83. Long-Term Roadmapping – From Pilot to Enterprise Adoption (AI in Retail Strategy)
Most retail businesses have dabbled in AI, often launching a pilot project or two to test the waters. But dabbling isn’t enough. To truly reap the benefits, companies need a long-term AI roadmap that guides them from those initial pilot programs all the way to enterprise-wide AI adoption. This roadmap is a strategic plan that ensures small experiments grow into transformative solutions across the entire business. In this article, we’ll explore how retail executives can chart this journey, avoid common pitfalls, and accelerate AI adoption at scale with confidence.
Q1: FOUNDATIONS OF AI IN SME MANAGEMENT - CHAPTER 3 (DAYS 60–90): LAYING OPERATIONAL FOUNDATIONS
Gary Stoyanov PhD
3/24/202524 min read
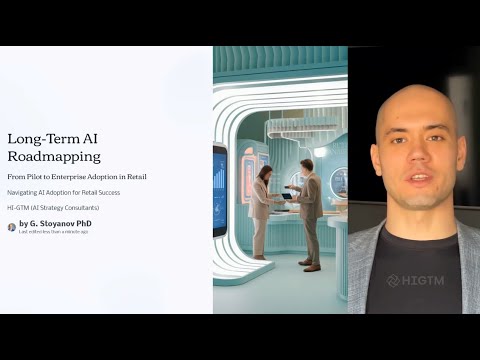
1. Introduction to Long-Term AI Roadmapping
Retailers large and small are already witnessing the promise of AI. A recent survey showed that 89% of retail organizations are either using AI in operations or at least piloting AI projects. From predicting fashion trends to managing inventory in real-time, AI’s potential in retail is vast. However, there’s a stark reality check: while pilots are common, successful enterprise adoption is rare – only a fraction of companies manage to fully scale AI beyond isolated projects. This gap between experimentation and transformation is where long-term planning becomes crucial.
Why a roadmap? Just as you wouldn’t build a new store without an architectural blueprint, you shouldn’t scale AI without a clear plan. A long-term AI roadmap helps align stakeholders, secure budgets, develop talent, and integrate technologies step by step. It turns a vision into a sequence of achievable milestones. In the sections that follow, we outline the key phases of AI adoption in retail – from selecting the right pilot use cases to rolling out AI across the enterprise. We’ll also highlight strategies, case examples, and best practices to ensure your AI investments deliver sustainable, compounding returns.
2. The Retail AI Opportunity and Urgency
Every retailer today faces a pressing question: How can we leverage AI to stay competitive and delight customers? The opportunity is enormous. AI can unlock insights and efficiencies at a scale impossible for humans alone, especially given the complexity of modern retail (think of managing thousands of SKUs, supply lines, and personalized marketing for millions of customers). Early adopters of AI in retail are pulling ahead, creating a widening gap between AI leaders and laggards.
Consider some transformative impacts already observed: personalization algorithms boosting e-commerce sales by curating the right products for the right people, machine learning models reducing excess inventory by accurately forecasting demand, and computer vision systems virtually eliminating checkout lines with cashier-less technology. These are not science fiction; they are happening now in retailers like Amazon, Walmart, and Alibaba, who invest heavily in AI. Moreover, surveys indicate 87% of retailers see AI positively impacting revenue, and 94% report AI is reducing operating costs – a clear signal that well-implemented AI pays off.
The urgency to act is underscored by competitive dynamics. If your closest competitor manages to roll out AI-driven dynamic pricing and you’re still manually updating prices, you will be at a disadvantage. If they use AI to optimize supply chain logistics, they can deliver faster or avoid stock-outs that might plague your operations. In short, the cost of inaction grows every day. However, acting without a plan can be just as risky – poorly managed AI projects can waste resources or even backfire (for example, implementing a bot that ends up disappointing customers due to lack of training). This is why a strategic roadmap is essential: it allows you to seize the AI opportunity methodically and responsibly.
3. Phase 1 – Pilot Projects: Laying the Foundation
3.1 Selecting High-Impact Pilot Use Cases (Start Small, Win Big)
The journey begins with choosing the right pilot project. A pilot is a limited-scope implementation of an AI solution aimed at solving a specific problem or achieving a quick win. In retail, ideal pilot candidates often lie in areas with abundant data and clear KPIs. For example, you might start with an AI-driven demand forecasting tool to address overstock and stockout issues, or deploy a customer service chatbot to handle common inquiries online. The key is to pick a use case that is strategically relevant but manageable in scope. It should address a pain point where improvement can be measured (e.g., forecasting accuracy, customer satisfaction scores, response times) within a few months.
When selecting the pilot, also assess feasibility: do you have the data required to train the AI model? Is there a champion on the team excited to run the experiment? Ensure the pilot has access to necessary resources (data engineers, a small budget, support from IT). A successful pilot will not only solve a problem, it will generate enthusiasm and credibility for AI within the company. That’s why it’s often wise to “start small, but think big.” Choose a pilot that, if successful, could be scaled to other parts of the business.
3.2 Defining Success Criteria and KPIs
Before diving into development, define what success looks like for your pilot. Clear metrics will keep the project focused and provide evidence for future scaling. For a demand forecasting pilot, success might be defined as “reduce excess inventory by 20% in the test category” or “increase in-stock rates to 98% for top products.” For a chatbot, it could be “automate 50% of customer queries within the first minute, with a customer satisfaction rating of 90% for those interactions.” Establish a baseline (current performance without AI) and target improvement.
It’s also important to set a time frame for evaluation – say, a 3-month pilot period. Use this period to gather data on performance, gather user feedback (store managers, customers, employees, etc.), and work out kinks in the solution. If the pilot doesn’t meet the targets, analyze why: Was the model inaccurate? Did users not engage with it? These insights are valuable whether you decide to pivot, improve, or even shelve that particular solution. Remember, not every pilot will be a home run, but every pilot should be a learning experience.
3.3 Execution with Agility
During the pilot phase, treat it as an agile project. Iterate quickly based on feedback. If you’re piloting an AI recommendation engine on your website, monitor how customers interact in real time. If certain recommendations are clearly not working (for example, recommending winter coats to someone shopping in summer), refine the algorithm or rules on the fly. Keep the pilot scope limited so that you have the flexibility to tweak and improve rapidly. Regular check-ins with stakeholders (like weekly or bi-weekly updates) can keep everyone aligned and excited.
It’s crucial to document the process and results. When the pilot concludes, you want a solid report: what was done, what the outcomes were versus the baseline, and what the key lessons are. This documentation will be gold when making the case to senior leadership about moving to the next phase. It shows professionalism and provides a knowledge base for future projects. At the end of Phase 1, you should have either a validated solution ready for broader use or a clear understanding of why it didn’t meet objectives (and whether that issue can be fixed in a second iteration).
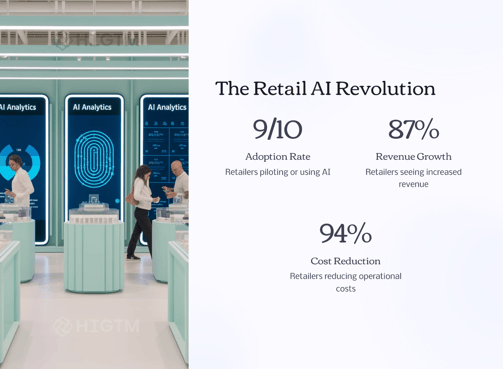
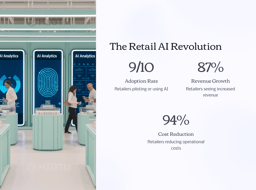
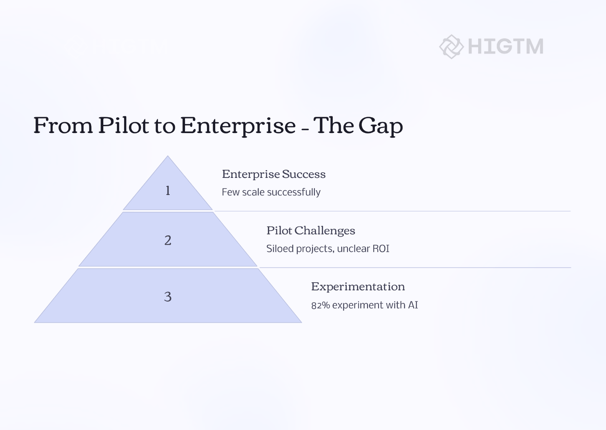
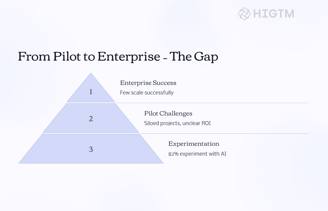
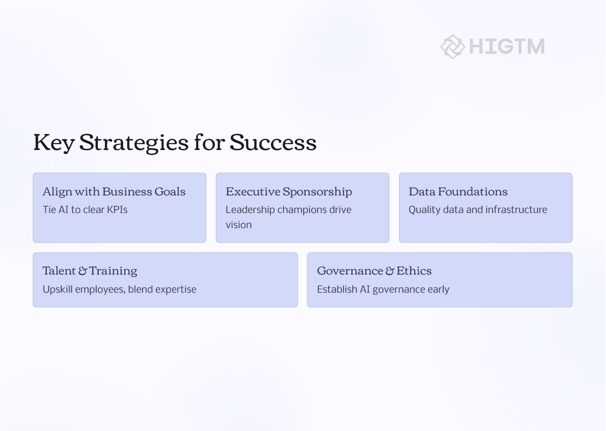
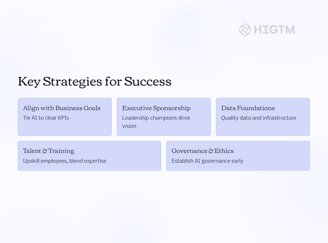
4. Phase 2 – Scaling Up: From Pilot to Program
4.1 Gaining Executive Buy-In and Budget for Scale
Once a pilot demonstrates promise (or better yet, clear success), it’s time to shift into scale mode. Transitioning from a small pilot to a larger implementation often requires fresh investment and cross-functional coordination. This is where executive sponsorship becomes vital. Hopefully, you’ve kept key leaders apprised of the pilot’s progress; now you will present them with results and a proposal for scaling. Focus on the ROI and strategic benefit: for instance, “Our AI forecasting pilot saved $500K in inventory costs in one category; scaling it to all categories could potentially save $5M annually.” Back your proposal with data from Phase 1 and a plan that outlines resource needs (budget for software, perhaps hiring data scientists or engaging an AI consulting partner like HI-GTM) and a timeline for rollout.
Obtaining buy-in isn’t just about money – it’s about permission to disrupt the status quo. Enterprise scaling will affect more teams and possibly change workflows for many employees. Having the CEO, CIO, or other C-suite champions actively endorse the AI program will smooth this path. Communicate a clear vision of the scaled solution: help everyone visualize what the business will look like when the AI isn’t just in one pilot area, but across the organization delivering value.
4.2 Building the AI Coalition (Cross-Functional Teams)
As you scale, you’ll need to involve more people. One best practice is forming a cross-functional AI task force or committee. This group could include the pilot team members, IT representatives, operations managers, a few front-line users, and certainly someone from data governance or security. The idea is to bring together all stakeholders who will be impacted by or have expertise relevant to the AI rollout. This coalition helps in several ways: it ensures you address integration with existing systems (IT’s concern), consider process changes on the ground (operations concern), manage data privacy and quality (governance concern), and drive user adoption (change management concern).
For example, if scaling an AI chatbot to all customer service channels, involve your call center manager, your web/app product manager, IT for integration with the CRM, and HR or training leads to plan how customer service reps will collaborate with the chatbot. Regular workshops or stand-up meetings with this group will identify potential issues early and create buy-in, since each department feels represented. Essentially, you’re creating internal champions across the company who will advocate for the AI initiative within their teams.
4.3 Technology and Infrastructure Scaling
A pilot might run on a small dataset or a standalone cloud instance; scaling often means beefing up the technology stack. This could involve migrating the AI solution to a more robust cloud environment (ensuring it can handle more data and more requests), integrating with databases or enterprise software (so AI outputs seamlessly flow into dashboards or operational systems), and setting up monitoring and maintenance processes. It’s at this stage you might formalize things like an MLOps pipeline – automated processes for retraining models, deploying updates, and monitoring performance drift over time.
Retailers should evaluate if their current technology infrastructure can handle scale. For instance, if you ran a pilot on a local server, you might now move it to a scalable cloud service like AWS SageMaker, Google Cloud AI, or Azure ML. Many major AI vendors provide tools specifically for scaling – like auto-scaling clusters, data pipeline services, etc. Ensure data pipelines are robust: if your pilot pulled data from one store’s point-of-sale, scaling up might mean pulling from every store daily. Does your network and data warehouse support that? These considerations are critical to avoid a scenario where the AI works in a sandbox but crumbles under real-world volumes.
4.4 Iterate and Expand Use Cases
Phase 2 is also about broadening the horizons. While you work on scaling the original pilot to broader use (vertical scaling), you can also consider starting new pilots in parallel (horizontal expansion), leveraging the growing expertise and enthusiasm. For example, while the data science team is busy scaling the demand forecasting AI globally, perhaps another team begins piloting an AI-based visual recognition system for planogram compliance in stores. By sequencing pilots and scales in a pipeline, you create a continuous innovation engine. Just be careful not to over-extend; ensure each new pilot still aligns with the overall AI strategy and that you have the capacity to support it.
Throughout Phase 2, maintain an iterative mindset. As you roll out to new departments or stores, gather feedback and performance data. You might find the AI model needs retraining with more diverse data, or that users in a new context use the tool differently. Be ready to make continuous improvements. Scaling isn’t a copy-paste of the pilot; it’s an evolution. Keep communication lines open – let the wider organization know of early wins as you scale (e.g., “after 3 months, all 50 stores in region X are using the AI system and saw a 10% sales uptick”), which builds momentum and appetite for more AI adoption.
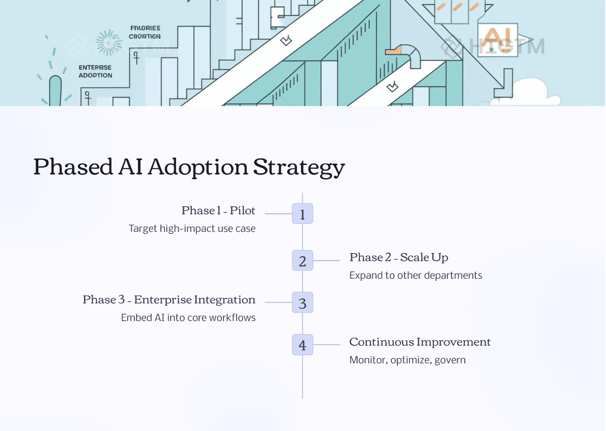
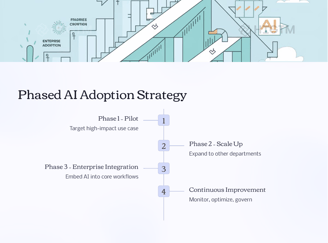
5. Phase 3 – Enterprise Adoption & Integration
5.1 Integrating AI into Core Business Processes
Enterprise adoption means AI is now an integral part of how you do business. It’s no longer a novelty or separate project. In Phase 3, the focus is on integration – technically and operationally. This often involves embedding AI outputs into decision-making processes and workflows. For example, your merchandising team might now use the AI demand forecast as the default basis for their buying decisions each season, or store managers might receive staffing suggestions from an AI system that predicts foot traffic.
To achieve this, ensure AI systems interface smoothly with the tools employees use daily. That could mean integrating AI recommendations into your ERP system, your CRM dashboards, or custom mobile apps for employees. This way, using the AI is as simple as clicking a familiar report or receiving an alert – no separate logins, no clunky exports/imports. AI adoption will flourish if it makes everyone’s job easier without adding complexity.
Process integration also means adjusting SOPs (Standard Operating Procedures). Rewrite parts of your playbooks: if the old process said “manager decides inventory levels based on experience,” the new process might be “manager reviews AI-generated inventory proposal and approves/adjusts based on local insights.” In essence, human-AI collaboration becomes the standard. You want to institutionalize the role of AI so it’s sustained even if individual champions move on.
5.2 Change Management and Cultural Adoption
The human side of enterprise AI adoption is often the toughest. By Phase 3, many employees will experience AI in their day-to-day work. Change management is paramount. Communicate the why behind each AI tool – how it helps the employee and the company. Provide ample training sessions, not just on how to use the systems, but on basic AI literacy: explain what the AI does, how it arrives at recommendations (to the extent possible), and what its limitations are. This demystifies the technology and builds trust.
It’s also important to address fears openly. Some employees may worry that AI will replace their roles. Leadership should reinforce that AI is there to augment human capabilities – to handle tedious tasks, crunch data, and offer insights – while humans focus on creativity, judgment, and customer interaction. In retail, the best outcomes often come from AI+Human synergy, for instance: an AI model predicts what a local store needs, but the store manager, with community knowledge, makes the final call, leading to optimal results. Celebrate stories of employees who used AI to achieve great results, making them internal heroes of digital transformation. This encourages others to embrace the tools rather than resist them.
A practical tip is to implement AI gradually in each team, if possible. Start with volunteers or early adopters who can be evangelists. They can coach peers and provide support. Make feedback loops easy – perhaps a quick survey or Slack channel where staff can say “Hey, the new recommendation system suggested something weird, can someone check it?” and get responses. Show that the organization listens and improves the AI tools based on user input, which in turn increases user buy-in.
5.3 Governance, Ethics, and Oversight
With great power comes great responsibility. As AI permeates the enterprise, governance and ethical considerations move to the forefront. Retail data often includes personal customer information, and AI decisions can affect real lives (e.g., who gets a discount offer, how customer complaints are resolved by an AI agent, etc.). It’s critical to have an AI governance framework: guidelines and committees to oversee AI use, ensure compliance with regulations like GDPR or CCPA for customer data, and uphold ethical standards (for example, ensuring your AI isn’t unintentionally biased against certain customer groups or regions).
Establish clear data policies, like how customer data is used for AI and how models are validated for fairness. You might set up an AI ethics board or designate certain officers (like a Chief Data Officer or AI lead) to periodically review algorithms. In one scenario, a retailer’s AI pricing tool could inadvertently start charging higher prices in low-income neighborhoods due to correlation with purchasing behavior – an ethical red flag if not caught. Oversight mechanisms, such as audits of AI outcomes and bias testing, help prevent such situations and maintain trust with customers and stakeholders.
Security is another aspect: AI systems should be secure from breaches or manipulation (imagine the chaos if someone tampered with your inventory AI to cause stockouts). Work closely with your IT security team to protect sensitive models and data. Document decisions – who approved an AI model for deployment, what checks were done – to create traceability. By Phase 3, you’re treating AI with the same seriousness as any mission-critical process, subject to internal controls and continuous monitoring.
5.4 Continuous Improvement and Innovation
Reaching enterprise adoption is a major milestone, but it’s not the end. In fact, it’s a new beginning of continuous improvement. AI models can degrade over time as trends change (a phenomenon known as “model drift”). For example, your sales forecast AI might become less accurate if a new competitor enters the market or if consumer behavior shifts year-over-year. Therefore, set up a schedule to retrain models with fresh data, perhaps every quarter or season, and to reevaluate if the features and algorithms used are still the best.
Also, encourage a culture of innovation. Now that AI is part of your DNA, how else can you use it? Maybe venture into new use cases that weren’t feasible early on. Perhaps explore generative AI for creating marketing content, or advanced image recognition for shelf-checking in stores. Keep an eye on industry trends: what are others doing? A framework like HI-GTM’s consulting can be valuable here – external experts can provide an outside-in perspective on new AI opportunities or optimizations you might not see, and ensure you remain at the cutting edge.
Finally, measure, measure, measure. At the enterprise level, you’ll want to track high-level KPIs like overall cost savings from AI this year, revenue uplift attributed to AI-driven campaigns, improvements in customer satisfaction, etc. Share these wins with the entire company – it reinforces the value of the AI transformation. Also share learnings from any failures or near-misses; transparency keeps the organization realistic and prepared. The roadmap in fact never truly ends; it evolves. Your long-term roadmap will extend further into the future as you add new legs to the journey – perhaps moving from traditional AI into newer frontiers like quantum computing or more sophisticated robotics in stores when the time comes. The key is, you have built a resilient, agile, AI-enabled enterprise that can adapt and thrive as technology and markets evolve.
6. Key AI Use Cases in Retail to Scale Across the Enterprise
One of the strengths of AI in retail is its versatility – it can enhance a wide array of functions. Here we outline several major AI use cases that retail executives should consider in their long-term roadmap. Many of these start as pilots in one area and eventually become enterprise-wide programs:
Personalized Customer Experiences: Perhaps the most visible AI application is personalization. This includes product recommendations on e-commerce sites (“Customers who bought this also bought that”), personalized email marketing, and targeted promotions. AI algorithms analyze browsing behavior, purchase history, and demographics to tailor the experience. For example, Amazon’s recommendation engine, one of the earliest and most famous AI systems in retail, drives a significant portion of its sales. Piloting personalization can start on a small subset of customers or a single category, then expand to omni-channel personalization (website, mobile app, email, in-store clienteling via associate apps). The end goal is a unified 360-degree customer profile informing every interaction.
Inventory Management and Demand Forecasting: Inventory is the lifeblood of retail, and getting the right balance is notoriously difficult. AI excels at detecting patterns in sales data, seasonality, local events, and even weather that influence demand. Retail giants and grocers use AI to forecast demand at the store and SKU level with higher accuracy than traditional methods, directly reducing overstock and stockouts. A pilot might involve one region’s data or a specific product line; scaled up, it becomes an automated system adjusting orders and distribution for the entire company. Some retailers also deploy AI-powered auto-replenishment, where the system triggers restocks to stores autonomously up to certain limits, freeing planners to handle exceptions.
Dynamic Pricing and Promotion Optimization: AI can adjust prices in real time based on supply, demand, competitor pricing, and customer segments. This is especially popular in online retail and for categories like electronics or travel (think airline ticket pricing algorithms). In traditional retail, dynamic pricing might be used more cautiously, but we see examples like supermarkets using electronic shelf labels to update prices or discounts throughout the day (e.g., marking down perishable goods as closing time nears). Piloting this could be in an e-commerce environment or a small set of stores, and scaling means integrating with pricing systems and perhaps rolling out new pricing infrastructure (like those electronic labels). The ROI can be significant: selling more at optimal prices and clearing inventory efficiently.
AI-Powered Customer Service (Chatbots & Virtual Assistants): Many retailers have introduced AI chatbots on their websites or messaging platforms to answer FAQs, assist with orders, or even make product recommendations. These bots, often powered by NLP (Natural Language Processing) and sometimes branded with a friendly persona, operate 24/7, providing instant support. A pilot chatbot might start with answering a specific category of questions (say, order tracking or store hours) and then evolve to handle more complex tasks (help with product selection, processing returns). At full scale, a sophisticated virtual assistant could be unified across channels – website chat, mobile app, and even in-store kiosks – offering a consistent helper to customers wherever they are. Importantly, these AI agents can hand off to human agents when needed, creating a co-working dynamic. Companies like H&M have used chatbots for style advice, and Sephora’s chatbot helps customers choose makeup – showing how AI can augment the sales process.
In-Store Analytics and Operations: Physical retail locations benefit from AI through computer vision and IoT. Examples include cameras that monitor shelf stock levels or detect shoplifting, sensors that track customer movement to optimize store layouts, and even AI that optimizes in-store music and digital signage based on customer demographics. Pilots here might involve outfitting a single “smart store” with these technologies to gauge impact on sales or loss prevention. If successful, the chain can roll out these capabilities more broadly. Another growing area is autonomous checkout (like Amazon Go stores) where a combination of sensors and AI identifies what customers pick up and charges them automatically – usually piloted in a few locations before wider deployment due to cost. While not every retailer will go full cashierless, elements of that tech (like smart shopping carts or scan-and-go apps) are scalable components.
Supply Chain and Logistics Optimization: Retail AI isn’t just customer-facing – it’s also behind the scenes in warehouses and distribution. AI can optimize delivery routes (saving fuel and time for fleets), predict delays or disruptions in the supply chain, and manage warehouse automation robots. A pilot could be using AI routing for one city’s deliveries; scaling up means your entire logistics operation is AI-optimized. Retailers like Walmart use AI to manage their trucking routes and loading, and UPS’s ORION system (while not retail, it’s a famous case) saved millions of miles by AI-optimizing routes. As sustainability becomes important, AI is also used to reduce waste and energy (e.g., optimizing refrigeration or lighting based on usage patterns in stores).
Each of these use cases may start in isolation, but the ultimate vision (and a key benefit of enterprise adoption) is when they work together. Imagine a future where your demand forecasting AI tells your supply chain AI about a coming surge, which in turn nudges your dynamic pricing AI to adjust, and alerts your marketing AI to prepare campaigns – all while your chatbot AI informs customers proactively if something is out of stock and suggests alternatives. This interconnected AI ecosystem is the end-game of a long-term roadmap, where the whole is greater than the sum of the parts.
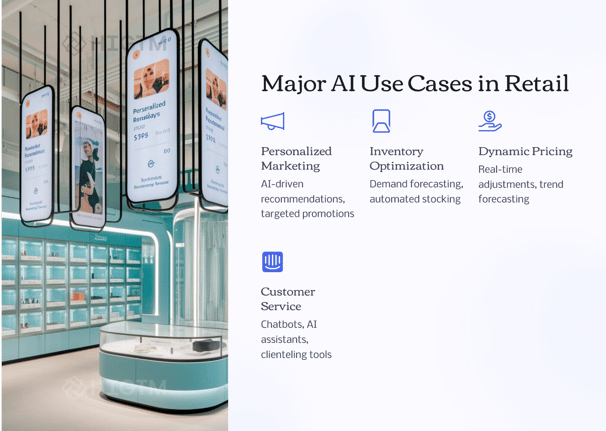
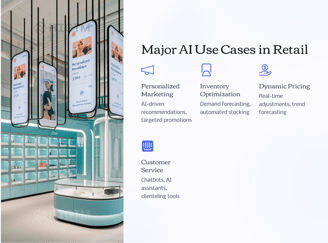
7. Best Practices and Strategies for Scaling AI Successfully
Scaling AI is as much an organizational challenge as a technical one. Here are some best practices and strategies distilled from successful AI adoptions:
7.1 Avoid the “Pilot Trap”
Many companies fall into the trap of running numerous AI pilots that never graduate to production. To avoid this, be very intentional: from the outset of a pilot, have an expansion plan in mind. Set criteria for when a pilot will be considered for scaling (e.g., “If we achieve X% improvement, we will seek budget to implement in 5 more stores.”). Don’t run too many pilots at once without scaling the winners – otherwise you accumulate a lot of prototype systems that don’t create real value. It’s better to fully deploy one successful AI project than to tinker with five forever. In essence, focus and follow-through are key. Ensure every pilot has an executive “sponsor” whose job is to push it forward if results are good.
7.2 Modular Design for Flexibility
When building your AI solutions, design them in a modular way anticipating future needs. For example, if you develop a forecasting model for one product line, build the system so it can ingest other product data with minimal fuss. Use scalable cloud architecture and standard APIs. This way, scaling to new data sources or higher volume is more of a flip-the-switch than a rebuild. Modularity also helps with maintenance – components can be updated or replaced (like swapping in a new algorithm) without overhauling everything. Many companies adopt a microservices approach for AI deployment, encapsulating each AI capability as a service that other applications can call.
7.3 Talent Development and Training
Your AI projects will only go as far as your people take them. Invest in training programs for different levels of your organization. Train executives on AI basics and strategic thinking (so they can dream up new applications and understand risks), train managers on integrating AI into their workflows and decision-making, and train frontline staff on using AI-powered tools effectively. Also encourage building internal communities of practice: maybe a monthly brownbag where teams share their AI project experiences or a Slack channel for AI enthusiasts within the company. This fosters knowledge sharing and keeps momentum. Additionally, consider rotating some staff through an “AI fellowship” – short stints in the data science or IT teams to get exposure. It breaks down the us-vs-them barrier between technical teams and business units.
7.4 Leverage External Expertise
Sometimes scaling requires help from outside. Don’t shy away from using consultants or vendor services especially in areas where your team lacks experience. Firms that specialize in AI strategy (like HI-GTM) or technical implementation can accelerate your progress, helping you avoid pitfalls others have faced. They can provide frameworks, workshops, and even help build initial versions of systems. Likewise, use the resources of major AI vendors: for instance, if you use Microsoft Azure, engage their cloud solution architects or AI specialists (big providers often have programs to support enterprise customers in AI adoption). Another avenue is partnering with startups through accelerator programs or pilots – many retailers run innovation labs that scout AI startups for solutions like visual search or voice commerce and collaborate to pilot and scale them.
7.5 Measure ROI and Communicate Success
As you scale, keep a sharp eye on return on investment (ROI). AI projects can sometimes drift into R&D land without clear business impact, which endangers long-term support. Define financial or operational metrics for every major rollout. Track them and report them. If your AI scheduling tool saved managers 10 hours a week, quantify that in dollar value of time, and perhaps how those saved hours improved sales or customer experience. Sharing these wins in concrete terms builds the business case for further AI investment. It also helps identify what’s not working – maybe one use case isn’t paying off as expected, which is a signal to revisit the approach or allocate resources elsewhere.
7.6 Maintain Ethical Standards
We discussed ethics in integration; as a best practice, bake ethics and responsibility into the project lifecycle. When designing a new AI solution, ask questions like: Could this negatively impact any group of customers or employees? Are we protecting privacy adequately? What’s our recourse if the AI makes a mistake? Having these discussions early and often ensures you scale responsibly. A public relations hiccup due to an AI error (like an insensitive automated message or biased decision) can derail enthusiasm and trust. Proactively avoiding those through diverse teams and ethical reviews is part of scaling safely. Being an ethical AI leader also becomes a brand advantage – customers and partners trust you more.
7.7 Stay Agile and Adaptable
Finally, keep the mindset that the roadmap can evolve. New technologies (like the surge of generative AI tools in recent times) can open up opportunities or require course corrections. For instance, an AI that was state-of-the-art two years ago might be outdated by a new approach – be ready to pivot, experiment, and upgrade. The companies that succeed with AI long-term are those that remain curious and not complacent. Use pilot projects not just at the beginning, but throughout, to test emerging ideas even as you are scaling others. This creates a pipeline of innovation where some teams scale proven solutions while others scout the next big thing.
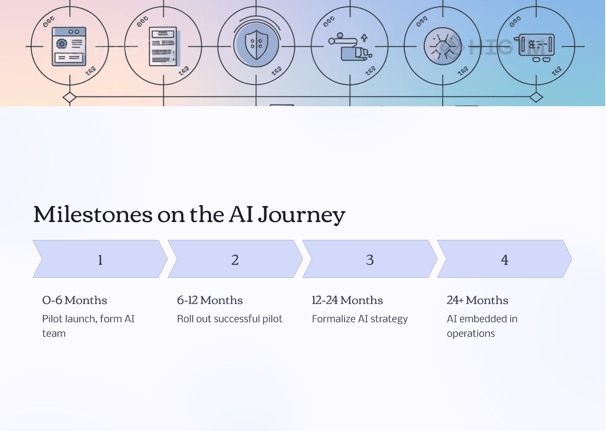
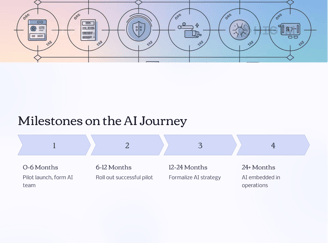
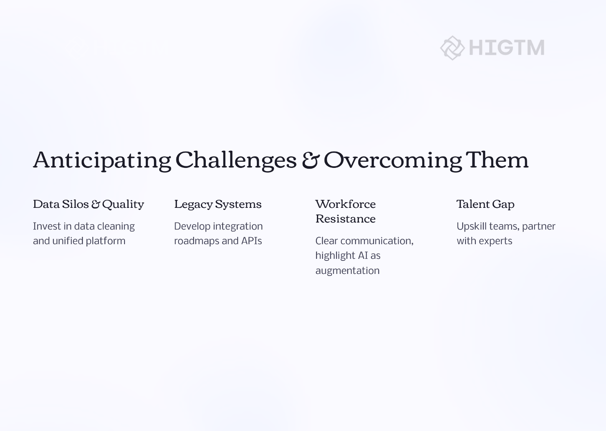
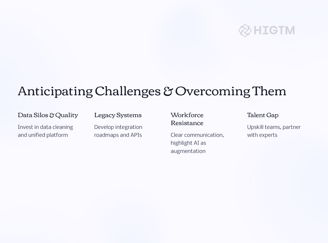
8. Case Study: RetailCo’s Journey from Pilot to AI-Powered Enterprise
To illustrate the roadmap in action, let’s walk through a fictional case study inspired by real retail transformations.
Background: RetailCo is a mid-sized retailer with 100 stores and an e-commerce presence. They were facing stiff competition and margin pressures. In 2023, they decided to invest in AI to improve operations and customer experience, but they had no prior AI projects.
Phase 1 (Pilots): RetailCo’s leadership, with guidance from HI-GTM consultants, identified two pilot projects to start with: (1) an AI-driven inventory optimization for one region’s stores, and (2) an online personalization engine for the e-commerce homepage. The inventory pilot aimed to reduce stockouts in 10 test stores by predicting demand more accurately for 50 high-margin products. The personalization pilot would show product recommendations to a segment of website visitors to increase average cart value.
They set success metrics: a 15% reduction in stockouts and a 5% increase in online sales from recommended products. Small teams were formed. In 4 months, the pilots showed positive results – stockouts dropped 20% in pilot stores, and online test users responded well, with a 7% sales lift. Equally important, store managers and the e-commerce team gave good feedback: the systems were user-friendly and indeed made their jobs easier.
Phase 2 (Scaling): Buoyed by pilot success, the CEO authorized a budget of $1 million to scale these solutions. A cross-functional “AI Taskforce” was created, including IT, store ops, merchandising, and marketing folks. RetailCo chose a cloud platform (Azure) to roll out the inventory AI to all 100 stores, integrating it with their central inventory management software. Over 6 months, they phased the rollout region by region, carefully training store managers on how to interpret and use the AI suggestions for ordering. Meanwhile, the personalization engine, initially for the homepage, was expanded to the entire site and mobile app – now recommending products on product pages, in marketing emails, and even feeding into targeted Facebook ads.
During scaling, they encountered a hiccup: the inventory AI initially struggled in one region due to an unexpected local event (a new competitor store opened nearby affecting demand). The taskforce noticed the anomaly, and data scientists retrained the model including competitor data and promotional calendars. This improved the robustness. The incident proved the value of having humans in the loop and responsive processes.
Additionally, RetailCo started a third pilot in this phase: a customer service chatbot for their call center, to handle simple inquiries. This was planned to complement the other projects, showing an example of parallel pilot-scale tracks.
Phase 3 (Enterprise Adoption): By the end of the year, AI-driven inventory management was the norm in all stores. It became embedded in the daily routine: every morning, store managers checked the AI’s replenishment suggestions and approved orders with a click. The company’s SOP manual was updated to reflect this new process. Inventory metrics company-wide improved – they saw overall inventory levels drop (less overstock) while maintaining or improving product availability. That freed up cash flow and reduced clearance markdowns.
The personalization AI was fully integrated into RetailCo’s marketing operations. The marketing team used its insights for planning campaigns, and the e-commerce team treated it as the “brains” behind site merchandising. Sales attributed to AI-driven recommendations grew significantly, and customer feedback on the online experience was positive (“I keep finding things I love on your site!”).
Culturally, RetailCo embraced AI as part of its identity. The CEO regularly talked about their “AI-enhanced decision making” in town halls. They ran internal training dubbed “AI 101 for RetailCo” so even store clerks and new hires understood the basics of how AI was used in the company. The fears some associates had at the start (“Will this AI take my job?”) eased as they saw these tools actually made their work smoother – stock arrives on time, customers are happier with fewer complaints about missing items, etc. The customer service reps, for instance, found the new chatbot handled the repetitive queries about order tracking, letting them focus on complex customer issues that needed a human touch.
RetailCo also established an AI governance committee under their CIO. This group set guidelines like how to handle data privacy (they decided not to use personalized data in ways customers didn’t agree to), and they reviewed AI outcomes for fairness. One such review ensured that the personalization algorithm was not inadvertently excluding certain product categories (early on, it over-recommended electronics and under-recommended eco-friendly products – they adjusted it to be more balanced, aligning with RetailCo’s brand values of sustainability).
Results: Two years into the journey, RetailCo’s efforts paid off. They saw a 10% increase in overall sales with significant contribution from AI-driven initiatives, and a 5% improvement in profit margins partly due to inventory efficiency and better-targeted promotions. Perhaps just as importantly, they gained a reputation as an innovator in their market segment. Customers started to notice the improved experience (“It’s like they always have what I need, and shopping online feels curated just for me”). Employees, having gone through the change, became proud ambassadors of the new tools. One store manager said, “I can’t imagine going back to gut-feel ordering; the AI system has become my trusty planning partner.”
RetailCo didn’t stop there – they now had a roadmap committee constantly evaluating new AI projects, like visual search for products (snap a photo of an item and find similar products) and using sensors to optimize energy usage in stores. They successfully navigated from zero AI to a full-fledged AI-powered enterprise by following a thoughtful, phased roadmap and focusing on people and processes as much as technology.
9. Conclusion and Next Steps: Achievable with the right approach
The journey from pilot to enterprise AI adoption is challenging, but as we’ve discussed, it’s absolutely achievable with the right approach. Long-term roadmapping is the compass that guides you through this journey. For retail executives, the message is clear: start now, start smart, and think big. A small pilot today can evolve into a competitive differentiator tomorrow – but only if you plan for scalability and integrate those successes into the fabric of your business.
Let’s recap the critical insights: Begin with focused pilots tied to real business goals, and make sure you define success in measurable terms. Treat those early projects as learning opportunities and proof points. With wins in hand, champion the cause to your leadership and across departments – break down silos and bring everyone on board for scaling. Invest in your infrastructure (both tech and data) and your people (skills and change management) so that AI can flourish. Always keep an eye on governance and ethics, because trust is the bedrock of any sustainable AI strategy. And continuously iterate – the roadmap isn’t static; adjust your course as technologies advance and your business needs evolve.
For retailers with 50+ employees, embracing AI at scale can level the playing field with larger competitors or give you a cutting-edge advantage in your niche. The technology is more accessible than ever, with major vendors and platforms offering robust, scalable solutions. The know-how is also more accessible, through playbooks like this, case studies, and expert partners ready to help.
The cost of doing nothing is rising — lost sales, inefficient operations, unhappy customers, and eventually, lost market share. On the flip side, the rewards of a successful AI transformation are substantial: improved revenues, streamlined operations, more personalized customer relationships, and a future-ready organization. By following a structured roadmap from pilot to enterprise adoption, you ensure that your AI investments lead to sustained, compounding benefits, rather than fizzling out after an initial spark.
As a next step, consider conducting an AI readiness assessment for your company: Where do you stand in terms of data readiness, skill sets, and current technology? Identify one or two high-impact pilot ideas (if you haven’t already started) and outline a rough roadmap using the phases described. Engage with a trusted AI consulting partner or your internal innovators to refine this roadmap. Think of it as drawing the blueprint for a long-term journey.
In closing, long-term AI roadmapping is about vision and execution. It’s about being strategic and pragmatic at once – having the audacity to envision an AI-transformed enterprise, and the discipline to get there step by step. The retail landscape is being reshaped by those who commit to this journey. With the insights and strategies outlined here, you have the guidebook in hand. Now it’s time to act. The pilot phase is your launch pad; enterprise AI excellence is your destination. Chart your course, assemble your team, and embark on the AI journey that will define the future of your retail business.
Interested in accelerating your retail AI adoption? HI-GTM specializes in AI consulting and long-term roadmapping. We’ve helped numerous companies go from pilot to powerhouse. Contact HI-GTM for a consultation and let’s build your AI roadmap together, ensuring your retail enterprise thrives in the AI era. Here’s to your success on the road ahead!
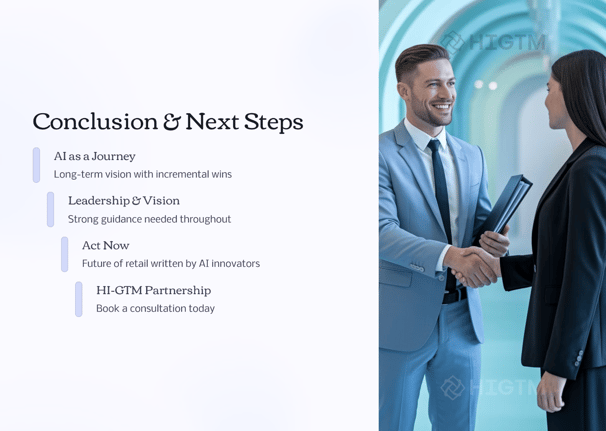
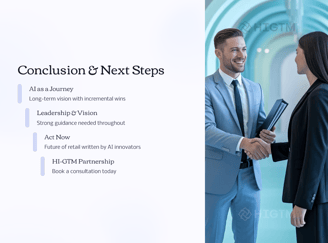
Turn AI into ROI — Win Faster with HIGTM.
Consult with us to discuss how to manage and grow your business operations with AI.
© 2025 HIGTM. All rights reserved.