing the value. Early adopters in e-commerce and finance have shown that models trained even 100% on synthetic data can perform impressively on real-world tasks, provided the synthesis is carefully done.
To get started, a retailer might pick a contained project: for example, generate synthetic augmentation data for a single store’s foot traffic and see if it improves a staffing optimization model. Or use synthetic profiles to test a new loyalty program algorithm. By starting small, the team can develop know-how in this emerging area. HI-GTM often helps clients pilot synthetic data initiatives by connecting them with the right generation tools and establishing validation frameworks to ensure the synthetic data is actually useful.
In conclusion, synthetic data is becoming an essential part of the AI toolkit in retail. It speaks to an overarching theme: retailers that leverage AI and MLOps smartly don’t passively wait for perfect data – they create it or simulate it. This proactive approach, combined with strong governance, allows for continuous innovation without the traditional constraints. For retailers, that means staying one step ahead in delighting customers and optimizing operations.
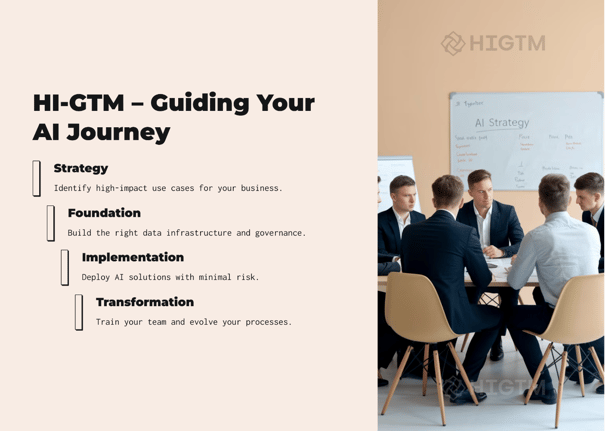
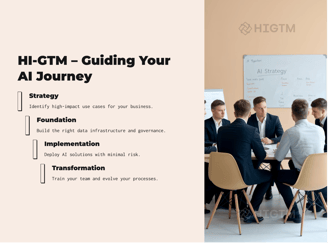
5. Conclusion: Turning AI Trends into Retail Transformation
The trends we’ve explored – real-time AI, multimodal and generative models, AI governance, and synthetic data – are not just buzzwords, but powerful forces already reshaping the retail industry. They each address core retail challenges: staying agile with market changes, engaging customers on their terms, maintaining trust and compliance, and overcoming data limitations. It’s evident that retailers who effectively harness these trends are gaining a competitive edge, from increased revenue to stronger customer loyalty. For example, companies using real-time analytics and dynamic pricing enjoy more reliable margins and fewer stockouts, while those embracing hyper-personalization see higher customer engagement (78% of marketers reported increased engagement through AI personalization). The industry data speaks loud and clear – the question is no longer if AI should be adopted in retail, but how to do it smartly and at scale.
Implementing these advances is not trivial. It requires a blend of strategic planning and technical execution – exactly where a strong MLOps foundation pays off. To summarize actionable steps for retail executives:
Identify High-Impact Use Cases: Rather than attempting a bit of everything, focus on 1-2 areas where AI can drive immediate value. It could be improving online conversion through better recommendations, or reducing labor costs via automation in inventory management. Use the trends as inspiration – maybe synthetic data can finally enable an AI for that niche problem you couldn’t solve due to data paucity, or maybe a multimodal chatbot can reduce customer service wait times. Quantify the potential impact (e.g., “a 0.5% lift in conversion is $X in sales”) to prioritize projects.
Secure Leadership Buy-In and Build Talent: Change needs champions. Ensure the C-suite and key stakeholders understand these AI trends not as tech experiments, but as strategic initiatives aligned with business goals. Education sessions or quick prototypes can help demonstrate value. Also, invest in your team – upskill existing analysts and IT staff in data science and MLOps, and consider hiring experienced ML engineers or partnering with firms like HI-GTM for expert guidance. A center of excellence for AI/ML in your organization can coordinate efforts and disseminate best practices.
Lay the Data and MLOps Foundation: Before diving headlong into AI, assess your data readiness. Clean, unified, and accessible data pipelines are essential. Modern cloud data warehouses (Snowflake, BigQuery, etc.) and lakes can store the multi-modal data you’ll need. Simultaneously, set up MLOps tools for version control (Git for code, DVC for data, etc.), CI/CD for model deployment, and monitoring (tools like MLflow, SageMaker Model Monitor, or Azure ML monitoring). It’s like setting up the assembly line before building the new car model – it accelerates and de-risks the actual AI development. Remember that 87% of models never reach production due to operational challenges. By emphasizing MLOps, you ensure your AI investments truly make it into day-to-day use.
Embed AI Governance from Day One: As discussed, don’t wait for an incident to then implement governance. Define your AI ethics principles now. Put checks in project charters that any model will be evaluated for bias and privacy impacts. Bring legal and compliance teams into the loop early when planning a new data-driven initiative. This not only prevents issues but can also shape a solution that is robust and sustainable. For instance, deciding upfront to use synthetic data for a particular model might avoid a lot of red tape and still deliver results. Transparency to customers can also be part of governance – some retailers openly communicate how AI is used (e.g., “This price was determined in part by an algorithm that factors in real-time demand”) which can demystify and build trust, provided the practices are fair.
Leverage the Ecosystem of Tools and Partners: The heavy lifting of AI technology is being done by the likes of OpenAI, Google, AWS, Microsoft, NVIDIA, and the open-source community. Retailers should not try to reinvent the wheel. Instead, capitalize on these platforms: use Azure OpenAI Service to access GPT-4 with enterprise security, use Google’s translation AI to instantly offer multi-language support, or NVIDIA’s optimized libraries to deploy vision AI faster. Hugging Face offers countless pre-trained models – before collecting data to train your own model, check if someone has shared a similar model you can fine-tune. Engaging with partners (technology providers or consultants) can accelerate adoption by bringing in outside expertise. HI-GTM, for instance, can help integrate these various services into a coherent solution tailored to a retailer’s needs, ensuring you get the best of all worlds.
Start Small, Then Scale Fast: A recommended approach is running a pilot project for each trend to learn what works in your context. Maybe start real-time AI with one product category’s dynamic pricing, or test a multimodal search on a subset of your catalog. Use those pilots to gather results and feedback. Once success is demonstrated (or lessons learned from failure), have a plan to scale across the chain or the platform. The benefit of modern AI platforms is scalability – if it works in one store or one region, it can often be rolled out quickly via cloud infrastructure. MLOps again helps here, as automated deployment means you can push a successful model to hundreds of stores or a million users with minimal friction. Keep an eye on change management – train your staff on new AI-driven processes, and possibly educate customers too (e.g., a brief intro on the website about a new AI feature).
Measure and Iterate: Define clear KPIs for each AI initiative. It could be revenue lift, cost reduction, NPS (Net Promoter Score) improvement, or efficiency gain. Track these religiously. AI projects should not be science experiments; they need business metrics attached. If a model isn’t delivering the expected lift, investigate and iterate – maybe the model needs retraining, maybe the feature needs rethinking, or maybe user adoption is an issue (are customers using that visual search feature?). Retailers that succeed with AI treat it as a continuous improvement process, not a one-off implementation. They use A/B testing to compare AI-driven decisions against traditional methods, ensuring the AI is truly adding value. The beauty of AI systems is that they can often improve over time (learning from new data), but only if retrained and tuned – which again underscores MLOps and ongoing investment.
In closing, the retail industry is at an inflection point. AI and robust MLOps practices are shifting AI from a buzzword to a baseline capability. Just as e-commerce went from novel to necessary, AI-driven operations will become standard in retail. Executives have a mandate to not only understand these technologies but to weave them into their strategic plans. The trends highlighted are those with the highest impact observed in the field today, and they also lay the groundwork for future innovations (for example, real-time AI and multimodal capabilities will be prerequisites for whatever comes next, be it the metaverse shopping or advanced robotics in stores).
HI-GTM (High Impact Go-To-Market) is positioned to be the go-to partner for retailers on this journey. We combine deep knowledge of these AI trends with hands-on experience in retail implementation. Whether it’s building a roadmap for AI adoption, executing a pilot with agility, or establishing the MLOps and governance structures necessary for scale, we ensure that our clients turn hype into real results. Retail executives exploring AI adoption should feel encouraged: the tools have matured, and the case studies of success are growing. With the right guidance and focus, any retailer can start reaping the benefits of AI – not in some distant future, but today.
Next Steps: If you’re ready to lead your retail organization into this new age of intelligent retail, consider scheduling a strategy session with HI-GTM. We’ll assess your current state, identify the quick wins from the trends that fit your business, and outline a clear plan to implement AI and MLOps for sustainable success. The companies that act decisively now will set themselves apart as industry frontrunners in the years ahead. The AI revolution in retail is here – it’s time to embrace it with both enthusiasm and prudence, turning cutting-edge tech into competitive advantage.
Turn AI into ROI — Win Faster with HIGTM.
Consult with us to discuss how to manage and grow your business operations with AI.
© 2025 HIGTM. All rights reserved.