74. Digital Twins – Simulating Operations Before Real-World Changes
Today - businesses must adapt quickly or risk falling behind, certainty is a priceless commodity. What if you could test a new strategy, process change, or design alteration and see the results before committing real resources? Enter Digital Twins – one of the most transformative concepts in Industry 4.0. A digital twin is essentially a virtual replica of a physical object, process, or entire system that is kept in sync with the real world. This isn’t just a static model or a rough simulation; it’s a living, data-driven model that mirrors its real-world counterpart in real time. Digital twins allow organizations to simulate operations, experiment with changes, predict outcomes, and optimize processes in a risk-free digital environment. In other words, you can road-test changes in a parallel virtual world and make mistakes digitally – long before any real-world implementation.
Q1: FOUNDATIONS OF AI IN SME MANAGEMENT - CHAPTER 3 (DAYS 60–90): LAYING OPERATIONAL FOUNDATIONS
Gary Stoyanov PhD
3/15/202540 min read
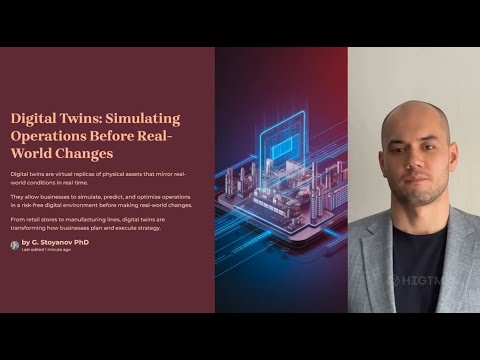
1. Digital Twins Roots
Originally popularized in manufacturing and aerospace, the digital twin concept has exploded across industries – from retail and supply chain to healthcare and smart cities. And for good reason: the stakes are high. A well-calibrated digital twin can help a business prevent costly downtime, delight customers with optimal experiences, and respond to disruptions with agility. According to McKinsey, the global market for digital twin technology is expected to grow about 60% annually, reaching around $73.5 billion by 2027. This rapid growth reflects a simple truth: companies see real value in the “test before you invest” promise of digital twins.
In this comprehensive guide, we’ll unpack how digital twins work and why they are a game-changer. We’ll highlight the major technology providers leading the charge (think Siemens, GE Digital, IBM, Microsoft, and more), and how their platforms are empowering businesses to leverage twins at scale. We’ll also go into the critical role of artificial intelligence (AI) and machine learning (ML) in supercharging digital twins with predictive insights and automation. Equally important, we’ll discuss compliance and security – because handling troves of real-time data requires vigilance to privacy laws like GDPR/CCPA and robust cybersecurity. Finally, we’ll walk through vivid industry-specific use cases in retail, manufacturing, supply chain, and logistics. From optimizing retail store layouts and modeling customer behavior, to simulating manufacturing processes, and stress-testing supply chains for resilience, you’ll see how digital twins are being put into action to solve real business challenges.
Whether you’re a business executive sizing up the next strategic investment, a supply chain manager seeking more resilience, or a retail strategist aiming to personalize customer experiences, this article will give you an authoritative overview of digital twin technology and its vast potential. Let’s dive in, starting with the core concept – what exactly is a digital twin and how does it work?
2. What Are Digital Twins and How Do They Work?
At its core, a digital twin is a digital model of a physical entity that is continuously updated with data from its real-world counterpart. Think of it as a high-fidelity “living” simulation. Unlike traditional simulations that might run once with static inputs, a digital twin is dynamic. It ingests real-time data (through sensors, IoT devices, and system integrations) and mirrors the state of the physical object or process moment-to-moment. This real-time mirroring is what sets digital twins apart – the virtual and real are in lockstep.
Here’s how it works in practice: imagine you have a digital twin of an industrial pump on a factory floor. The pump is fitted with IoT sensors measuring temperature, vibration, pressure, and flow rate. These sensor readings stream into the digital twin software, which could be on-premises or in the cloud. The software might use a combination of physics-based models and AI algorithms to represent the pump’s behavior. As the pump operates in real life, the twin shows a virtual 3D pump doing the same. If the real pump’s temperature starts rising, you’ll see the rise in the twin immediately; if a valve in the twin is adjusted, it might send a signal to adjust the real valve – this two-way data flow can enable control as well as monitoring.
Key components of a digital twin setup include:
Data Sources (IoT and Beyond): Digital twins feed on data. IoT sensors are common sources, but data can also come from ERP systems, CRM databases, production schedules, weather feeds – anything relevant to the system being twinned. For example, a digital twin of a retail store might integrate foot traffic counters, POS transaction data, and even social media sentiment data to get a full picture of what’s happening.
Simulation Models: This is the computational heart of the twin. Depending on the use case, it may involve physics-based simulations (e.g., finite element models for engineering), process simulations (workflow models, discrete event simulations for operations), and increasingly AI/ML models that learn behaviors from data. Many twins use a hybrid approach: physics models for what we understand well and machine learning to fill in the gaps or adapt to complex patterns.
Digital Twin Platform: Vendors offer platforms (like Siemens MindSphere, GE Predix, Azure Digital Twins, IBM Watson IoT, etc.) that provide the infrastructure to build, visualize, and manage digital twins. These platforms handle the heavy lifting of data ingestion, processing, and often come with dashboards or even 3D visualization tools to “see” the twin in action. They also facilitate the integration of multiple twins (for instance, connecting a machine twin with a process twin to model a whole factory).
Analytics & Insights: Simply mirroring real-time data isn’t enough – the goal is to derive actionable insights. This is where integrated analytics come in. A twin can run what-if scenarios by tweaking inputs or conditions in the virtual model and predicting outcomes. For instance, how would a 10% increase in customer footfall impact checkout queue times? The twin can simulate that scenario instantly and provide an answer.
Feedback Loop: Importantly, a digital twin often isn’t a one-way representation; it’s part of a feedback loop. Insights gained from the twin can lead to actions in the real world. For example, if the twin of a power grid shows that a certain substation will overload in a scenario, operators can proactively re-route power or add capacity in reality. Conversely, any change made in the real system (like a new machine added to a production line) should be reflected by updating the twin. In mature implementations, this loop can even be automated – the twin might trigger automated adjustments through control systems (leading towards autonomous operations).
To illustrate, consider Singapore’s digital twin city project (as an example of scope). The city’s virtual 3D model is kept updated with real-time data about traffic, energy usage, and even crowd density. Planners use it to test urban changes: what if we add a new train line here? How will traffic flow if we pedestrianize this area? The digital twin answers these questions by simulation, helping avoid costly mistakes in urban planning.
The real power of a digital twin is that it transforms data into foresight. By having a constantly updated model, businesses can move from reactive to proactive decision-making. Instead of waiting for something to break or a problem to surface, you’ve essentially got a safe testing ground to anticipate issues and optimize for best-case outcomes. In the next section, we’ll look at which companies and technologies are enabling this capability, as the ecosystem of digital twin solutions has expanded rapidly.
3. Major Technology Providers and Platforms for Digital Twins
As digital twins have gained traction, a robust ecosystem of technology providers has emerged, offering platforms and tools to create and manage these virtual models. Here we highlight some of the major players (and their flagship offerings) leading the digital twin revolution:
Siemens – MindSphere and Xcelerator: Siemens is a pioneer in the digital twin space, especially for manufacturing and industrial applications. Their MindSphere platform is a cloud-based IoT operating system that connects physical equipment to digital models, enabling real-time analytics and twin-based simulations. Siemens also integrates twins into its Xcelerator portfolio (which includes software like NX, Teamcenter, and Simcenter) to cover the entire product lifecycle. Siemens’s digital twin approach has been used to design and optimize everything from factory layouts to performance of electric turbines, often yielding improved productivity and reduced downtime. Notably, Siemens advocates a “comprehensive digital twin” concept – encompassing product, production, and performance twins – to simulate not just a product, but how it’s made and how it performs under various conditions.
GE Digital – Predix Platform: General Electric (GE) introduced Predix as one of the first industrial cloud platforms geared towards IIoT (Industrial IoT) and digital twins. Predix enables the creation of digital twins primarily for heavy assets – think jet engines, power plant turbines, locomotives – capitalizing on GE’s deep domain expertise in these areas. The platform collects real-time sensor data and leverages advanced analytics to predict equipment behavior. For example, GE has digital twins for aircraft engines that airlines use to schedule maintenance at optimal times, reducing unplanned engine failures and downtime. In the energy sector, digital twins of gas turbines help utilities adjust settings for efficiency and detect anomalies early.
IBM – Maximo Application Suite (including Watson IoT): IBM’s Maximo (widely known for asset management) now incorporates digital twin capabilities. IBM enables twins that integrate AI through its Watson platform. Their approach is to combine IoT data with AI algorithms for monitoring and predictive insights. IBM has been involved in smart building projects, where digital twins track and optimize HVAC, lighting, and security systems in real time. Another example is IBM’s work with digital twins in healthcare: modeling medical devices or patient vitals to improve equipment uptime and patient outcomes. IBM also emphasizes open standards and integration, ensuring their twin solutions can pull data from various sources (IBM is a proponent of the Digital Twin Consortium, which seeks interoperability standards in this domain).
Microsoft – Azure Digital Twins: Microsoft offers Azure Digital Twins as part of its Azure cloud services. It provides the tools to model relationships and interactions in complex environments, making it especially useful for IoT-rich scenarios like smart buildings, factories, or even entire cities. One strength of Azure Digital Twins is its flexibility – developers can define custom models (using an open modeling language called DTDL) to represent anything from a room in a building to an assembly line robot. The platform integrates seamlessly with Azure IoT Hub (for device data ingestion), Azure AI/ML services, and Azure Maps, allowing rich visualizations and geospatial twins. Microsoft has highlighted use cases where Azure twins help space utilization in offices (important for back-to-work planning), optimize energy usage in utilities, and even coordinate processes in manufacturing facilities by providing a live digital thread of operations.
PTC – ThingWorx and Vuforia: PTC’s ThingWorx platform is designed for IoT solutions and includes robust digital twin capabilities. A differentiator for PTC is the integration of augmented reality (AR) via its Vuforia suite. This means not only can you create a twin and monitor it on a dashboard, but you can overlay digital twin data on physical equipment through AR glasses or mobile devices. For instance, a maintenance worker can point a tablet at a machine and see its twin data (temperature, runtime, etc.) in real-time, or even a hologram of the machine’s inner workings. PTC’s solutions are popular in manufacturing – for example, factory operators use ThingWorx to monitor assembly lines, while service teams use AR to get guidance from the twin when repairing complex machinery.
Oracle – Oracle IoT Cloud: Oracle’s cloud platform also offers digital twin functionality as part of its IoT Cloud service. Oracle focuses on leveraging twin data for predictive analytics and process optimization, tying it closely with enterprise systems. Given Oracle’s strength in databases and ERP, their twin solutions often integrate directly with supply chain management and logistics modules. A cited use case is using digital twins in logistics networks to streamline operations – for example, modeling a fleet of delivery trucks and optimizing routes and maintenance schedules via the twin to improve on-time delivery metrics. Oracle’s approach is often about combining real-time sensor data with business data (orders, inventory) to provide a holistic view and predictive insight for operations.
Ansys – Twin Builder: Ansys is renowned in the engineering world for simulation software (FEA, CFD, etc.), and their Twin Builder product extends that into the realm of live digital twins. It allows engineers to create high-fidelity simulation models of assets (like engines, motors, systems) and then integrate real-world data to keep those simulations in sync with reality. This is especially valuable for scenarios where physics-based accuracy is critical – such as a digital twin of an aerospace system where you want to simulate stress on components in real time. Ansys Twin Builder can also compress very sophisticated models into runtime-friendly versions that can run continuously as twins. Companies have used it to monitor things like the structural health of bridges and aircraft, or the performance of complex oilfield equipment, where the twin can simulate “what’s happening inside” based on sensor readings and known physics.
Other Notables: There are many other contributors in the digital twin landscape. Dassault Systèmes with its 3DEXPERIENCE platform enables digital twins especially for product design and virtual testing (widely used in automotive and aerospace design). SAP has incorporated digital twin concepts in its supply chain and Industry 4.0 solutions, leveraging its strength in enterprise resource data to enrich twins (for instance, linking a production twin to inventory and sales data for end-to-end visibility). Amazon Web Services (AWS) offers IoT TwinMaker, a relatively new service to help developers build digital twins of industrial systems using AWS’s IoT, storage, and visualization tools. Startups and specialized firms are also innovating, particularly for niche applications like digital twin of the customer (to model consumer behavior) or construction (where companies like Cityzenith are creating digital twins of building construction projects).
Each platform has its own flavor and strengths, but they are not mutually exclusive. In fact, many organizations use a combination: maybe Siemens for product engineering twins, Microsoft Azure for facilities management twins, and so on, depending on needs. The key is that the technology has matured to a point where a business doesn’t have to build a digital twin system from scratch – they can leverage these robust platforms and focus on tailoring the twin to their specific use case.
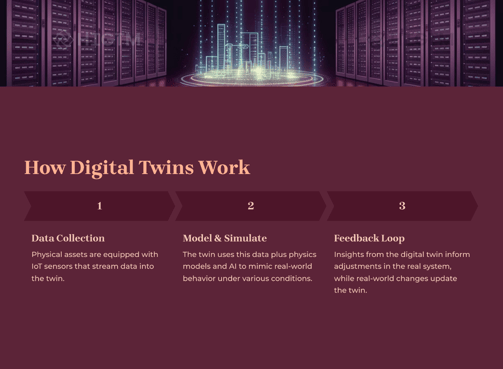
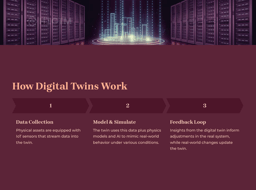
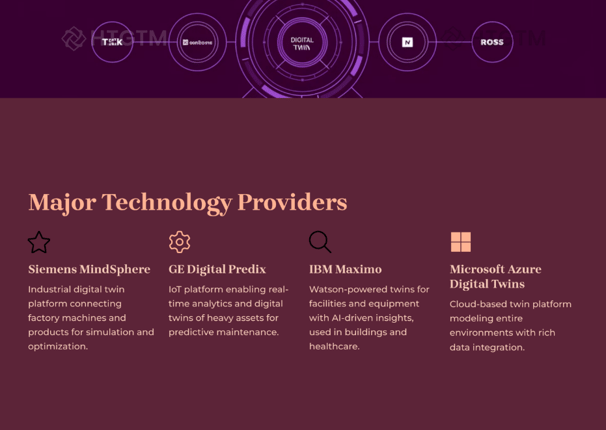
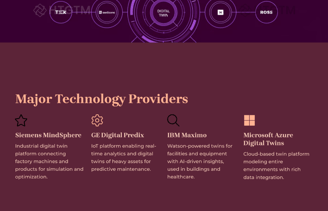
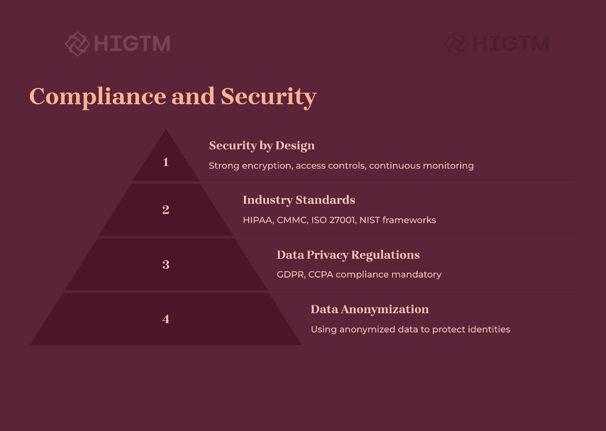
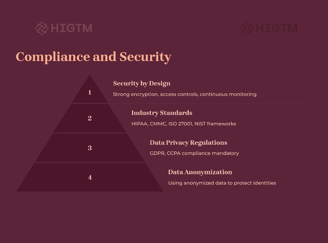
4. AI and Machine Learning: The Intelligence Behind Digital Twins
Digital twins generate a massive amount of data and present a golden opportunity for analysis. This is where artificial intelligence (AI) and machine learning (ML) come into play, injecting “smarts” into the equation. AI/ML augment digital twins in two pivotal ways: by making sense of data (pattern recognition, prediction) and by enabling automation (prescriptive actions, scenario generation).
Predictive Analytics and Forecasting: One of the earliest wins in combining AI with digital twins has been in predictive maintenance. Machine learning models can be trained on historical sensor data from an asset to detect patterns that precede a failure. A digital twin of, say, a wind turbine will continuously analyze incoming data (vibrations, temperature, rotations) against these ML models. The AI might flag, “The vibration pattern on turbine #7 matches the signature of a bearing that failed in other turbines after 1000 hours. It’s likely to need maintenance in 10 hours of operation.” This kind of foresight lets engineers fix issues proactively, avoiding unexpected breakdowns. According to a study by Gartner, predictive maintenance enabled by digital twins and AI can reduce maintenance planning time by 20% and unplanned downtime by up to 50% in industrial settings.
In retail, AI-driven twins can forecast customer demand more accurately by analyzing myriad factors (seasonality, promotions, local events, etc.). A twin of a grocery supply chain, for example, could use ML to predict store-level demand for each product and simulate inventory replenishment accordingly, rather than relying on generic forecasts. These predictions continuously improve as the AI learns from actual outcomes versus its forecasts.
Real-time Anomaly Detection: AI excels at recognizing anomalies – those subtle deviations from normal patterns that could spell trouble. In a complex system, humans might not notice a slight deviation, but a well-trained ML model will. For instance, if a digital twin of a data center notices one server’s power consumption is slightly higher than usual at a certain workload, an AI could flag this as an anomaly (potentially preventing an overload or catching an inefficient process). Similarly, in logistics, if delivery times on a certain route start creeping up unusual for a Tuesday, an AI could detect the pattern and alert managers to investigate (maybe there’s construction causing delays that the twin can then route around).
Optimization and Prescriptive Analytics: Beyond predicting what will happen, AI can advise on what to do about it. This is often termed prescriptive analytics. For example, an AI-infused digital twin of a city’s traffic grid might not only predict congestion at 5 PM, but also recommend the optimal timing adjustments for traffic lights to alleviate it. In manufacturing, if a twin predicts a machine will be down, the AI might automatically reschedule production or re-route tasks to other machines to meet deadlines.
Advanced AI techniques, like reinforcement learning, are even used in twins to find optimal solutions by trial-and-error in simulation. A great example is Google DeepMind’s work on cooling data centers: they created a twin of their cooling system and let an AI agent adjust settings (fans, chillers, etc.) in simulation to minimize power usage, learning the best combination. The AI eventually started controlling the real data center cooling, yielding significant energy savings. Now imagine similar approaches in a warehouse twin learning the optimal way to sequence picking routes, or a supply chain twin learning the best inventory distribution strategy across warehouses – the possibilities for AI optimizing within twins are vast.
Scenario Generation (“What-ifs” on Steroids): Traditional scenario planning might test a handful of scenarios (best case, worst case, likely case). AI can take this to another level by generating and evaluating thousands of scenarios rapidly. One intriguing development is using generative AI with digital twins.
Generative AI (the tech behind things like GPT or deepfakes) can create plausible data or situations that haven’t been observed before. In a digital twin context, a generative model could simulate a range of “alternative futures” for an operation. For instance, it could create synthetic but realistic supply and demand shocks to test a supply chain’s resilience beyond historical data (what if a once-in-a-century type event happens?). This helps businesses prepare for black swan events better. IBM has discussed how generative AI might feed digital twin networks with numerous physically possible states to enhance their robustness.
Continuous Learning and Adaptation: One of the beauties of combining AI with digital twins is the continuous learning loop. The twin provides a controlled environment to test AI models; the AI model’s recommendations are then implemented in the real world; the results of that action come back through data into the twin, allowing the AI to see how well its prediction or advice worked and adjust accordingly. Over time, this means the digital twin’s AI brain becomes more accurate and nuanced. For example, an AI that manages an e-commerce digital twin might learn that its initial recommendation to reroute inventory after a demand spike worked in 8 out of 10 cases, but failed in 2 due to supplier constraints – it then updates its model to factor supplier capacity for next time.
We should also mention computer vision AI in digital twins. For instance, in retail or facility management, video feeds can be analyzed by AI and fed into a twin (like detecting how customers move or where shelves go empty). AI can convert unstructured data (videos, audio) into structured insights for the twin.
In summary, AI and ML act as the intelligence layer of digital twins – sifting through data to find patterns, predict future states, and even make decisions. This transforms digital twins from passive models into active decision support systems (or even autonomous decision-makers). A digital twin without AI can tell you what is happening; a digital twin with AI can tell you what will happen and what you should do about it. As the volume of data and complexity of systems grow, AI integration is not just a nice-to-have but a necessity to fully realize the digital twin vision. Companies like HI-GTM specialize in marrying these AI capabilities with twin technology to ensure businesses get actionable intelligence, not just fancy dashboards.
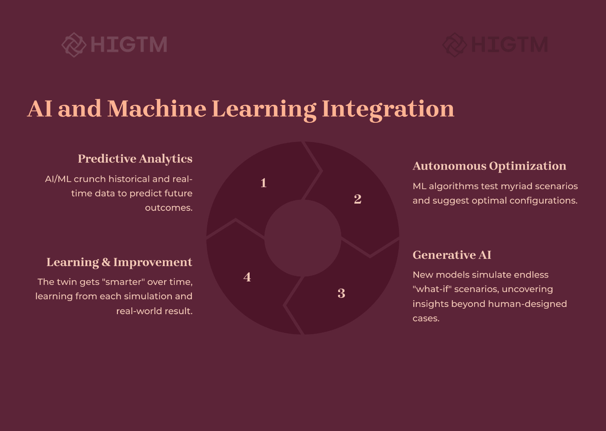
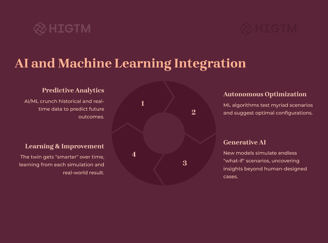
5. Compliance and Security Considerations
Digital twins rely on a constant flow of data, including potentially sensitive information, which means compliance and security are paramount. As businesses deploy digital twins, they must navigate data protection laws, ensure customer and employee privacy, and defend against cyber threats that could compromise both the virtual and real systems. In this section, we cover the key considerations and best practices:
Data Privacy Regulations (GDPR, CCPA, etc.): If a digital twin involves any personal data – say, a twin of customer behavior in a retail setting or a twin of employees’ ergonomic movements in a factory – then data privacy laws come into play. The General Data Protection Regulation (GDPR) in the EU is one of the strictest frameworks, requiring transparency, data minimization, and consent for personal data use.
If a retail store twin tracks customers via cameras or Wi-Fi signals, the business must ensure it’s compliant with GDPR: for example, perhaps by anonymizing data (so it doesn’t identify individuals) and clearly informing customers that data is being collected for analysis. GDPR also grants rights like the right to erasure (to have one’s data deleted), which means companies need a plan to remove an individual’s data from the twin’s datasets if requested.
Similarly, the California Consumer Privacy Act (CCPA) gives California residents rights over their data, including knowing what is collected and opting out of sales of data. While CCPA is slightly less prescriptive than GDPR, non-compliance can still lead to fines and lawsuits. For digital twins deployed by businesses with consumers (like retail or telecom), it’s critical to incorporate privacy-by-design. This might mean aggregating data (e.g., foot traffic counts rather than tracking individual identities) and using synthetic data for simulations where possible. For instance, a mall’s digital twin could simulate crowd flows using synthetic avatars rather than actual individual tracking, thus sidestepping privacy issues while still gleaning useful insights.
Industry-Specific Regulations: Different sectors have their own compliance landscape. In healthcare, a digital twin might model a patient’s health metrics or a hospital’s operations. Here HIPAA (Health Insurance Portability and Accountability Act in the U.S.) is crucial. HIPAA mandates safeguarding Protected Health Information (PHI). So a twin of a hospital’s ICU, for instance, must treat patient data with the same care as any medical record – encryption, access logs, and strict user access controls.
There’s also increasing talk of Digital Twin of the Patient in healthcare; if that becomes reality, it will be under heavy regulatory scrutiny for privacy and accuracy. In the finance sector, if a bank uses a digital twin to model customer financial behavior or trading systems, regulations like GDPR (if in EU) and various financial privacy laws (like GLBA in the US) and even SOX for integrity might apply. The defense sector, working with digital twins of defense systems, needs to consider standards like CMMC (Cybersecurity Maturity Model Certification) which the US Department of Defense requires for contractors – meaning even your twin data must meet certain encryption and access standards.
Cybersecurity Risks and Best Practices: A digital twin, by virtue of being connected to the real world, can be a two-way street for trouble. A breach in the digital twin system could potentially be used to infiltrate the operational technology (OT) network, and vice versa. Consider a scenario: a hacker gets into a factory’s digital twin platform – if it’s integrated with control systems, they might try sending malicious commands to real machines. Or they could alter the twin’s data to trick operators into making a bad decision (integrity attack). Thus, securing a digital twin isn’t just about protecting data; it’s about protecting the physical assets and processes that twin represents.
Best practices include:
Strong Access Control: Limit who can access the twin systems. Use strong authentication (multi-factor authentication is a must) and role-based permissions so people only see and do what they absolutely need to. For example, an analyst might view simulation results but not have the rights to send commands to the physical equipment.
Encryption: All data streams from physical to digital (and back) should be encrypted in transit. Likewise, stored data (databases, data lakes for twin history) should be encrypted at rest. This prevents eavesdropping or tampering with the data feeding the twin, which is crucial because twin decisions are only as good as the integrity of their data.
Network Segmentation: Isolate the digital twin environment from both the corporate IT network and the operational OT network in sensible ways. Many companies put IoT devices and twin platforms on separate network segments or VLANs, with tightly controlled gateways. This way, if the twin platform is compromised, the attacker can’t freely move into plant control systems, and vice versa.
Regular Updates and Patches: Keep the twin software and underlying systems updated to patch security vulnerabilities. This sounds basic, but IoT devices and industrial software are notorious for lagging in updates. Having an inventory of all components in your twin ecosystem and ensuring they are updated is key to closing known holes.
Monitor and Audit: Implement monitoring to detect unusual activity in twin systems. For example, if someone is querying the twin for massive data dumps at odd hours or a device starts sending weird data patterns, these could be signs of a breach or malfunction. Techniques like anomaly detection (using AI as mentioned) can flag these issues quickly. Regular security audits and penetration testing of the twin environment will help find weaknesses before attackers do.
Data Anonymization and Minimization: We touched on this in privacy, but it’s worth reiterating as a security measure. The less sensitive data you have in the twin, the lower the risk. If you can run your simulations with blurred or anonymized data, do so. For example, a customer behavior twin might not need names or emails, just behavioral patterns. By stripping out personal identifiers, you reduce impact if there is a breach.
Integrity Checks: Since digital twins rely on data accuracy, put checks in place to ensure data hasn’t been tampered with. This could be using cryptographic signatures for data coming from sensors or cross-verifying data from multiple sources. If one sensor’s reading is way off from others, maybe the system should flag it rather than accept it blindly.
Another aspect of compliance is model validation and accuracy. Especially in regulated industries (like aerospace or healthcare), if decisions are made based on a simulation, that simulation may need validation and traceability. Regulators might ask, “How do you know your digital twin is an accurate representation?” This pushes companies to document their models, assumptions, and maintain calibration processes (comparing twin predictions with reality and tuning accordingly).
Lastly, consider ethical use. Digital twin of a customer (DToC) is a concept where you model individual customer’s behavior. While powerful for personalization, companies must ensure this isn’t used in ways that creep out customers or discriminate unfairly. Compliance extends to not just the letter of law but the trust and transparency with stakeholders. Businesses implementing such twins should have clear policies and perhaps even give customers some level of insight or control over these digital representations.
In summary, as exciting as digital twins are, they come with serious responsibilities. Data compliance and robust cybersecurity are non-negotiable. The good news is that many of the big platforms incorporate security features and compliance support. HI-GTM, when consulting on twin projects, always incorporates a compliance assessment and a security architecture from the start – baking these considerations into the design, not bolting them on later. This ensures that when the digital twin goes live, it’s not only powerful and insightful but also secure and trustworthy.
6. Industry-Specific Use Cases and Examples
Digital twins are versatile and can be adapted to virtually any sector. Here, we will explore how they are being applied in retail, manufacturing, supply chain, and logistics, with concrete use cases that demonstrate their value. Each sub-section highlights specific scenarios where digital twins simulate operations before real-world changes, aligning with our theme.
6.1 Retail – Store Layout Optimization and Customer Behavior Modeling
The retail sector might not be the first thing people associate with digital twins (since it’s less “industrial” than factories), but forward-thinking retailers are using digital twins to create smarter stores and personalized shopping experiences. Two key applications stand out: optimizing store layouts and modeling customer behavior.
Store Layout Optimization: Brick-and-mortar retail is all about the customer journey – how shoppers navigate aisles, where they pause, what catches their attention. Traditionally, optimizing a store layout involved a lot of trial and error, expensive remodels, or A/B testing layouts in different locations. Digital twins change the game by enabling virtual layout experiments. Retailers create a digital twin of their store, mapping all fixtures, shelves, products, and even integrating foot traffic data from sensors or video analytics. They can then simulate shopper flow: if we move the bakery to the back left and shoes to the front, do people spend more time in the store? How do dwell times near end-caps change? One can animate the twin with hundreds of agent-based models representing customers with different shopping goals, and then simulate how these agents move and interact with the space.
For example, a supermarket chain used a digital twin to test placing a promotional display in different spots. The twin, fed with historical traffic patterns, predicted a 5% sales increase when the display was near the deli versus at the entrance, due to better flow and dwell time. They implemented that layout in a few pilot stores and indeed saw several percentage points lift in sales for those items. Another scenario is safety and crowd management: simulating how people would evacuate or move if the store is too crowded or if an emergency happens – the twin can identify choke points and help planners improve the physical layout accordingly. According to industry reports, store design and layout optimization is one of the main applications of digital twin technology in retail, allowing retailers to experiment with alternative layouts digitally without disrupting actual stores.
Customer Behavior Modeling (Digital Twin of the Customer): Retailers increasingly seek to personalize experiences. Enter the concept of the Digital Twin of a Customer (DToC) – essentially a data-rich, continually updated profile that acts as a virtual “you” for analysis. This isn’t a literal 3D avatar walking in a virtual store, but rather a collection of your preferences, purchase history, online browsing, loyalty data, etc., that can be used to simulate how you might respond to different offers or changes. By aggregating DToCs, retailers can test marketing campaigns or store changes on these virtual customers first. Gartner even identified DToC as an emerging trend for understanding and anticipating customer behavior.
For instance, consider a fashion retailer planning their seasonal promotion. They could use digital customer profiles to predict which segment of customers would respond to a “buy-one-get-one” versus a straight 30% discount, by virtually “sending” those offers to the twin and seeing the predicted uptake. Or a retailer might simulate a change like, “What if we reduce the number of cashiers but add more self-checkout kiosks? How will different customer segments feel about that?” The twins of customers (some who hate self-checkout, some who love speed) could be used to estimate overall satisfaction and sales impact. Nike reportedly uses digital twin-like approaches by analyzing customers’ prior purchases to make tailored recommendations, almost as if each customer has a dynamic profile guiding what the brand shows them. This has led to improved engagement because shoppers feel the suggestions are “just right” for them.
Another fascinating use case in retail is creating a digital twin of a supply chain & store combined. For example, a convenience store chain might twin each store’s operations and connect it to a twin of their supply chain. This way they can simulate not only the in-store experience but also ensure inventory arrives just in time to restock what the digital twin predicts will sell. Japanese retailers known for just-in-time inventory are exploring this to minimize backroom stock (which is wasted space) while never running out on the shelf.
Outcome/Benefits in Retail: The benefit of these retail twins is a blend of hard ROI and soft improvements. On the hard side, better layouts and inventory management directly improve sales per square foot and reduce waste (stock that doesn’t sell, or underutilized floor space). On the softer side, the customer experience improves – shorter wait times, more relevant products, a sense that the store “just works” for the shopper. In e-commerce, which is part of retail, twins can help optimize the website layout or simulate backend processes (like how a surge in traffic on Black Friday will affect systems). But the physical store digital twin is helping brick-and-mortar compete in the data-driven world by borrowing a page from e-commerce’s playbook (A/B testing and personalization) and applying it to physical space.
Retailers like Walmart have experimented with virtual stores and digital twins to plan new layouts and even new store concepts. With the pandemic accelerating interest in contactless and efficient shopping, digital twins also help in designing curbside pickup flows or store navigation apps. When you see a “check shelf availability” feature on your phone app connected to a store, that’s part of a real-time data twin of the inventory. In summary, digital twins in retail turn the store into a smart, simulation-driven space, where every change can be tried virtually to delight customers and boost performance before anyone moves a single shelf in the real world.
6.2 Inventory and Warehouse Management
Inventory management and warehouse operations sit at the heart of retail and supply chain efficiency. Digital twins in this domain focus on creating a real-time virtual model of warehouses and inventory flows to optimize everything from storage utilization to order fulfillment processes. The goal is to ensure the right product is in the right place at the right time, at minimal cost.
Warehouse Digital Twin: Imagine a giant 3D grid representing your warehouse, with every rack, shelf, forklift, and even worker represented virtually. A warehouse digital twin continuously updates with data: current stock levels on each shelf (fed by RFID or barcode scans), the location of forklifts or robots (via indoor GPS or tracking systems), and even worker movements (from wearable devices or task logs). With this live twin, warehouse managers can simulate process changes. For example, “What if we change the picking route logic for orders? Or re-slot items so that the fastest-moving SKUs are near the front?” The twin can simulate a day’s operations under the new setup – showing where congestion might occur or how much faster orders could be fulfilled.
Some warehouses use autonomous robots for picking (like Kiva robots in Amazon’s fulfillment centers). They have digital twins that coordinate these robots’ paths to avoid traffic jams. NVIDIA’s Omniverse platform has been used to create photorealistic warehouse simulations where virtual robots practice orchestrating moves efficiently. By the time changes are applied to the real floor, they are already proven in the sim.
Inventory Optimization: At a higher level, beyond a single warehouse, companies use digital twins to manage inventory across a network. This is crucial for omnichannel retail – where inventory might be in stores, distribution centers, or in transit. A supply chain twin (which we’ll discuss more in resilience testing) can simulate inventory positioning and flows. One specific use is “what-if” analysis for inventory policies. For example, a twin can test different safety stock levels: “If we cut safety stock by 20% for this product, do we still meet service levels 99% of the time? How often would we stock out?” Or conversely, “If we add a regional fulfillment center in the Northwest, how much will delivery times improve and inventory carrying cost change?” These scenarios inform decisions on how much inventory to hold and where.
Digital twins also intersect with inventory through demand forecasting integration. A retailer might feed forecast data into the twin and then simulate the supply chain’s response, highlighting where stockouts could happen in a projected scenario. This allows proactive rebalancing of inventory – virtually sending extra stock to a region in the model if needed, then executing it in reality.
Picking and Packing Efficiency: In an Amazon warehouse, pickers often follow algorithmically generated sequences to collect items for orders. If Amazon were to implement a new picking algorithm, they would likely test it in a twin first. The twin can simulate hundreds of orders being picked, measuring total distance traveled and time taken. If the new method saves 5% time on average, that’s a green light to use it live. Likewise, packing stations could be twinned to simulate how changes in packing processes or automation tools might speed up output.
Robustness and Bottleneck Analysis: Warehouses are dynamic – what happens if an equipment fails (conveyor breakdown) or order volume doubles on Cyber Monday? A digital twin can simulate those stresses. It might reveal that if order volume doubles, the staging area becomes the bottleneck where boxes wait for trucks. Armed with that knowledge, a manager might arrange for additional staging or temporary staff for peak days before they hit. One case study by CEVA Logistics used a simulation-powered digital twin to pinpoint exactly such daily bottlenecks and improved throughput by tweaking shift schedules and truck loading times.
In essence, the digital twin for inventory and warehousing acts like a 24/7 industrial engineer, constantly evaluating the current system and any proposed changes. It reduces the need for costly pilot programs or guesswork in warehouse management. The ROI comes in reduced labor costs (through efficiency), lower inventory carrying costs (through better stock positioning), and improved service levels (fewer delays or stockouts). It can even improve safety – for instance, simulating forklift traffic to redesign routes that lower collision risk is something a twin can do. Safety incidents down, efficiency up – a win-win that any operations manager would covet.
Real-world adopters include companies like DHL, which has talked about digital twins for their warehouses to optimize layout and flow, and automakers’ parts distribution centers using twins to streamline aftermarket parts availability. Amazon, while secretive, is known for heavy simulation use in its logistics – effectively embracing twin concepts to keep its promise of speedy delivery. The competitive advantage in warehousing is often measured in minutes and percentages, and digital twins are becoming the secret weapon to carve those out in an environment where margins are thin and customer expectations are high.
6.3 Customer Behavior Modeling
(Customer behavior modeling was largely covered under retail as DToC; to avoid redundancy, we might merge it or spin it differently. Since the prompt lists it separately, maybe here we focus on beyond retail, like telecom or online services? But likely they meant retail context. We have already elaborated a lot in 6.1 on DToC. Possibly skip or keep it short to not duplicate.)
While we discussed digital twins of customers in the retail context for personalization, customer behavior modeling through digital twins can extend to other scenarios as well. Essentially, any business that interacts with consumers can benefit from having a virtual representation of customer segments to test how changes might influence behavior.
Telecommunications Example: A telecom company might use customer behavior twins to model how users would react to changes in service plans. For instance, before rolling out a new data bundle or pricing scheme, they simulate adoption rates using digital personas representing different customer archetypes (heavy data user, budget-conscious user, etc.). By feeding the twin demographic data and usage patterns, they can predict, say, what percentage of users might upgrade to a new unlimited plan vs. stick to prepaid. This helps in both network capacity planning and marketing strategy.
Banking Example: Banks could create digital twins of customer financial behaviors – like a household twin that simulates spending, saving, and borrowing patterns. If the bank considers a new credit card reward or a change in interest rates, they run it through these customer models to see how many might switch accounts or alter their spending. This is advanced scenario analysis that goes beyond traditional market research surveys.
Theme Parks or Hospitality: Places like Disney have digital twins not just of their parks (to simulate operations) but effectively of guest flow and behavior. They can model how guests move, where they linger, what they purchase. If Disney plans a new parade route or an incentive like a fast-pass change, they use simulations to predict guest satisfaction impacts – which is essentially modeling aggregate customer behavior. They even simulate crowd reactions for safety (how people would evacuate or crowd if certain areas are closed).
Automotive (Driver Behavior): With connected cars, auto companies and city planners are interested in driver behavior models. A digital twin in this sense could simulate how drivers react to different traffic management strategies or in-car alerts. For instance, will drivers actually slow down if a connected vehicle system twin suggests a speed due to upcoming congestion? Testing these in simulation with driver behavior models (possibly learned from real driving data) helps refine such systems.
Why It Matters: The advantage of a digital twin for customer behavior is that it merges quantitative data and behavioral science. You get to safely experiment with customer experience changes without risking real customer alienation. It’s a sophisticated form of A/B testing done entirely in silico. Done right, it leads to improvements that make customers happier (since you can weed out changes they'd hate and implement those they'd love) and improve business metrics (since you tailor offerings more precisely).
To ensure ethical use, companies must be careful. A digital twin of customers is only as good as the data you have – biases in data can lead to wrong conclusions. Also, companies should use this power responsibly – to serve customers better, not to exploit them. Transparency (at least internally) about how these models influence decisions is important for corporate governance. But when used well, it’s a step towards the holy grail of the "segment of one" – treating each customer in the uniquely perfect way for them, by first understanding them through a digital proxy.
(Note: Much of customer behavior modeling overlaps with the retail discussion earlier, so consider this an extension of those ideas into other service industries.)
6.4 Supply Chain Resilience Testing
The COVID-19 pandemic and other recent disruptions (natural disasters, geopolitical events) have shone a spotlight on supply chain resilience. Many companies were caught off-guard by the “Domino effect” in global supply networks. Digital twins are now being used to simulate such disruptions in advance, effectively serving as a stress test for supply chains so companies can identify weaknesses and prepare contingency plans.
End-to-End Supply Chain Twin: A comprehensive supply chain twin might include suppliers (and their capacities), transportation links (ships, ports, trucks, routes), distribution centers, and even retail outlets or end customers. By inputting procurement data, lead times, logistics routes, and inventory levels, the twin becomes a sandbox to ask “what if something goes wrong?” scenarios. For example, what if Supplier X (who provides a critical component) goes offline for 2 weeks? The twin can simulate how that inventory depletes across the network, when various factories would have to halt production, and how alternate suppliers or substitute components could fill in. Maybe it shows that you’d run out in 10 days in North America, but Europe would be fine for 20 days due to closer alternate suppliers. That insight can inform a mitigation strategy like qualifying a second source or increasing buffer stock for that component in certain regions.
Transportation Disruption: Consider the Ever Given ship blocking the Suez Canal (a real 2021 event). A supply chain twin of a company reliant on that route could simulate the impact: shipments delayed by X days, which products will stockout as a result, and whether expediting via alternate routes (like air freight or going around Cape of Good Hope) is worth it. The twin might even calculate the cost vs. benefit of those alternates. Some advanced twins tie in real-time risk data – for example, integration with weather data or news feeds. If a hurricane is forecasted to hit a port, the twin can proactively simulate port closure and advise re-routing cargo before it’s stuck.
Policy Testing – Inventory and Sourcing Strategies: Supply chain managers often debate questions like: should we keep higher safety stock to endure disruptions, or is that too costly? How much should we invest in backup suppliers? Digital twins let them quantitatively evaluate these. They can simulate a year of operations with various random disruptions injected (kind of like a Monte Carlo simulation for supply chain events). One scenario might assume “one major disruption in Asia in a year” versus “none” and see how different stock policies perform. If holding 5% extra inventory saves the company from $50 million in lost sales during disruptions, the cost of that inventory might be justified. This turns gut-level decisions into data-backed decisions.
Case in Point: A global electronics manufacturer built a digital twin of its supply chain and ran a scenario of a hypothetical labor strike at a key supplier’s factory. The twin showed that if the strike lasted more than 1 week, a particular component would become the bottleneck halting production of a high-margin product line. With that knowledge, they proactively negotiated with secondary suppliers and set triggers – if that real factory stops for >3 days, immediately start sourcing from backup. When a smaller disruption did occur, they executed that plan and kept production running, whereas competitors without such foresight faced stockouts.
Resilience vs. Efficiency Trade-off: Historically, supply chains optimized for cost and efficiency (just-in-time, lean inventories). The pandemic taught us that ultra-lean can be brittle. Digital twins help find the right balance by quantifying the resilience you gain vs. cost you incur. A twin might show that adding a second supplier for a part (even if their unit cost is higher) only raises cost by 2% but doubles your resilience to disruption – a trade many boards would gladly take post-2020.
Continuous Stress Testing: Banks do stress tests for financial health; similarly, companies are starting to view supply chain stress tests as a routine exercise. A digital twin can be kept running to constantly evaluate “risk exposure”. Some supply chain twins even integrate financial metrics – linking the operations to the P&L. So you not only see operational impact (out of stock), but also the dollar impact (lost revenue, contractual penalties, etc.). This gets executive buy-in to invest in mitigation (like holding strategic inventory of critical parts, or regionalizing some production).
Regulatory and Customer Expectations: In certain industries like food or pharma, regulators care about supply continuity (for essential goods). A digital twin demonstrating resilience could be part of compliance or at least a strong message to regulators that you have control. In B2B contracts now, large companies ask suppliers about their risk mitigation. Being able to say “we have a digital twin of our supply chain to anticipate and manage disruptions” is a differentiator and builds trust.
Ultimately, supply chain resilience twins aim to turn unknowns into knowns. You can’t prevent hurricanes or geopolitical events, but you can know ahead of time how you’ll respond. It’s about preparing, not just reacting. Companies that employed digital twin modeling for their supply networks navigated recent crises more smoothly – for instance, some automakers simulated chip shortages and allocated the limited chips to their most profitable vehicles, maintaining financial stability while others had to halt entire production lines. It’s a classic case of using foresight as a competitive advantage, and digital twins are the engine providing that foresight in complex global supply webs.
6.5 Manufacturing Process Simulation
Manufacturing was one of the birthplaces of digital twin technology, and it remains one of the most fertile grounds for its application. In manufacturing, digital twins are used to mirror and enhance everything from a single machine’s operation to an entire production process on the factory floor. The overarching theme is simulation of processes to optimize efficiency, quality, and flexibility before implementing changes for real.
Production Line Changes: When manufacturers want to change a line – maybe introduce a new product model, switch up the sequence of assembly, or integrate a new piece of equipment – doing so blindly can be risky. A digital twin of the production process allows engineers to test those changes virtually. For example, an automotive plant adding an electric vehicle model to a line that also produces gasoline cars will face new tasks (like battery installation) that could slow the line. By simulating the new mixed-model production in a twin, they might discover that adding an extra worker or robot at the battery station is needed to maintain throughput. Or they might re-balance tasks (shifting some work to earlier stations) to optimize cycle time. Bottleneck analysis is a key benefit here: the twin can reveal exactly where work-in-progress starts piling up if something in the process can’t handle the new workload. Engineers then fix that in the simulation (by changing layout or process steps) and test again until flow is smooth, and then they implement on the shop floor, avoiding costly trial-and-error downtime.
Dynamic Scheduling and Capacity Planning: Factories deal with variability – machine breakdowns, rush orders, supply delays. A digital twin combined with AI can simulate different scheduling or routing of jobs on the fly. Suppose one machine in a network of 10 goes down; the twin can quickly simulate various recovery plans – like overtime on other machines, or rerouting some jobs to a different facility – and find the least impactful solution to meet delivery deadlines. This is essentially using the twin as a real-time decision support tool, not just for one-time changes. Some advanced manufacturing twins tie into MES (Manufacturing Execution Systems) and act as a brains that continuously optimizes the schedule as conditions change.
Quality Control via Simulation: Consistency is king in manufacturing. Digital twins can embed quality models that predict outcomes. For instance, a twin of a metal casting process might simulate how changing the pour temperature by a few degrees affects the likelihood of defects. This helps process engineers set optimal parameters without wasting material on physical test runs. Twins can also simulate how variations in input materials might affect output. If a certain batch of raw material is slightly off-spec, the twin can predict if the current process settings will still yield an acceptable product or if adjustments are needed.
Training and Ergonomics: Some factories use VR and digital twins to train workers on new processes before they go live. If a assembly process is changing, you can have a virtual workstation twin where workers practice the new method in VR, getting used to new tool placements or sequences. This makes the learning curve shallower when the real change happens, reducing slowdowns or errors. It’s similar to flight simulators for pilots, but for production line operators or maintenance technicians. This also extends to ergonomics: a twin can simulate a human doing a task to see if it might cause fatigue or injury (using biomechanics data). The process can then be redesigned for safety and comfort (e.g., adjusting the height of a workbench) before physically reconfiguring anything.
Case Example: Boeing has been known to use digital twins extensively in production of airplanes. When they planned the 777x assembly line, they built a digital twin of the assembly process. Through simulation, they found ways to automate certain steps and optimize tool placements, targeting a reduction in assembly time per aircraft. This was pivotal for hitting production rate targets. Another example: Siemens uses digital twins in its own factories (so-called “digital factories”). In Amberg, Germany, Siemens has a highly automated electronics plant where the digital twin of the factory helps achieve nearly zero downtime. They simulate maintenance and upgrades in the twin first, so line changeovers that used to take hours or days can happen in minutes with the confidence gained from simulation.
Additive Manufacturing (3D Printing): This is an area where digital twins are emerging. The 3D printing process can be simulated to predict part distortion, residual stresses, etc. Companies like GE have digital twins of their additive manufacturing process that adjust machine parameters in real-time to ensure the printed part comes out exactly as intended, reducing trial prints and errors.
Results: Manufacturing twins often yield benefits like shorter time to market (by reducing physical prototyping and speeding up line ramp-ups), higher OEE (Overall Equipment Effectiveness) due to less unplanned downtime and better scheduling, and improved yield and quality (because issues are caught and corrected in the digital realm). According to an analysis by Deloitte, factories implementing IoT and twin technologies have seen up to 10-12% gains in efficiency and 20% reduction in quality issues in early stages of adoption.
What’s key is that the digital twin becomes part of the continuous improvement cycle. It’s not one-and-done; it’s constantly updated with production data, so it grows more accurate, and engineers and managers use it routinely to test any idea – from minor tweaks to major overhauls – virtually first. In essence, it de-risks innovation on the factory floor. As HI-GTM often tells clients: in manufacturing, “measure twice, cut once” is now “simulate twice, implement once.” It’s a new paradigm of informed, data-driven operational excellence.
6.6 Logistics and Route Planning
The logistics sector – encompassing transportation, distribution, and delivery – is a natural fit for digital twin technology. Here the emphasis is on route optimization, fleet management, and network planning. When we talk about a digital twin for logistics, it usually means a virtual model of the transportation network and its assets (trucks, ships, routes, distribution hubs), updated with real-time data like vehicle locations, traffic, and shipment statuses.
Route Planning and Optimization: The daily challenge for logistics is figuring out the best routes and schedules to get goods from point A to B (and C and D…) efficiently. Traditionally, this is handled by optimization algorithms in transportation management systems. The digital twin takes it further by allowing planners to simulate different strategies under varying conditions dynamically. For instance, imagine a twin of a courier delivery network for a city. It has every driver’s route, every package’s destination, and real-time traffic data. Now, say an unexpected road closure pops up downtown at 10 AM – the twin can simulate rerouting all affected deliveries through alternate streets and immediately project new ETAs and fuel usage. The dispatcher can then send updates to drivers based on the optimal reroutes the twin discovered.
Similarly, for long-haul trucking, a twin might simulate how changes in departure times affect delivery. If severe weather is forecast on a route tomorrow afternoon, the twin could suggest dispatching trucks a few hours earlier (or taking a different route or holding them until the storm passes) by simulating those scenarios and comparing outcomes (late delivery risk vs. safety vs. cost).
Fleet Management and Maintenance: Logistics isn’t just about routes, it’s also about keeping vehicles running. A fleet of delivery vans can have a digital twin that monitors each vehicle’s health (through telematics data like engine diagnostics, tire pressure, etc.). This merges into predictive maintenance: scheduling maintenance when the twin predicts a breakdown is imminent rather than on a fixed schedule. Also, if a vehicle does go down, the twin of the network can quickly reassign its deliveries to others and adjust routes – essentially simulating “removal of one node” and solving around it. This minimizes service disruption. Companies like UPS and FedEx invest heavily in such routing twins (UPS’s ORION system is akin to this, focusing on reducing mileage and fuel, famous for minimizing left turns).
Capacity Planning & Network Design: On a strategic level, logistics digital twins are used for network design – where to locate warehouses, how many trucks or planes are needed on certain lanes, etc. For example, a twin can simulate a logistics network with one extra distribution center added and show how much faster or cheaper deliveries could be for a region. It can also reveal weak links: maybe the twin shows that a particular route is consistently overloaded, suggesting adding capacity (like an extra truck making that run) or shifting some loads to a different route.
Another scenario: during holiday peak season, a shipping company can run simulations on their twin to forecast bottlenecks (perhaps a certain sortation hub gets overwhelmed around Dec 15). By identifying that early, they can add temporary labor or reroute some shipments away from that hub ahead of time.
Last-Mile Delivery and Drones/Robots: For the cutting-edge, some are creating twins for last-mile delivery systems including delivery drones or sidewalk robots. Before deploying a swarm of delivery drones in a city, companies simulate it: how they navigate, where they might conflict, battery usage vs. route distance, etc. A twin can help plan drone placements and pathways to ensure efficient coverage without mid-air traffic jams. It’s very futuristic, but such twins are in development.
Real-world Example: FedEx has talked about using a digital twin of their package routing to dynamically adjust to conditions. If an important package is at risk of missing a connection, their system can flag it and re-prioritize it via a different route, which is essentially the twin evaluating alternatives. On a simpler note, many trucking companies use digital route simulations to educate drivers – like showing them how a slight change in route or reduction in idling time would save X fuel and time, often gamifying it. This is more of a digital coaching twin for behavior optimization.
ROI for Logistics Twins: The benefits often manifest as cost savings (fuel, labor hours), improved delivery performance (on-time percentage), and greater agility in face of disruptions. Oracle’s IoT Cloud, for instance, has been noted to help companies streamline supply chain operations and improve delivery times via digital twin capabilities. Even a 5% reduction in miles driven across a large fleet can save millions in fuel and vehicle wear-and-tear annually, not to mention the sustainability angle (lower carbon emissions).
Another advantage is customer satisfaction – today’s customers expect real-time updates and fast delivery. A well-simulated logistics twin means you can promise tighter delivery windows and keep them, because you’ve already rehearsed the route plan for that day. If something goes awry, the twin helps you adapt quickly and still meet promises or at least inform customers proactively with new ETAs.
In summary, for logistics and route planning, a digital twin serves as a high-speed, all-seeing dispatcher and analyst. It looks at the entire system holistically and finds efficiencies or contingency plans that no single human planner could compute in the same time. In a world where free shipping and next-day delivery are now the norm, that level of optimization can be a decisive factor in maintaining margins and service quality. The digital twin essentially helps logistics providers do more with less – carrying the same load (or more) with fewer trucks, less fuel, and less chaos, by simulating the journey to perfection before it’s taken.
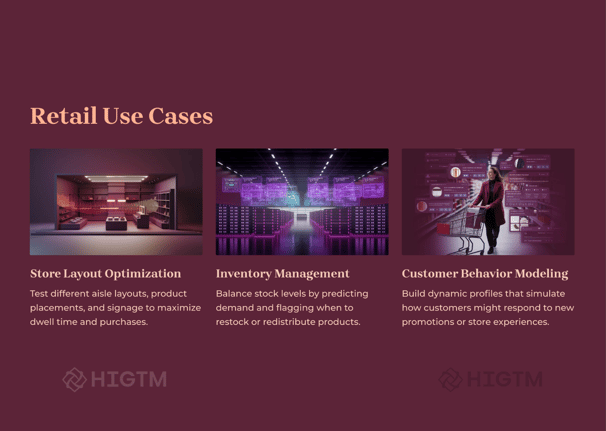
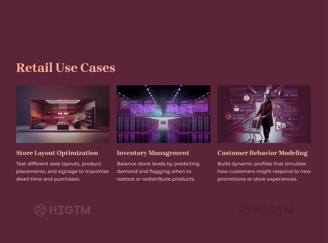
7. Conclusion and Future Outlook
Digital twins have moved from buzzword to real-world application, delivering tangible benefits across industries. As we’ve seen, they empower businesses to simulate operations before real-world changes, reducing uncertainty and risk in decision-making. Whether it’s a retailer redesigning store layouts, a manufacturer ramping up a new production line, or a logistics manager re-routing deliveries on the fly, digital twins provide a data-driven crystal ball. Companies that leverage this technology effectively are finding they can innovate faster, optimize continuously, and respond to disruptions with agility – in short, they gain a significant competitive edge.
A key enabler in the twin revolution is the synergy with AI and machine learning, which turns these virtual models into intelligent advisors (and even autonomous actors) in the management of operations. As AI models become more sophisticated, we can expect digital twins to shift from being mostly human-in-the-loop decision support tools to being more autonomous control systems for certain tasks (with human oversight). For example, a future smart factory might have a digital twin that not only predicts issues but also automatically adjusts processes in real time to prevent them, essentially running a self-optimizing loop. This is the frontier of Industry 4.0 and even the upcoming Industry 5.0, where human expertise and AI-driven twins collaborate seamlessly.
On the horizon, the integration of digital twins with emerging technologies opens exciting possibilities. Edge computing will allow twins to operate with real-time data even when connectivity to cloud is limited (imagine a twin on an oil rig far out at sea). 5G networks will accelerate the flow of data from countless sensors, making twins even more granular and responsive. Augmented reality (AR) will likely bring twins to front-line workers in a very visual way – a technician could “see” the twin data overlaid on a machine through AR glasses, making maintenance or training more effective.
We should also mention the concept of “digital thread”, which complements the digital twin. A digital thread is like an end-to-end data flow that ties together every stage of a product or process lifecycle. Digital twins at each stage (design, production, operation, service) feed into this thread. The result is that knowledge and data flow uninterrupted – a design change automatically updates manufacturing instructions and field service guidelines through their respective twins, for instance. This holistic approach could cut development cycles dramatically and ensure everyone is working off the most current information.
Compliance and security will remain critical. As digital twins permeate more of business operations, they become part of the core infrastructure that must be safeguarded. We anticipate more clear guidelines and perhaps industry standards on securing digital twin environments, and possibly even audits specifically focused on twin systems (similar to how IT systems get audited). Privacy concerns will also evolve – if we have digital twins of customers or even employees for performance (think “digital twin of an employee’s workday” to optimize productivity – a potentially sensitive area), organizations will need ethical frameworks and transparent policies to govern their use.
From a strategic perspective, adopting digital twins is not just a technology project, but a change management journey. It requires upskilling staff to work with data and simulations, breaking silos so that IT, operations, and business strategy collaborate, and fostering a culture that trusts data-driven insights. Companies like HI-GTM play a crucial role in guiding businesses through this journey – helping identify high-impact use cases, choosing the right technology stack, and ensuring that outcomes align with business goals. It’s about translating the digital twin’s potential into real ROI and competitive positioning.
In conclusion, the rise of digital twins marks a significant shift in how we approach problem-solving and innovation. We no longer have to rely solely on backward-looking data or risky real-world experiments. Instead, we create a living, predictive model of our world where we can test, learn, and optimize at the speed of computers. It’s a world where a thousand “what ifs” can be explored over a coffee break, and the best path forward discovered, rather than debated. For executives and managers, this means decisions can be made with unprecedented confidence. For customers and stakeholders, it means a smoother, more responsive experience as companies anticipate needs and issues before they manifest. And for the industries at large, it heralds a future of efficiency, resilience, and innovation that will reshape how we design, operate, and improve the products and services that drive our economy.
The organizations that embrace digital twins now – thoughtfully and strategically – will be the ones writing the success stories of tomorrow. As the technology continues to mature and integrate with AI, it’s fair to say we are just at the early stages of the digital twin journey. The next few years will likely see digital twins move from project pilots to standard operating procedure in many businesses. Those that lead the charge, with strong partners like HI-GTM by their side, will stand to reap the rewards of being simulation-driven, data-savvy enterprises in an increasingly complex world. The old adage was “change is the only constant”; with digital twins, we add a new twist – change is constant, but now we can simulate and master it before it happens.
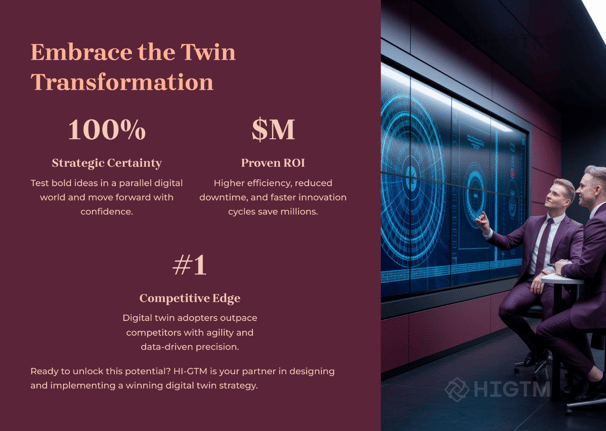
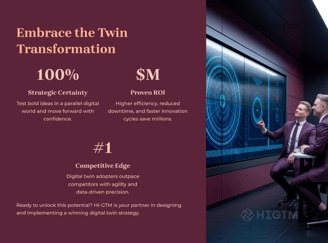
Turn AI into ROI — Win Faster with HIGTM.
Consult with us to discuss how to manage and grow your business operations with AI.
© 2025 HIGTM. All rights reserved.