87. Pivoting AI Projects: How Enterprises Re-Align AI Initiatives for Maximum ROI
Business leaders are finding that the key to AI success isn’t just choosing the right project – it’s knowing when to pivot that project. Major AI vendors like OpenAI, Microsoft (Azure), Google Cloud, AWS, and IBM Watson have all inspired transformative use-cases, but enterprises often discover mid-journey that a shift in direction is needed to truly capitalize on AI. This article explores the most impactful AI pivot scenarios in enterprise settings, from shifting a project’s focus (e.g. from predictive analytics to full automation) to adapting due to new regulations or scaling challenges. We’ll dive into data-driven strategies for recognizing when an AI pivot is necessary – using performance metrics, cost-benefit analysis, user adoption trends, and risk assessments.
Q1: FOUNDATIONS OF AI IN SME MANAGEMENT - CHAPTER 3 (DAYS 60–90): LAYING OPERATIONAL FOUNDATIONS
Gary Stoyanov PhD
3/28/202520 min read

1. What is an AI Project Pivot and Why Do It?
In business, a “pivot” means a structured course correction – and for AI projects, a pivot involves making a significant change in the project’s direction, scope, or implementation approach. This could mean repurposing an AI solution for a different use-case, switching underlying technologies or vendors, or altering how an AI tool is deployed and used within processes. Unlike a minor tweak or feature update, a pivot is more fundamental: it’s a response to the realization that the current trajectory won’t achieve the desired results or could achieve far greater results if adjusted.
Why pivot an AI project?
Because the reality of deploying AI in enterprises is full of surprises. Maybe the initial model isn’t hitting accuracy targets or business KPIs, maybe users aren’t adopting that new AI-driven system, or perhaps a disruptive AI technology (like a new powerful open-source model) emerged, offering a better path. External forces can compel pivots too – regulatory changes or shifting market conditions might suddenly make your AI approach outdated or non-compliant. As an executive, recognizing the need to pivot and acting on it can save a project from failure or elevate it to new heights. In fact, by some estimates more than 80% of AI projects fail to reach their intended goals, often because organizations stick with flawed assumptions too long. The ability to pivot effectively is what separates AI initiatives that deliver real ROI from those that end up as expensive experiments.
2. Data-Driven Signs That It’s Time to Pivot
How can you tell, in practical terms, that an AI project needs a pivot? Successful course correction starts with data-driven decision-making. Here are key indicators and metrics executives should monitor:
Performance Plateau or Decline: If your AI model’s performance metrics (prediction accuracy, error rate, etc.) have stagnated below the target threshold and efforts to improve yield diminishing returns, it’s a strong signal. For example, imagine a predictive maintenance AI stuck at 60% precision when 90% is needed – despite more training, it won’t budge. That plateau suggests a pivot to a new model architecture or data source might be necessary. Always benchmark metrics against the business impact: if the AI isn’t moving the needle on critical KPIs (like conversion rate or cost savings), staying the course may not be justified.
Poor Cost-Benefit Balance: Keep an eye on the ROI equation. During pilots, it’s common to invest in AI with uncertain returns, but as data comes in, you must calculate whether benefits outweigh costs. If an AI-driven process automation saved $200K in labor but cost $500K in development and infrastructure, the cost-benefit analysis might tell you to pivot to a different approach or scale down scope. In fact, a recent survey of CIOs found that cost concerns were one of the top reasons AI pilots fail to scale (cited by 68% of respondents). Executives should set clear ROI targets or payback periods. When an AI project consistently fails to meet those, it’s time to explore alternatives – maybe a simpler solution or a different vendor’s platform that is more cost-efficient.
Low User Adoption and Engagement: One underrated metric for AI success is human adoption. You might deploy an AI tool for your sales team, but if most reps find ways to work around it or revert to old tools, the utility of your AI solution is in question. Such user behavior is a loud alarm: either the AI isn’t user-friendly, it’s not trusted, or it’s not truly solving the right problem. For instance, Hugging Face originally launched as a fun AI chatbot app for consumers but noticed that improvements in the AI’s conversational ability didn’t increase user retention. Their user engagement hit a ceiling – a clear sign something was off in the value proposition. The founders smartly pivoted to a completely different model (an open-source AI platform for developers), which turned Hugging Face into a $4.5B success story. In an enterprise setting, if your internal users or customers are not embracing an AI-driven product, use surveys, usage analytics, and direct feedback to understand why. Those insights will point to whether a pivot (in functionality, UX, or target use-case) is needed.
Emerging Risks – Ethical, Regulatory, or Operational: AI projects operate in dynamic environments. A change in privacy laws, a new industry regulation, or discovery of a bias issue in your model can all necessitate a pivot. For example, a bank developing a machine learning model for credit scoring might face new fair-lending regulations requiring explainability. If the current AI is a complex black-box, the bank may need to pivot to a more interpretable AI approach or add robust explanation modules. Not doing so could expose the company to compliance violations or reputational damage. Likewise, internal risk assessments might reveal concerns: perhaps an AI system automating decisions is making errors with serious consequences (e.g. an AI ops tool misrouting critical alerts). If the risk profile becomes unacceptable, it’s time to pause and pivot to either add safety layers or change the project scope entirely. In one famous case, Amazon developed an AI recruiting tool that unfortunately learned to discriminate against female candidates, reflecting historical biases. Upon realizing this, Amazon scrapped the project – essentially a pivot to stop pursuing that AI approach and find a more equitable solution. The lesson: when AI risks emerge, a swift change of direction can save you from bigger troubles down the road.
Opportunity Pull – New Tech or Market Shift: Sometimes it’s not about something going wrong, but about a new, better opportunity arising. The AI field moves fast; a breakthrough (like a new model that is far more powerful) can justify pivoting to leverage it. We saw this when OpenAI’s GPT-3/4 and ChatGPT arrived – many companies pivoted their AI roadmaps to incorporate generative AI capabilities that barely existed a year prior. Similarly, shifts in market demand can prompt an AI pivot: perhaps mid-project you discover a different application of your AI would reach a larger customer base or a more urgent business problem. In these cases, data from market research or competitive analysis – not internal performance metrics – is the trigger. A strategic pivot can help you seize the initiative and not be left behind. The key is ensuring this new direction still aligns with your core business objectives and isn’t just chasing shiny tech for its own sake.
3. Four High-Impact AI Pivot Scenarios for Enterprises
Pivoting is not a one-size-fits-all move; it comes in various flavors. Here we outline four common and impactful AI pivot scenarios that enterprises encounter, each with its own rationale and benefits:
1. From Predictive Analytics to Automation: This is a pivot from insight to action. Enterprises often start with AI for predictive analytics – e.g., forecasting sales, predicting equipment failures, or analyzing customer churn probability. These predictions are valuable, but organizations may struggle with the “so what.” Without integrating those predictions into automated workflows or decision systems, the impact can be limited. For instance, a supply chain team might get a prediction of a spike in demand for a product but fail to adjust production in time, thereby missing sales. Many companies have pivoted such projects into AI-driven automation, essentially upgrading from predictive to prescriptive or autonomous systems. One example: a global retailer initially used AI to predict out-of-stock items in stores, providing reports to store managers. They found managers weren’t acting on the data consistently. The pivot was to let the AI directly initiate restock orders and price adjustments (with managerial override available). The result was faster response and a tangible drop in stockout incidents. In sum, if your predictive AI isn’t translating to outcomes, consider pivoting to an automation approach where AI closes the loop by triggering or even executing decisions. This often delivers a more direct ROI and justifies the AI investment through clear action.
2. From Customer-Facing AI to Backend AI: Chatbots, virtual assistants, recommendation engines – many AI projects put the technology directly in front of customers. While there are great successes in this area, some customer-facing AI implementations falter. Maybe the chatbot isn’t as smart as customers expect, leading to frustration and brand damage, or an AI recommendation system is not boosting sales as hoped. Rather than abandon AI entirely, companies pivot these capabilities to backend or internal uses. An example can be seen in how some banks handled AI chatbots: a few years ago, banks launched customer-facing AI assistants for online banking help. Some found the uptake and satisfaction were low – customers still preferred human agents for complex queries. Banks like one in our earlier slide example then repurposed the chatbot’s NLP engine for internal support, assisting call center reps with suggested solutions and automating simple tasks like password resets behind the scenes. The customer never chats with AI, but they experience better service because AI is working in the background. Another scenario is in e-commerce: if an AI personalization algorithm isn’t lifting sales on the website, the retailer might pivot it to help merchandisers internally (e.g., an AI tool that analyzes trends and assists human buyers with deciding what products to stock). The core technology often remains the same; it’s the interface and user (customer vs employee) that change. This pivot can salvage value from an AI that wasn’t ready for prime time by putting it to work where its limitations are less exposed and its strengths (speed, data processing) can augment human work.
3. Adapting AI to Regulatory & Ethical Changes: Perhaps no pivot is more mandatory than the one forced by regulators or ethical considerations. AI operates in society – handling personal data, making decisions that affect people’s lives – so the rules around it are tightening. Enterprises have faced scenarios where a perfectly functioning AI system had to be significantly altered or replaced to meet new compliance standards or ethical norms. A cross-industry example: the EU’s GDPR and forthcoming AI Act are game-changers. Companies doing AI-driven consumer analytics pivoted away from using certain personal data once GDPR came into effect, some moving to anonymization techniques or synthetic data to keep their AI models useful without violating privacy. In finance, after the 2008 crisis, regulators demanded more transparency in credit models. Banks that had adopted opaque AI models for credit risk had to pivot to Explainable AI (XAI) solutions for compliance – for instance, swapping out a complex ensemble model with a more interpretable one, or adding an explanation layer that regulators could review.
These pivots are often non-negotiable: if you don’t adapt, you might have to shut down the AI system entirely. Ethical pivots, while not always legally mandated, are similarly crucial. For example, if an HR hiring AI shows bias, a company might pivot it to a tool that assists humans rather than making decisions, or retrain it with bias mitigation, or as Amazon did, cancel it. The benefit of such pivots is sustaining the license to operate – maintaining trust with customers, avoiding lawsuits or fines, and upholding your brand’s reputation. Over time, being proactive here turns regulatory constraints into a competitive advantage – you gain expertise in compliant, ethical AI that others will also need to figure out.
4. Scaling from Pilot to Full Deployment (“from Lab to Factory”): This pivot is about moving from proof-of-concept to production – a step so challenging it has its own term: “pilot purgatory.” Many organizations have dozens of AI prototypes that never scale. A pivot in this context means changing your approach to implementation so that the AI project can roll out widely. It may involve choosing a different technology stack that’s more enterprise-ready, re-architecting the solution for cloud scalability, or even changing the process and team structure around the AI (for example, establishing a centralized AI engineering team to productize a model built by a research team). A McKinsey study noted that about one-third of companies were only piloting AI without integrating it into the business.
Breaking out of that pattern might mean pivoting the project’s goals: instead of a narrow focus in the pilot (say, optimizing one step of a manufacturing process), re-scope the project to solve an end-to-end problem which justifies integration. In manufacturing, one firm piloted an AI vision system to detect product defects on a single line. It worked, but scaling to all lines meant huge data and infrastructure challenges, and line managers were hesitant. The pivot was two-fold: technically, they moved to a more robust cloud-based platform for scaling the vision AI; organizationally, they paired the AI with a QA task force in each plant to champion the new process.
They also phased the rollout and iteratively improved the model per new line. This shift in strategy – treating deployment as a core part of the project, not an afterthought – enabled enterprise-wide adoption over a year, where initially the project was shelved after the pilot. The message is clear: a brilliant pilot can still fail if not deployed, so sometimes you must pivot your project management and strategy to go from an AI demo to an AI product used every day.
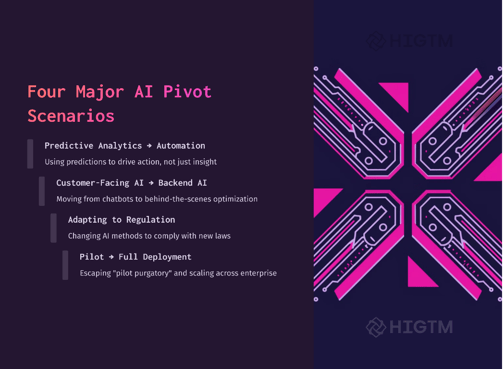
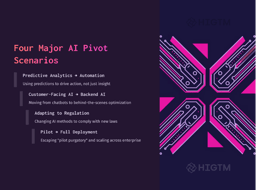
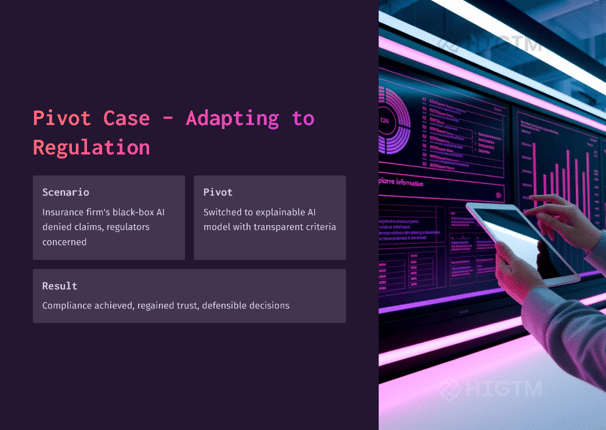
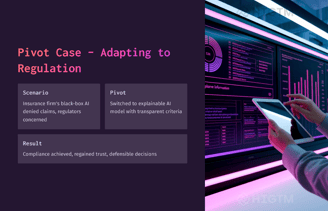
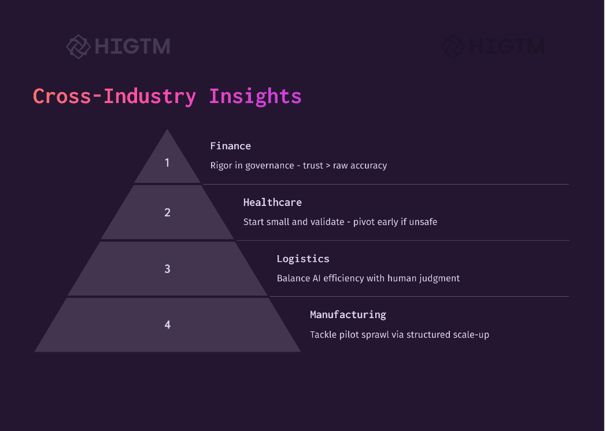
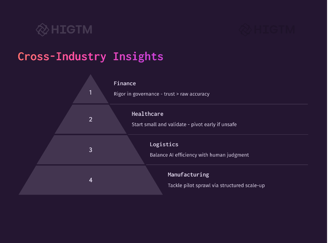
4. Real-World Examples: Pivot Successes and Failures
It’s insightful to examine real cases where AI pivots were made – some that rescued projects and others where a pivot (or lack thereof) taught painful lessons:
Success – Monday.com’s GenAI Rethink: Monday.com, a work management platform, dove into generative AI like many companies after ChatGPT’s rise. Their first implementation – a general AI assistant integrated into the app – didn’t get the user ROI they hoped. Rather than doubling down blindly, they listened to users and pivoted their approach. The result was “AI blocks,” modular AI features embedded directly in workflows (think of an AI that fills in project data in a table column, rather than a clunky chat window). This backend integration pivot led to higher adoption because it fit users’ contexts. Monday.com then extended this to a “digital workforce” concept, showing how a timely pivot can set the stage for innovative product evolution. The lesson: if your first AI use-case isn’t hitting, dig into what users really want. Pivoting to an AI solution that actually solves day-to-day problems can turn an underwhelming feature into a flagship offering.
Success – Standard AI’s Retail Refocus: Standard AI (mentioned in a Big Think interview) provides autonomous checkout technology. They realized that while their cashierless checkout AI worked, it wasn’t scaling to mass retail adoption – possibly due to high installation costs and retailer change resistance. Instead of giving up, Standard AI identified a bigger unmet need: in-store analytics. They pivoted to leverage their computer vision tech to deliver rich data on shopper behavior and store operations, akin to e-commerce analytics for physical stores. This pivot expanded their market and value proposition, turning a narrow solution into a broader retail AI platform. It’s a classic case of repurposing the technology (and the data it collects) to solve a pain point the market was willing to pay for. Retailers who were lukewarm on pure autonomous checkout were very interested in insights on shelf inventory, foot traffic, and loss prevention – all achievable with Standard AI’s tech after the pivot. The takeaway: sometimes your AI is generating a by-product (data, insights) that is more valuable than its primary output. Recognize that and pivot to sell the gold you’re inadvertently mining.
Failure – IBM Watson for Oncology: IBM’s Watson was a media superstar after winning Jeopardy!, and IBM pivoted the technology to tackle healthcare (oncology). It seemed a brilliant move – AI helping doctors cure cancer – but this pivot failed spectacularly in execution. Watson for Oncology struggled with accuracy and relevance in treatment recommendations, leading to unsafe or incorrect suggestions in some cases. Hospitals pulled back, and by 2021 IBM effectively sold off and shut down major parts of Watson Health. What went wrong? In hindsight, IBM likely needed an earlier pivot or reset. They might have pivoted the project to a narrower scope (decision support for clinical trial matching, for example, rather than full treatment recommendations) or pivoted to invest much more in data curation and expert knowledge integration after early warning signs in 2014-2015. Oncologists found the system wasn’t adapting well to different hospital contexts and had biases. IBM’s failure to pivot in time – essentially persisting with an over-ambitious approach – turned a high-profile AI venture into a cautionary tale. The lesson: even with big investment and big hype, an AI project should pivot if real-world feedback (from domain experts, in this case) is consistently poor. Pride and publicity shouldn’t keep a misaligned project going.
Failure (or Lesson) – Over-Automation at Tesla: Outside of software, we saw a form of AI/automation pivot at Tesla. Elon Musk attempted to heavily automate the Model 3 production line with advanced robotics and AI coordination, reducing human roles. This backfired initially – the line couldn’t meet production targets, and it turned out some tasks were better done by humans. Musk famously admitted “excessive automation at Tesla was a mistake… Humans are underrated”. Tesla had to pivot by reintroducing human assembly steps and simplifying automation. In essence, the AI/robotics strategy was dialed back – an unusual pivot that involved removing some cutting-edge automation in favor of reliability. This scenario is a reminder that pivoting an AI project doesn’t always mean doing more AI; it can mean finding the right human-machine balance. For manufacturing and operations, the best ROI might come from augmenting skilled workers with AI, not outright replacing them. The success metric is overall throughput and quality, not how “AI-driven” the process is. Tesla learned and recovered, but the experience underscores the importance of adaptability. If a high-tech approach isn’t yielding results, pivot without ego – even if it feels like reverting to an older approach, it’s about what works best in practice.
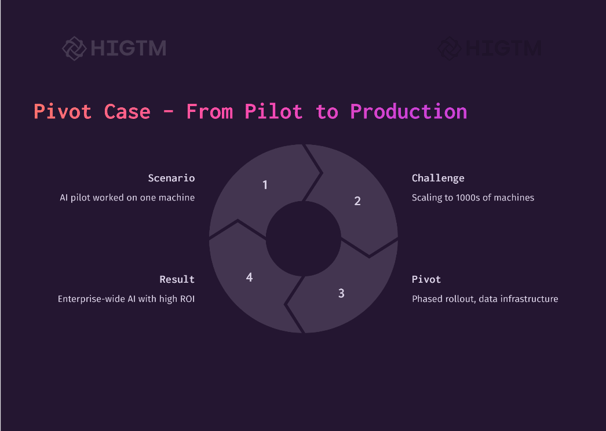
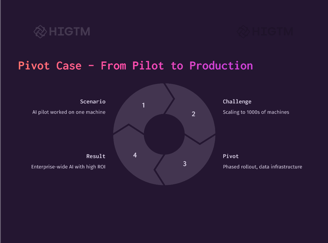
5. Cross-Industry Insights: What Retail (and Others) Can Learn from AI Pivots in Finance, Healthcare, Logistics, etc.
Each industry has its own journey with AI, but the beauty of pivoting and course correction is a universal concept. Here are cross-industry insights into AI pivots, and specifically how retail (a sector undergoing AI transformation in areas like online personalization, supply chain, and in-store experience) can learn from other sectors:
From Finance (Banking & Fintech): Financial services were early adopters of AI for things like algorithmic trading, fraud detection, and customer analytics. A big lesson from finance is the value of governance and explainability. Banks have pivoted AI models when they proved too opaque or risky – for example, pivoting from a black-box credit scoring model to a more transparent one to satisfy regulators and customers. Retailers can learn the importance of trust in AI decisions. If you deploy a price optimization AI and it starts setting prices that anger customers or trigger a PR backlash, be ready to pivot strategy (perhaps introduce human review for certain decisions, or adjust the algorithm’s objectives to weigh customer fairness more). Finance also taught us about “pilot purgatory” – banks often have many innovation labs but few deployments. How did leading banks pivot out? By focusing on integration: choosing fewer projects but fully implementing them with frontline involvement. Retailers exploring AI (say, an AI planogram optimizer for store layouts) should similarly avoid spreading too thin. It could be smarter to pivot from ten trial tools to three that get enterprise-wide rollout with training and support.
From Healthcare: Healthcare emphasizes safety, validation, and human expertise. The failure of IBM Watson in oncology highlighted that domain expertise and context are everything. A pivot insight here for others: narrow the scope to something manageable and build evidence gradually. Some healthcare AI successes (like certain diagnostic image AI or patient risk scoring systems) started very humbly in one hospital department and with extensive peer review before scaling. A retailer could take this cue by piloting an AI in one store or region and really analyzing the outcomes (did it increase sales? improve customer satisfaction?) before rolling it out. If results are mixed, pivot the use-case or parameters rather than launching chain-wide and hoping for the best. Another cross-industry point: healthcare had to pivot many AI projects to align with privacy laws (HIPAA, etc.). Retail now faces its own data privacy challenges – e.g. using AI on customer data under GDPR/CCPA. Learning from healthcare, retail firms should be proactive in embedding privacy compliance from day one, and pivot their data strategies (maybe invest in anonymization or better customer consent practices) as regulations evolve. It’s better to pivot your data handling approach early than to have an AI project halted by a regulator later.
From Logistics & Transportation: This sector is all about efficiency and timing. AI and automation here include route optimization, fleet management, and warehouse robotics. A big pivot lesson from logistics is incremental rollout and fallback options. When automating a warehouse with AI-driven robots, companies like Amazon learned to have contingency plans – if the AI system goes down or proves inefficient in a scenario, operations can revert to manual or an alternate system seamlessly. That’s a kind of pivot-on-demand built into design. Retail operations, say in distribution centers or inventory management, should similarly design AI integrations that can be adjusted or reversed without chaos. If an AI starts mismanaging stock levels, you detect it through metrics and can pivot back to manual control or an improved algorithm quickly. Another insight: logistics firms often start with RPA (robotic process automation) – simple rule-based automation – and only pivot to full AI/ML solutions when the simple approach hits a ceiling. Retailers should not use AI just for bragging rights; if a simpler automation yields 90% of the benefit, use it until diminishing returns, then pivot to ML for the rest. This phased approach ensures each pivot to more advanced tech is justified by clear ROI.
From Manufacturing: Manufacturing has seen pivots around the degree of automation (as Tesla’s case showed) and around predictive maintenance. One insight is the importance of people in the loop. Many factories introduced AI quality inspection but pivoted to a hybrid model when pure AI had too many false negatives/positives – e.g., an AI flags defects and human inspectors verify them, or vice versa. Retail could apply this to, say, AI in customer service: instead of fully automated AI handling of customer emails, have AI do first-pass drafting or triage and humans finalize responses (improving quality while saving time). If a retailer tried full AI customer service and saw complaints rise, a quick pivot to a semi-automated approach can rescue the situation. Manufacturing also teaches about the “one factory at a time” approach to scaling – similar to pilot to production, but specifically, successful firms create a template at one plant, then replicate. Retail could mimic that: develop an AI-driven process in one flagship store (or one business unit), document the heck out of it, refine it, then replicate. If a replication fails, pivot by tweaking the template (maybe the model needs retraining for a different store size, etc.).
In essence, cross-pollination of lessons helps avoid costly pivots by learning upfront, and when pivots are needed, you can execute them more gracefully having seen parallels elsewhere.
6. Best Practices for AI Course Corrections (Maximize ROI, Minimize Disruption)
Executing an AI pivot is a high-stakes exercise. Here are best practices to ensure your pivot leads to a positive outcome with controlled disruption:
1. Re-align with Business Objectives: Before pivoting, reaffirm what business goal the AI project serves. A pivot should bring the project closer to delivering on a key objective (be it increasing revenue, reducing cost, improving customer experience, etc.). Avoid pivots that chase technology trends without a clear business case. It may help to ask, “If we make this change, how will we measure success differently and does that success matter to our bottom line or mission?” For example, pivoting from a customer-facing AI to a backend AI (Scenario #2 above) should be because you expect better customer satisfaction or efficiency, which ties to retention or margins – not just because your team thinks backend is “easier.” Keeping ROI in focus guides the pivot design.
2. Stakeholder Communication and Buy-In: Pivoting an AI project will fail if stakeholders interpret it as a failure or randomly changing direction. It’s crucial to manage the narrative. Communicate to your team and executives why you’re pivoting – use data and examples to show it’s a calculated move. For instance, present that “We found user adoption is only 20%, and feedback indicates a different need, so we are pivoting the AI to address that directly.” This turns a potential critique (“the project didn’t work as first planned”) into a positive (“we learned and are making it better”). Additionally, involve key stakeholders in planning the pivot. Frontline employees, IT staff, data scientists, and compliance officers – each should have input if the pivot touches their domain. This inclusive approach not only catches blind spots (perhaps Legal knows of a regulation impact the pivot could have) but also creates champions who support the new direction. Essentially, treat a pivot like a new project launch in terms of change management.
3. Incremental Pivot with Pilot of its Own: Unless truly urgent, avoid “big bang” pivots where you completely switch the system overnight. Instead, pilot your pivot. If you’re changing an AI’s application, test the new approach on a small scale (e.g., one department, a subset of users or data) while keeping the old system running in parallel if possible. This minimizes disruption and allows you to validate the pivot hypothesis. Suppose you pivot from one AI vendor’s platform to another’s for better performance – run them side by side on a sample for a period. Measure the differences in outcomes and only then cut over fully. This iterative approach to pivoting ensures you don’t swap one problem for another unknowingly. It also gives you a fallback; if the pivot’s pilot reveals issues, you can refine or even roll back. Think of it as A/B testing your pivot path before committing enterprise-wide.
4. Preserve What Works (Reuse and Recycle): Pivoting doesn’t mean starting from scratch. Identify components of the AI project that are successful or could be salvaged. Data pipelines, cleaned datasets, trained sub-models, domain knowledge gained, or integration hooks – these are assets. For example, you might pivot from a complex AI to a simpler automation rule-based system in the short term; but the data collected by the AI or some analytics models can still be used within the new system. Reuse not only saves time and investment, it also reduces change fatigue for users (since not everything changes at once). It’s also a morale boost to the team: acknowledging that their work wasn’t all in vain, but rather is being applied differently. A practical tip: maintain modular architecture in AI projects. If components are loosely coupled, you can replace or repurpose one part (say the front-end interface, or the ML model) without rebuilding the entire stack. That makes pivots far less onerous technically.
5. Document Learnings and Update Governance: After a pivot, document why it happened and what was learned. Feed this back into your AI governance framework or project playbooks. Maybe your company’s AI strategy gets an update: e.g., “In initial projects we learned customer-facing AI should always have a human fallback – that’s now our standard.” By codifying these lessons, future projects benefit and may avoid needing similar pivots. It also helps in auditing and accountability – stakeholders understand decisions made and the rationale, which is useful for compliance and historical record. Moreover, widely sharing pivot success stories (and even failures) within the organization builds a culture that accepts and expects iteration. People become less fearful to flag issues (“Hey, this AI isn’t working as we thought”) because they see examples where pivoting led to success. As Zillow’s tech executive noted, it’s okay to make mistakes as long as you learn and pivot quickly. Making that a celebrated part of your culture is a best practice that pays dividends beyond a single project.
6. Partner with Experts if Needed: Sometimes a project hits a wall because of internal blind spots. Engaging external experts or consultants during a pivot can provide fresh perspective. Major vendors like Microsoft, Google, AWS, and IBM have their own solution architects who’ve seen countless deployments – tapping into their knowledge can inform your pivot (perhaps another client had a similar issue and solved it in a novel way). Likewise, bringing in a specialized AI consultancy (such as HI-GTM, to give an example) can accelerate a pivot by auditing your project and recommending the best path forward. They might see that a different algorithm, a different data strategy, or a different way of measuring success is needed. While not every pivot justifies external help, high-impact ones – say you’re overhauling an enterprise AI strategy due to new regulation or scaling up 10x – often do. The objective viewpoint and experience base can validate your pivot plan and even run interference, reducing risk as you implement the changes.
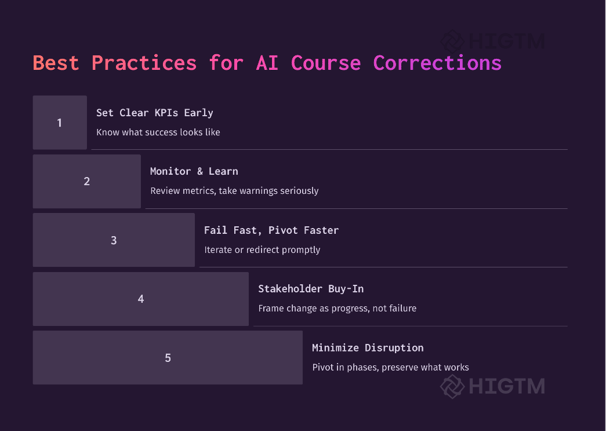
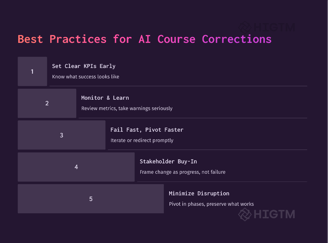
7. Conclusion: Embracing Pivoting as a Strategy, Not an Emergency
The narrative around AI projects is often “plan well, execute, and you’ll succeed,” but the truth in enterprise settings is more nuanced. Even with good planning, AI initiatives are exploratory by nature – you uncover new information, and conditions change. Therefore, treat pivoting as a natural and proactive strategy rather than a last-resort move. By building continuous monitoring and feedback loops, you can anticipate pivots (sometimes making minor adjustments continually so you don’t need a dramatic pivot at all). When larger pivots are needed, a company that has a culture of agility will handle them decisively and effectively.
The ultimate measure of success is not whether you avoided ever changing course; it’s whether the AI delivers value and advances your business goals in the end. If a mid-course correction is what it takes, then making that call is a sign of strength, not weakness. Executives should empower their teams to surface candid insights and options, even if it means admitting the current approach isn’t perfect. As we’ve seen, some of the biggest names (from IBM to Amazon to Tesla) faced setbacks when they didn’t pivot quickly enough or had to learn publicly why a pivot was needed. Meanwhile, many quiet success stories – in finance, retail, tech – happened because someone noticed a trend or risk and steered the AI project to a better path.
In summary, AI project pivots, done right, maximize ROI and minimize waste. They ensure your AI efforts remain aligned with a moving target: business value. Whether you’re transitioning from predictions to automation, moving an AI out of the spotlight to work its magic behind the curtains, adjusting for new rules, or scaling up a pilot, the ability to pivot is what turns AI from a gamble into a strategic asset. Keep your eyes on the data, stay humble about initial assumptions, and be fearless in redirecting efforts to where they matter most. Your organization will then view AI not as a rigid plan to execute, but as a journey where learning and adapting lead to ultimate success.
(Interested in assessing or pivoting your AI projects for better results? Consider reaching out to HI-GTM’s consulting team – we specialize in guiding businesses through AI strategy refinements to achieve Go-To-Market excellence.)
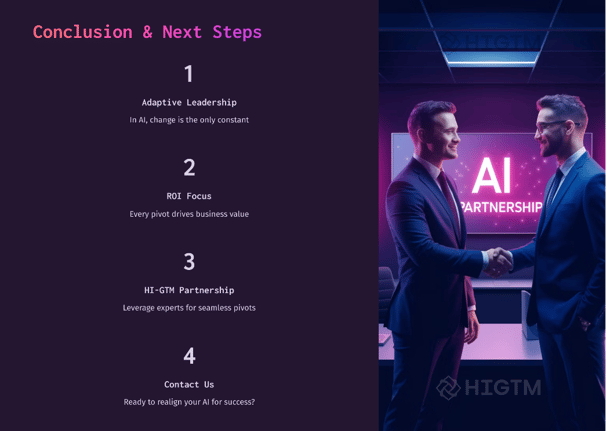
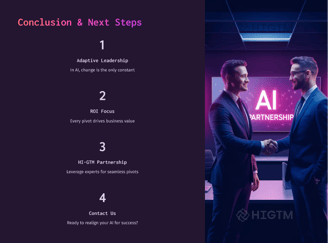
Turn AI into ROI — Win Faster with HIGTM.
Consult with us to discuss how to manage and grow your business operations with AI.
© 2025 HIGTM. All rights reserved.