71. AI Governance Frameworks for E-Commerce Supplement Brands - Combining global compliance, ethics, and risk management for retail AI success
Artificial intelligence is powering new opportunities in retail – from personalized vitamin recommendations and smart chatbots to automated supply chain decisions. For e-commerce supplement brands, AI can boost efficiency and customer engagement. But without proper oversight, the same AI systems can introduce serious risks, from privacy violations to biased outcomes. Ensuring AI systems are compliant with laws and aligned with ethical standards is now a strategic priority. In this article, we present a structured AI governance framework covering compliance, ethics, and operational risk management. You’ll learn how global industry leaders (Amazon, Walmart, Alibaba, etc.) govern AI and how to apply these best practices to your own retail brand.
Q1: FOUNDATIONS OF AI IN SME MANAGEMENT - CHAPTER 3 (DAYS 60–90): LAYING OPERATIONAL FOUNDATIONS
Gary Stoyanov PhD
3/12/202561 min read
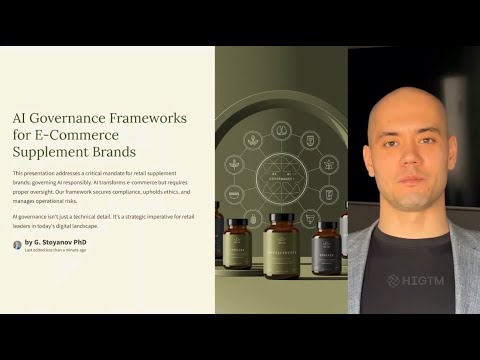
1. Why AI Governance Matters for E-Commerce Supplement Brands
1.1 The Growing Role of AI in Retail
AI has rapidly woven itself into the fabric of retail operations. E-commerce supplement brands use AI to personalize product recommendations (“Customers who bought Vitamin D also like…”), manage inventory (predicting stock levels for protein powders), handle customer inquiries via chatbots, and even to create marketing content. These intelligent systems can analyze vast consumer data – purchase history, browsing behavior, health goals – to drive sales and improve customer experience. In short, AI offers supplement retailers a powerful tool to increase efficiency and connect with customers in a targeted way.
However, as AI’s role grows, so does its impact. Decisions that were once made by humans (like which supplement to upsell to a customer) are now sometimes made by algorithms. This transfer of decision-making means brands must ensure AI actions align with company values and policies just as a human employee’s decisions would. Unlike a human, an algorithm won’t inherently know the boundaries of fairness or privacy unless we instill those guidelines. That is why governance is essential – it’s the process of embedding our human values and legal obligations into AI systems from design to deployment.
1.2 Risks of Unchecked AI in Retail
While AI can deliver great benefits, unchecked AI can backfire badly. One major risk is bias and unfairness. If an AI model is trained on skewed data, it may, for example, start favoring certain products or customers unfairly. A notable case was observed by Alibaba: their e-commerce recommendation algorithm tended to create a “Matthew Effect,” where top sellers kept getting more visibility at the expense of new entrants. Without intervention, this kind of bias not only harms smaller merchants but can also reduce the diversity of products shown to customers. It took conscious governance for Alibaba to recognize and correct the imbalance in their algorithm.
Another risk area is privacy and security. AI systems in retail often crunch personal data – purchase histories, demographic info, maybe even wellness data from customers. If not carefully controlled, this could lead to privacy violations. For instance, a personalization algorithm might infer sensitive health information about a customer (like pregnancy or a medical condition) and target them with supplements in a way that feels invasive. This could breach privacy laws or simply erode customer trust if they feel spied upon. Unprotected AI systems are also targets for cyber attacks; a hacker might try to manipulate an AI (through data poisoning or adversarial inputs) to produce harmful outputs.
Regulatory and legal risks loom large as well. Governments are holding companies accountable for what their algorithms do. An AI-driven pricing error that overcharges certain customers could lead to discrimination claims. Or an automated marketing message that violates health claim regulations could attract regulatory penalties. These aren’t hypothetical – regulators have already fined companies for data misuse and unfair practices, and AI could trigger those issues if unmanaged.
Finally, there’s reputational risk. In the age of social media, a single AI misstep – say a chatbot giving a terribly inappropriate answer – can go viral and damage a brand’s image overnight. Customers expect brands to use AI responsibly. Surveys consistently show that consumers are wary of AI’s influence; if a company can’t demonstrate control over its AI, people may take their business elsewhere.
In essence, without governance, AI can become a high-risk experiment. As one example of things gone wrong, Amazon famously had to scrap an AI recruiting tool that learned to discriminate against women – a PR black eye and a waste of resources. Retail supplement brands must learn from such examples. By implementing AI governance, you actively prevent biases, protect customer data, ensure compliance, and safeguard your brand reputation. Governance is what allows you to reap AI’s rewards safely – it’s the fail-safe that every high-stakes innovation needs.
2. Regulatory Compliance Landscape for AI
2.1 Data Privacy Laws (GDPR, CCPA) and AI
Any discussion of AI governance in retail must start with data privacy compliance. The EU’s General Data Protection Regulation (GDPR) and California’s Consumer Privacy Act (CCPA) are two landmark laws that directly impact how companies can use consumer data in AI systems.
Under GDPR, personal data can only be processed on specific legal bases (like user consent or legitimate interest) and for declared purposes. GDPR enshrines principles like data minimization, transparency, and fairness in all data processing, AI included.
For instance, GDPR’s data minimization means an AI system should not hoover up more personal data than necessary for its task. If your supplement recommendation AI doesn’t need a customer’s precise birthdate or ethnicity, you shouldn’t be collecting it – that reduces risk and respects privacy. GDPR also gives individuals rights such as the right to access their data, correct it, or even delete it. This means your AI pipelines must be designed to allow data to be removed or updated if a customer requests – not always a trivial task if data is deep in training models, but necessary.
Crucially for AI, GDPR’s Article 22 gives EU individuals the right not to be subject to purely automated decisions that have significant effects on them, unless certain conditions are met (like explicit consent or necessary for a contract). While a product recommendation or personalized ad in retail is usually not “legally significant” enough to trigger Article 22, things like automated credit approvals or pricing decisions might. Brands need to be mindful: if you ever use AI to, say, automatically set insurance premiums for wellness programs or determine eligibility for a special offer, you may need to provide an opt-out or human review to EU customers. Even when Article 22 doesn’t strictly apply, the spirit of GDPR pushes us toward humans and AI working together, not AI making important calls in a black box.
The CCPA, on the other hand, gives California consumers rights to know what personal data is collected and for what purpose, to opt out of the sale of their data, and to request deletion of their data. For an AI context, if your supplement brand’s website uses an AI-driven personalization service that involves sharing data with a third-party, that might count as a “sale” of data under CCPA definitions – meaning you must offer an easy opt-out (“Do Not Sell My Personal Information”) for California users. CCPA is less prescriptive than GDPR (it doesn’t mandate principles like data minimization as explicitly), but it still demands transparency. You should be able to explain in your privacy policy how any AI algorithms are using consumer data. Additionally, multiple U.S. states (like Virginia, Colorado, others) are enacting their own privacy laws similar to CCPA, creating a patchwork – so a best practice is to meet the highest common standard to simplify compliance.
It’s important to note that these privacy laws apply to AI because AI runs on data. As one commentary put it, as soon as AI uses personal data, GDPR is triggered.
So AI doesn’t exist in some legal vacuum – the same rules of consent, purpose limitation, and security apply. In fact, regulators often scrutinize AI projects under existing privacy laws through mechanisms like Data Protection Impact Assessments (DPIAs). GDPR requires DPIAs for high-risk data processing, which can include certain AI profiling activities.
If you launch a new AI tool that does extensive user profiling, performing a DPIA isn’t just compliance – it’s a governance exercise to identify and mitigate risks early.
In practice, ensuring compliance means building privacy-by-design into your AI systems. This includes steps like anonymizing or pseudonymizing customer data before using it to train AI models, prompting users for consent in clear language if AI will use their data in non-obvious ways, and having retention schedules so data isn’t kept longer than necessary. It also means training your staff on these laws – everyone from data scientists to marketing managers should know the basics of GDPR/CCPA. Ignorance is not bliss; authorities have not shied away from heavy fines (GDPR fines can go up to 4% of global annual turnover for serious infringements). While a small supplement brand might not face a multi-billion euro fine, even smaller fines or legal action can be devastating to your finances and reputation.
To summarize, compliance is a foundational pillar of AI governance. By adhering to GDPR, CCPA, and other privacy regulations, you not only avoid penalties but also earn customer trust. When users see that you handle their data responsibly (e.g., giving clear privacy notices and honoring opt-outs), they are more comfortable with your AI-driven services. It’s a win-win: compliance protects your business and shows respect for your customers – an essential recipe for long-term success.
2.2 The EU AI Act and Emerging AI Regulations
Beyond data privacy, governments are introducing AI-specific regulations. The most notable is the upcoming EU AI Act, which, once in force, will be the first comprehensive law regulating AI systems. It follows a risk-based approach :
Unacceptable risk AI – uses of AI that threaten safety or fundamental rights (like social scoring or real-time biometric surveillance in public) will be outright banned.
High-risk AI – AI systems in sensitive areas (healthcare, finance, employment, law enforcement, etc.) will be allowed but heavily regulated. Providers of high-risk AI must meet strict requirements: robust risk assessment, high-quality data, transparency, human oversight, and obtain certification (a conformity assessment) before deploying.
Limited risk AI – these include AI like chatbots or deepfakes that interact with people. They won’t need upfront certification but will have transparency obligations (e.g., you must inform users that they’re interacting with an AI, and clearly label deepfake content as AI-generated).
Minimal risk AI – all other AI (e.g., spam filters, AI in video games) is largely unregulated by the Act aside from basic principles, similar to how most software is treated.
For a retail supplement brand, most AI systems you use (recommendation engines, customer chatbots, inventory optimizers) would likely fall in the limited or minimal risk categories. That means the EU AI Act might require you to be transparent (telling users a chatbot is an AI, for example), but you wouldn’t face the full brunt of high-risk system rules unless you venture into areas like AI for medical advice or credit services. However, if your brand’s AI ventures into something like analyzing customer health data to make personalized supplement plans, regulators might view that as a higher risk (health-related AI can become high-risk). You’d then need to implement the Act’s requirements: rigorous testing for accuracy, keeping detailed technical documentation, enabling human oversight, etc.
Even if your current AI use is low-risk, the EU AI Act is a harbinger of global standards. It effectively sets a benchmark for AI governance. Many of its practices – risk assessments, transparency measures, monitoring for bias, etc. – are simply good governance that you might want to do voluntarily.
And if you operate internationally, complying with the Act will be necessary for EU markets anyway. It’s wise to start mapping your AI inventory and see if any system might be considered “high-risk” under the Act’s definitions. For instance, using AI to automatically filter job applicants or to set insurance pricing for wellness plans could be high-risk, whereas using AI for product recommendations would be limited risk requiring transparency.
Outside the EU, other jurisdictions are also moving on AI regulation. China has issued ethical guidelines and regulations focusing on algorithmic fairness and transparency. In early 2022, China implemented rules on recommendation algorithms that, among other things, ban algorithms from engaging in unfair price discrimination against consumers.
This is directly relevant to e-commerce: it means an online platform shouldn’t use AI to show different prices to different people in an exploitive way. Chinese regulations also require recommendation AI to incorporate ethical principles and not endanger public order.
In the United States, there isn’t a federal AI law yet, but there are sectoral regulations (like FDA oversight for AI in medical devices) and active discussions about principles. The NIST AI Risk Management Framework released in 2023 is a voluntary set of guidelines that companies are using to self-regulate – it covers identifying AI risks, mitigating them, and governance structures. There’s also movement at the state level (e.g., Illinois has an AI Video Interview Act requiring notice and consent for AI analysis in hiring). And notably, the White House secured voluntary commitments from leading AI companies (including Amazon, Google, Meta, Microsoft) to ensure AI safety and security – signaling that more formal regulation could follow. Areas like transparency, bias avoidance, and cybersecurity in AI are emphasized in those commitments.
The takeaway for a supplement brand is that regulatory compliance in AI goes beyond just data privacy. You need to keep an eye on emerging rules that target AI directly. Adopting a proactive stance is key: implement internal policies now that meet or exceed these new standards.
For example, start documenting your AI systems and decisions in anticipation of laws that might require such documentation. If you have a recommendation algorithm, consider providing an explanation feature (like “Why am I seeing this product?”) to align with the spirit of transparency requirements. Being ahead of regulation not only avoids last-minute scrambles, but it positions you as a trustworthy leader in the eyes of consumers and partners.
In summary, the global regulatory landscape is evolving quickly. Europe’s AI Act will likely set the tone, influencing laws in other countries or at least establishing best practices. Meanwhile, privacy laws like GDPR/CCPA already apply. A robust AI governance framework will ensure you comply with current laws and are nimble enough to adjust to new ones. Compliance isn’t just about avoiding penalties; it’s part of your brand’s promise to customers that you use technology responsibly. Embracing that mindset now will make regulatory compliance a natural outcome of doing the right thing.
3. Embedding Ethical AI Principles in Retail Operations
3.1 Fairness and Bias Mitigation
Fairness is a cornerstone of ethical AI. In the context of retail and supplements, fairness means ensuring your AI systems treat individuals and groups equitably and do not reinforce discrimination or unethical biases. This matters both legally (to avoid discriminatory outcomes that could violate laws) and morally (to uphold your brand’s values of inclusivity and equal opportunity).
AI systems can inadvertently introduce or amplify bias because they often learn from historical data, which might reflect societal biases. For example, if an AI notices that high-income customers bought more premium supplement bundles in the past, it might start favoring showing expensive products to male users over female users (if income levels differed historically), or urban over rural customers, etc. Without checks, this could lead to uneven customer experiences or opportunities. In hiring or promotions (even indirectly via AI scanning resumes for a new store nutritionist), biased AI could filter out qualified candidates from underrepresented groups – as happened with Amazon’s recruiting AI that was found to be downgrading female applicants.
To mitigate bias, the governance framework should include bias testing and audits at multiple stages of the AI lifecycle. This involves measuring outcomes by different demographics or segments to see if the AI is treating anyone unfairly. For a recommendation engine, you might test whether it systematically suggests cheaper products to one group of customers and premium products to another in a way that isn’t justified by their behavior. If such patterns are found, the AI model or rules should be adjusted.
Leading companies embed fairness commitments formally. Walmart, for instance, has pledged to evaluate its AI tools for bias and mitigate any unjust impacts.
This is part of their six responsible AI commitments, directly addressing fairness. What does that look like in practice? It means Walmart will, say, check an AI scheduling tool to ensure it isn’t giving better shifts only to certain employees or ensure their customer service chatbot treats all dialects of English equally without bias. Your brand should adopt a similar practice: regular bias audits of AI systems that affect customers or employees.
One effective tactic is to form a review team (which could be part of the AI governance committee) that specifically looks at AI outputs for fairness. They can use techniques like counterfactual testing (tweaking input data slightly – for example, change a customer’s gender in the data – and seeing if the output changes inappropriately) or protected class analysis (checking outputs across gender, age groups, etc.). Additionally, ensure diversity in the team developing and testing AI. Different perspectives can spot biases others miss.
If a bias is identified, document it and address it. Sometimes it might require collecting more diverse training data, or excluding a problematic data attribute, or applying algorithmic techniques to adjust outcomes (like equalizing recommendation exposure across seller types). In other cases, the solution might be putting a human in the loop for certain decisions to add judgment that the AI lacks.
Remember that fairness isn’t just about avoiding bad outcomes; it’s also about actively pursuing equitable outcomes. For example, Alibaba saw their platform’s AI was giving established sellers a big advantage. They responded by changing the system to give new sellers some exposure. That’s governance turning into a competitive and ethical advantage – new merchants got a fair shot, customers saw a wider variety of products, and the platform’s vibrancy improved.
In sum, for fairness: make it a KPI. Just as you track sales lift from AI, track fairness metrics (like distribution of recommendations or approval rates across groups). By weaving bias mitigation into your AI development and monitoring processes, you uphold an ethical standard and reduce the risk of discrimination complaints or toxic PR. Over time, a reputation for fairness can become a selling point, especially in an era where consumers are alert to issues of bias and representation.
3.2 Transparency and Explainability
Transparency is about being open and clear regarding how your AI systems operate, especially in their interactions with people. Explainability is a related concept – it means having the ability to explain an AI’s decision in understandable terms. In retail, these principles significantly boost customer trust and can be a legal requirement in some jurisdictions (and are emphasized in guidelines like the EU’s trustworthy AI principles).
What does transparency look like for a supplement brand using AI? At a basic level, it means telling users when AI is in play. For example, if a customer is chatting with a support agent that is actually an AI chatbot, you should disclose that it’s a virtual assistant. Hiding AI can lead to feelings of deception if customers figure it out. A simple statement like, “Hi, I’m an AI assistant, here to help you with product questions!” sets the right expectation. Transparency also involves data practices – e.g., letting users know that “we use an AI recommendation engine that considers your browsing and purchase history to suggest products.”
In user interfaces, providing explanations for recommendations or decisions can demystify AI. You might implement a feature like: “Why am I seeing this supplement recommendation?” and have the AI provide a reason: “We suggested this because you indicated interest in fitness and this protein has high ratings from buyers with similar goals.” This kind of explainability not only satisfies curiosity but allows the user to correct the system if the reason is off (“Actually, I’m not into high-protein diets”). It makes the AI interaction a two-way street rather than a black box.
From a governance standpoint, achieving explainability means choosing AI models and techniques that are interpretable or developing methods to interpret them. Some advanced AI algorithms (like deep learning neural networks) can be like “black boxes.” But there’s a growing field of XAI (eXplainable AI) providing tools to interpret such models (like SHAP values or LIME for feature importance, which highlight what factors influenced a particular decision). Your data science team should integrate these tools so that for any given automated decision – say an AI decides to flag a transaction as fraud or decides not to show a product to a user – you can audit why it did that.
Transparency also extends to regulators and internal stakeholders. Document your AI systems: what data they use, how they make decisions, and what testing you’ve done. That way, if questions arise (internally or externally), you have answers ready. Amazon, for example, has committed to publicly reporting their AI systems’ capabilities, limitations, and appropriate use cases.
While you may not need to publish a report for each system, you should maintain internal documentation at least.
Another facet is algorithmic transparency in policy. If your AI inadvertently does something that affects users significantly, proactively inform users. For instance, if a pricing AI had a glitch and showed wrong prices, come clean about it and fix the error. People are forgiving when you’re transparent and take responsibility, but they are unforgiving if they sense a cover-up or discover an issue themselves that you kept quiet.
Industry leaders highlight transparency. Walmart’s AI principles begin with Transparency – they commit to helping customers, members, and associates understand how AI is used in the company.
That might mean clear signage (e.g., if Walmart uses shelf-scanning robots, a sign might explain their purpose) or communications about new AI features and what data they utilize. Emulating this, your brand could write blogs or help-center articles explaining your AI-driven personalization, including what data is considered and how it benefits customers (and reassuring them of privacy protections in place).
Explainability isn’t just for customers; it’s also critical for your internal decision-makers. If an AI recommends reducing stock of a supplement, your inventory manager should be able to get an explanation (“sales trends for the last 8 weeks suggest lower demand”) to trust that recommendation. Without that, employees might resist using AI outputs. So, governance should ensure AI decisions can be questioned and understood internally as well.
In summary, transparency and explainability turn AI from a magic box into a collaborative tool. They fulfill regulatory expectations (like the EU AI Act’s transparency requirements for AI that interacts with people) and align with ethical best practices. By being transparent, you signal respect for your customers’ right to know and control their experience. By ensuring explainability, you maintain oversight and trust in the system’s functioning. Both are essential for integrating AI smoothly into business processes without alienating the people involved – whether they are customers, employees, or regulators.
3.3 Accountability and Governance Structures
For AI to be used responsibly, there must be clear accountability – people or teams who are answerable for the behavior of AI systems. “The AI did it” is not an acceptable excuse in governance. Your organization should treat AI outcomes as ultimately a human responsibility. This is why establishing governance structures, such as committees or designated roles, is a key part of an AI governance framework.
Many forward-thinking companies create an AI or technology ethics committee to oversee major AI deployments. Alibaba, for example, formed a Technology Ethics Committee chaired by their CTO, bringing together experts in technology, law, and ethics. This committee’s job is to be a “gatekeeper for tech innovation” – essentially, ensuring that new AI systems meet ethical standards and align with the company’s principles. They set guidelines and can veto or require changes to projects that don’t measure up. In practice, a committee like this might review a proposal to use AI for analyzing customer health data and impose conditions (like adding stricter privacy safeguards or narrowing the scope) before approving it.
If you’re a smaller company and a full committee feels heavy, you can still assign an AI governance champion – maybe a Chief Data Officer or a Head of Compliance with knowledge in AI – who chairs a working group on AI oversight. The key is cross-functional involvement: include someone from legal/compliance (for regulatory perspective), someone from the technical side (data science/IT), and someone representing business interests (like marketing or operations). This ensures that decisions around AI consider multiple angles.
Accountability also means that for each AI system, it’s clear who is managing it and who reviews its outcomes. For instance, if you deploy an AI that automates discount offers to customers, decide which manager is responsible for its performance and fairness. That manager should regularly review metrics and also respond if something goes wrong. Walmart’s Responsible AI principles explicitly highlight accountability: they pledge that AI will be “managed by people” and that the company holds itself accountable for its impact.
That might mean requiring a human sign-off on certain AI-driven decisions, or it could mean performance reviews include metrics from AI systems that a manager oversees.
An effective practice is to integrate AI accountability into existing structures. If you have a risk management committee or an IT governance board, slot AI into their agenda. Alternatively, add AI risk review as part of your regular compliance audits. Some companies tie it into their Ethics and Compliance program – e.g., extending their Code of Conduct to cover AI usage (Netflix famously included “no unethical use of data” in employee guidelines, which would cover AI as well).
Another important aspect is escalation paths and incident handling. Employees should know where to report an AI-related concern (say, if someone in customer service notices the chatbot giving potentially biased answers, or a data analyst finds something odd in model behavior). Your governance structure should define how such concerns are evaluated and addressed. Is there a fast-track way to shut off or rollback an AI system if a serious issue is found? Who has the authority to do that? Deciding these beforehand is critical – you don’t want to be figuring out the chain of command in the middle of a crisis.
Having accountability also means keeping leadership informed. Boards of directors are increasingly interested in how companies manage AI risk. Including AI governance in board reports or annual CSR (Corporate Social Responsibility) reports can show that the company is on top of the issue. Some organizations even create an external advisory council to get independent feedback on their AI ethics (for example, Microsoft has an external AI advisory board in addition to its internal Office of Responsible AI).
In short, accountability is about putting human oversight and ownership around AI. That can be accomplished through structures (committees, roles) and processes (reviews, reporting channels). The tone from the top matters too – if executives openly champion responsible AI use and empower governance bodies, it creates a culture of accountability. When everyone knows that AI isn’t a wild experiment but rather a well-supervised tool, the company can innovate with confidence. As a retail executive, you want to be able to say, “Yes, we use advanced AI – and we are fully in control of what it does.” Governance structures make that statement true.
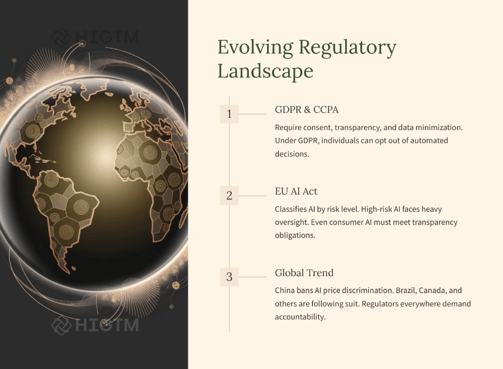
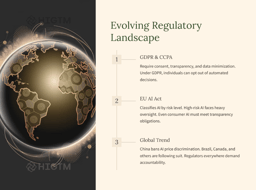
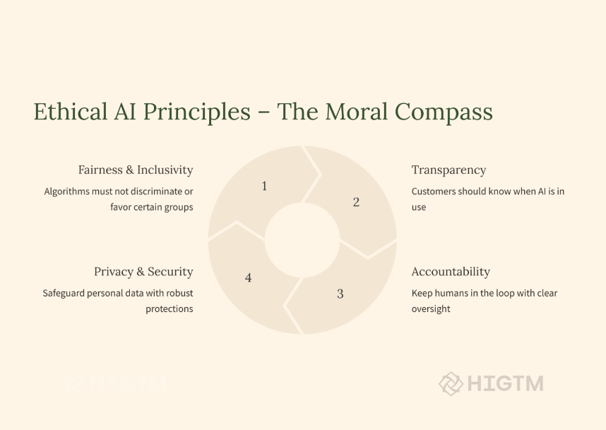
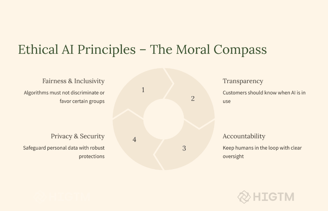
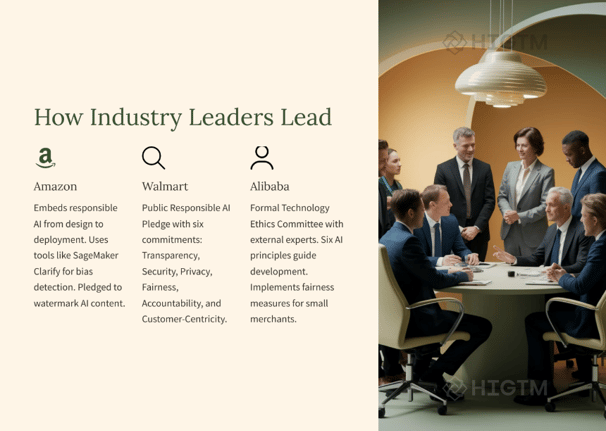
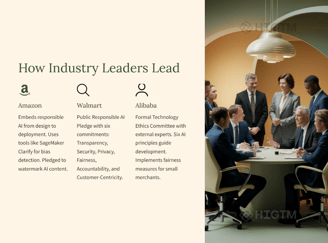
4. Operational Risk Management for AI Systems
4.1 Risk Assessment and Continuous Monitoring
AI systems, like any other critical business system, require ongoing risk management. This starts with risk assessment – identifying what could go wrong – and continues with continuous monitoring to catch issues early.
A prudent step is to conduct an AI risk assessment for each significant AI application. This means systematically thinking through possible failure modes or negative impacts. For a supplement brand, consider an AI-driven product recommendation engine: risks might include recommending an unsafe combination of supplements to a user, or systematically excluding certain products due to a quirk in data, or a bug that causes incorrect pricing. By listing these, you can then plan controls for each (e.g., have a nutritionist review the recommendation rules to ensure safety, ensure a diverse product pool is used, set up alerts for price anomalies).
Regulators and standards bodies have begun pushing formal risk management for AI. For instance, the NIST AI Risk Management Framework (in the US) suggests practices like mapping AI use cases, measuring risks, and monitoring effectiveness of mitigations. The EU AI Act will require high-risk AI providers to implement risk management systems throughout the AI lifecycle – including evaluating residual risk and determining if the level is acceptable or not. Even if your AI isn’t “high-risk” by law, adopting a similar mindset is wise governance.
After assessment, you need continuous monitoring of AI in production. AI models can degrade over time (a phenomenon known as “model drift”) as user behavior or data patterns change. What was accurate last year may become less so this year, leading to rising error rates or biased outcomes. By monitoring key performance indicators (KPIs) of your AI, you can detect drift or emerging problems. For example, you might track the conversion rate of AI-generated recommendations – if it plummets, maybe the model lost relevance. Or monitor the distribution of recommendations – if suddenly the top 10% of products are all that’s ever recommended, something might be off.
Set up alerts and review cycles. If you have thresholds (like, if the recommendation diversity index falls below X, or if any demographic is getting offers at half the rate of another), an alert triggers a review. Some companies practice “live auditing” – e.g., randomly sample AI decisions periodically and have an auditor (human) verify them. In a retail context, that could mean reviewing a sample of personalized marketing messages each month for compliance and tone.
Another aspect is monitoring the external environment for new risks. Perhaps a new regulation is passed that affects your AI, or a competitor had a public AI failure you can learn from. Incorporate those lessons. For example, if another retailer’s dynamic pricing AI was criticized for price-gouging during emergencies, you might proactively ensure your algorithms have rules against raising prices on essential goods during crises.
From an operational standpoint, document mitigation plans for identified risks. If the risk is “AI might recommend a supplement that conflicts with another in the cart,” the mitigation might be “build a conflict-checking rule into the recommendation system”. If the risk is “model may drift and become less accurate over time,” mitigation is “retrain model every 3 months with latest data and validate performance”. Each major AI system should have a mini “risk register” where these are recorded.
It’s also beneficial to perform scenario planning for worst-case AI failures, similar to disaster recovery drills. Ask, what would we do if our AI made a harmful recommendation that went viral on social media? Having a plan (take system offline, issue apology, fix error, etc.) prepared will save precious time and reduce chaos if it ever occurs.
In highly sensitive AI uses, consider employing external auditors or using third-party AI audit services. An independent review can catch issues internal teams might overlook and will add credibility if you need to demonstrate governance to regulators or business partners. In some sectors, audits might become mandatory (the EU AI Act may effectively mandate some form of third-party assessment for high-risk AI). Proactively doing it for critical systems like anything affecting health advice can put you ahead of the game.
In summary, AI risk management is an ongoing process, not a one-time checklist. By integrating AI into your existing risk management and quality assurance processes, you maintain vigilance. This continuous oversight is what prevents small issues from growing into big failures. It’s analogous to financial auditing – you don’t just trust that all transactions are fine; you regularly reconcile and check for anomalies. In AI, we must do the same with our algorithms’ “decisions.” With diligent monitoring, your team can catch an off-course AI and correct it long before it crashes into an iceberg.
4.2 Security and Robustness of AI Systems
When thinking of AI governance, it’s easy to focus on ethics and compliance and overlook that AI systems themselves need to be secure and robust. These systems are software at their core, and they can have vulnerabilities like any other software. Additionally, AI introduces new kinds of vulnerabilities, such as the risk of adversarial attacks where malicious inputs can fool the model.
Security: Ensure that AI models and the data they train on are protected from unauthorized access or tampering. For instance, if you’ve developed a proprietary machine learning model that gives you a competitive edge (say, a unique algorithm predicting supplement trends), that model might be an intellectual property asset. Protect it from being stolen or leaked. Amazon and other tech companies have recognized this – Amazon committed to investing in cybersecurity and insider threat measures to protect AI model weights and systems from theft or misuse. In a retail company, this might translate to controlling access to AI model files and the datasets, using encryption, and monitoring for any unusual access patterns.
Also, consider the supply chain of your AI: if you use third-party AI services or libraries, ensure they’re from reputable sources and kept updated to patch known security flaws. Attackers could target an AI pipeline by inserting malicious code into open-source AI tools, for example. Thus, part of governance is IT governance – apply your standard cybersecurity protocols to AI servers, APIs, and data storage. Don’t let the excitement of AI cause a lapse in basic cyber hygiene like strong authentication and network security for systems running AI.
Robustness: This refers to an AI’s ability to handle unexpected inputs and conditions without failing. A robust AI won’t be easily tricked or broken by odd scenarios. For instance, an image recognition AI might be fooled by a deliberately modified image (adversarial example) that a human would still see correctly. In retail, adversarial attacks are less of a concern than in say, security screening, but robustness still matters for, say, fraud detection AIs (an attacker might try to fool your fraud model by crafting orders that evade detection).
Testing for robustness means throwing edge cases at your AI. If you have a chatbot, test it with misspellings, slang, or outrageous queries to see how it responds. If you have a recommendation AI, consider what happens if a user has very little history (cold start) or an extremely unusual combination of interests – does the AI still give sensible results? Part of governance is setting up these tests and improving the AI based on them. Perhaps you need fallback rules for the chatbot (“if input is gibberish or offensive, respond with a polite default message and hand off to human”) or constraints on recommendations (“never recommend more than X dosage, to avoid any potential health risk, no matter what the data says”).
There’s also the aspect of fail-safe design. If the AI system crashes or yields an error, what happens? For example, if your personalization engine fails for some reason, does your website have a default experience to fall back on (perhaps showing bestsellers)? Ensuring a graceful degradation is part of robust system design.
Adversarial resilience: This is advanced, but worth noting. Researchers have shown that AI models can be manipulated in ways normal software can’t. For example, subtly altering a product image might cause an AI to mislabel it. Or a user could try to reverse-engineer how your recommendation works to game the system (like repeatedly clicking certain items to trigger bigger discounts). As part of governance, stay informed about these risks and consider them for critical systems. Implementing things like rate-limiting (to prevent spamming the AI with inputs) or randomization (so the AI’s behavior isn’t too predictable) can mitigate gaming attempts.
Testing and validation are key for both security and robustness. Before fully deploying an AI, do a penetration test on the system – can someone inject SQL via the AI’s input? Can they retrieve other users’ data by tricking the API? Also, validate the model on a variety of data including worst-case scenarios. The goal is to bulletproof the AI as much as possible.
From a governance perspective, security and robustness should be topics on your AI project checklist. Ask: Have we secured the training data (so no one can tamper with it)? Who has access to our models? Are we handling personal data safely while training (privacy enhancing techniques like anonymization or federated learning can help)? And, how does the AI perform under stress?
Incident response ties in here: despite best efforts, if an AI-related security breach or failure happens, have a response plan. If someone were to hack your pricing algorithm to give themselves ultra-low prices, you need to detect it (monitoring) and fix it swiftly (maybe rolling back transactions, patching the exploit, informing affected customers if needed).
In conclusion, think of AI systems like you think of any critical IT system: defend them and design them for resilience. In retail, losing customer trust due to a data breach or a glaring AI error can be costly. Many of the measures for AI security/robustness overlap with standard IT security, but they also include new AI-specific tests and safeguards. Governance is about making sure these steps aren’t skipped. By building security and robustness into your AI development lifecycle, you reduce downtime, prevent breaches, and ensure your AI reliably delivers value even in the face of surprises.
4.3 Incident Response and Continuous Improvement
Even with strong controls, incidents can happen – an AI might produce an unintended bad outcome or a new risk might emerge. How you respond can make a huge difference in limiting damage and learning lessons. Additionally, the world of AI and regulations is constantly evolving, so continuous improvement of your governance processes is essential.
Incident Response for AI: Treat AI incidents similarly to how you’d treat a product recall or a data breach – with urgency, transparency, and a focus on those affected. For example, say your AI-powered supplement recommendation tool inadvertently suggested a combination of supplements that could be unsafe if taken together. The moment this is discovered, an incident response might involve: immediately removing or fixing the faulty recommendation rule (technical remediation), checking if any customers followed that advice and reaching out to them with corrective information (customer care), and reviewing how the system allowed that recommendation (investigation).
An incident response plan for AI could include predefined steps:
Detection and Alert – How is the issue identified and who gets notified? (Maybe employees are encouraged to report anomalies, or you have automated monitors that flag unusual outputs.)
Containment – Can you suspend or rollback the AI function quickly? For instance, switching the site to a simpler recommendation list while investigating the AI’s error.
Impact Analysis – Determine who or what was affected. Did this error reach customers? How many? Over what time? This is where logs and records help.
External Communication – Decide if stakeholders or users need to be informed. It’s often better to be transparent if customers saw the error: e.g., send an apology email if customers got a misleading recommendation or if the chatbot gave some offensive answers due to a glitch.
Correction and Future Prevention – Fix the immediate issue, then do a root cause analysis to prevent it from happening again. Update processes: maybe your testing missed this scenario, so add it in the future; or maybe the AI lacked a certain constraint, so implement a new rule or oversight.
Documentation – Document the incident and the resolution. This record is valuable for governance audits and for training purposes.
Having an incident response plan means when something goes wrong, the team isn’t scrambling in confusion – they have a playbook. For critical AI systems, you might even do practice drills or “fire drills” for potential incidents. For instance, simulate what you’d do if the AI started giving bizarre product recommendations due to a data feed error.
Continuous Improvement: AI governance isn’t “set and forget.” You should periodically review how well your governance framework itself is working. Are the AI audits catching issues? Do staff feel comfortable raising concerns? Are there new best practices or tools we should adopt (for example, new bias mitigation techniques or updated guidelines from regulators)?
Set a schedule, maybe annually or semi-annually, to formally evaluate the AI governance program. This can be part of a larger compliance review. In that review, gather input: metrics like number of AI incidents, results of the latest bias audits, feedback from the committee on any challenges they faced, changes in law (like if since last year, the EU AI Act text finalized, you’d incorporate those new requirements).
Also, consider getting external input for improvement. Sometimes an external audit or consultancy (like HI-GTM, shameless plug) can assess your framework and suggest enhancements gleaned from industry developments. Or participating in industry forums about AI ethics can provide insights. For example, the retail industry might share non-competitive info on handling AI in supply chain or marketing in a responsible way.
As part of improvement, keep an eye on customer feedback. Customers might not say “your AI is biased” in so many words, but they will express dissatisfaction that could point to an AI governance issue (like “I keep seeing irrelevant products” or “this app feels creepy in how it targets me”). Use surveys or feedback channels to detect such sentiment and investigate if an AI system tweak is needed.
Employee feedback is equally valuable. Front-line employees using or overseeing AI might spot inefficiencies or risks. Maybe your customer service reps notice the AI chatbot struggles with certain queries – feeding that back allows you to retrain the chatbot and perhaps adjust your governance to include that scenario. Encourage a culture where AI is everyone’s responsibility, so improvements come from all corners. As the European Commission’s ethics guidelines say, AI systems should have mechanisms for feedback and redress, so ensure people know how to flag issues.
Finally, update training continuously. As policies change or new cases emerge, refresh employee knowledge. If you found that teams weren’t aware of a certain protocol during an incident, incorporate that learning. Learning culture is key: treat near-misses or small incidents as opportunities to fortify the system.
In essence, AI governance is a cycle – implement, monitor, respond, learn, refine. This continuous loop ensures that governance keeps pace with the fast-moving AI landscape and the growth of your business. Over time, a mature governance process will handle most issues smoothly as standard procedure, leaving your organization free to focus on innovating and serving customers, with confidence that the guardrails are solid.
One concrete example of continuous improvement: Walmart’s customer-centric commitment in their AI pledge is to measure customer satisfaction and “listen to feedback,” using it to continually review AI tools for accuracy and relevance.
That is a feedback loop in action – they aren’t just deploying AI and leaving it; they’re constantly tuning it based on what customers say. Your brand should do the same, making incremental improvements a routine part of your AI strategy.
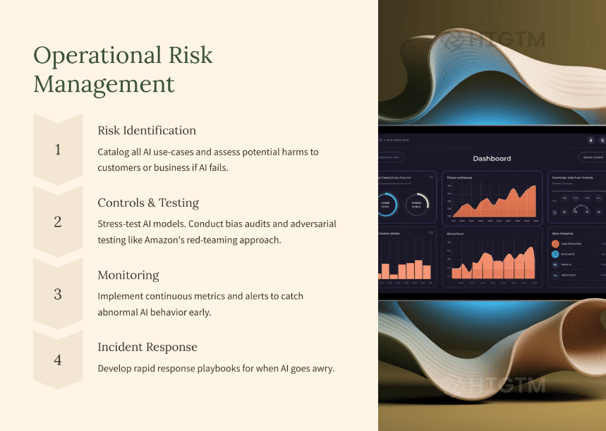
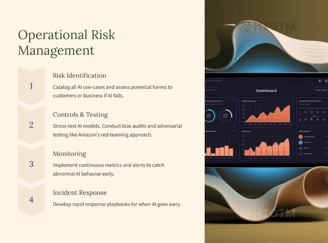
5. Global Industry Best Practices and Case Studies
5.1 Amazon’s Approach to Responsible AI
Amazon, as a pioneer in e-commerce and AI (with everything from product recommendations to Alexa), has developed robust practices to ensure AI is used responsibly. One hallmark of Amazon’s approach is its commitment to safe and secure AI development. In 2023, Amazon – along with other tech giants – made voluntary pledges to the White House to uphold certain AI governance standards.
These include performing internal and external red-team testing of AI models to probe for vulnerabilities or bias, sharing information on AI risks with other organizations, and enabling techniques to identify AI-generated content (like watermarking images or text). This shows Amazon’s recognition that transparency and collaboration are crucial at the cutting edge of AI.
Internally, Amazon integrates responsible AI checks at each stage of development. They have stated that they consider factors like accuracy, fairness, safety, and privacy during the design, development, and deployment of AI.
For example, before an AI feature goes live on Amazon’s retail platform, it would be scrutinized for potential bias or error impacts. Amazon Web Services (AWS) has even published principles and tools for customers to build responsibly – indicating the company’s philosophy of embedding governance into the process.
A practical example is Amazon’s tool SageMaker Clarify, which Amazon uses and offers to others. This tool helps detect biases in machine learning models and explain model predictions.
Within Amazon, deploying such tools means their developers can automatically check if, say, a product ranking algorithm is inadvertently skewing results. By leveraging technology for governance, Amazon can govern at scale across thousands of models.
Another example is Amazon’s CodeWhisperer (an AI coding assistant): Amazon built it with security in mind from the start – it has built-in scanning to avoid vulnerable code and even tracks open-source code usage to respect licenses.
This hints at a broader mindset where AI is not released into the wild without guardrails.
For a supplement brand, the takeaway from Amazon is: make AI responsibility part of the innovation DNA. Use automation to help with governance (like Amazon does with bias detection tools), and adopt a proactive stance on industry standards. Amazon’s willingness to publicly commit to AI safety standards sets a benchmark. It indicates that even the most advanced AI practitioners see governance not as a hindrance but as an enabler of trust and innovation. The result for Amazon has been relatively smooth sailing despite their heavy use of AI – they haven’t faced major scandals around Alexa or their recommendation engine in terms of ethics, largely because they work to prevent problems (aside from occasional critiques, which they respond to with improvements).
In implementing Amazon-like practices, consider establishing your own AI development guidelines akin to Amazon’s internal principles, and possibly utilize or adapt tools like Clarify for your models. If using AWS, you can directly employ their responsible AI services. Ensuring security (as Amazon does with watermarks and cybersecurity for models) is also key – for instance, if you provide an AI-driven feature, you might mark content as AI-generated for transparency.
Amazon also focuses on constant improvement by learning from customer interactions at scale. They likely use the massive feedback data to refine their algorithms relentlessly. Emulating that, use the data you gather (feedback, usage patterns) to refine your AI and keep it aligned with desired outcomes.
In summary, Amazon’s best practices revolve around embedding governance into the tech stack and corporate commitments. They treat responsible AI as part of their brand promise (much as they do fast shipping or customer obsession). And for any retail brand, that’s a powerful lesson: responsible AI use can become part of your value proposition, where customers and partners know that your AI is trustworthy because you’ve built it with care.
5.2 Walmart’s Responsible AI Commitments
Walmart, the world’s largest retailer, has taken a very public and principle-driven approach to AI governance. In October 2023, Walmart announced its Responsible AI Pledge, which centers on six commitments.
These commitments are noteworthy because they succinctly capture key governance themes in language for customers and associates alike:
Transparency: Walmart promises to help people (customers, members, employees) understand how it uses AI in the business and what the AI’s goals are
. For example, if Walmart uses AI to manage inventory or recommend products, they aim to be open about that usage. This might manifest as explanatory signage in stores or informational content online about their AI tools. It ensures trust through openness.
Security: The company commits to using advanced security measures to protect data and to continuously update those defenses against new threats. This acknowledges that AI is built on data, and keeping that data (and the AI systems) secure is paramount.
Privacy: Walmart pledges to use AI in ways that protect privacy, evaluating its systems to ensure sensitive information is handled properly. So, even if AI is scanning shopping patterns, it must do so in compliance with privacy standards, and personal data won’t be misused.
Fairness: They explicitly state they will evaluate AI tools for bias and mitigate potential bias, with regular re-evaluation. This aligns with what we discussed – making fairness a continuous process. Walmart knows its decisions can affect millions, so bias checks are essential to avoid discriminating in hiring, lending (they have financial services), or customer experiences.
Accountability: Walmart says it will use AI that is “managed by people” and holds themselves accountable for AI’s impacts. This is essentially the company saying: we won’t blame the algorithm; we take responsibility. Practically, it means human oversight on AI decisions and clear ownership of AI systems internally.
Customer-Centricity: They commit to measuring customer satisfaction with AI interactions and continually improving AI so it’s accurate, relevant, and truly helpful. This is about not losing sight of the end user – if an AI feature isn’t actually making customers happier, they will refine or even reconsider it.
What’s powerful about Walmart’s approach is how it ties AI ethics directly to their brand promise (“helping people live better”). They communicated these principles not just internally, but to the public, which adds pressure to uphold them but also earns trust. It’s a blueprint other retailers can follow: set clear principles and share them, so everyone knows the standards you’re working to meet.
In practice, Walmart’s governance likely includes things like an internal review board for AI projects (to enforce these principles), training for developers on these six points, and regular audits. The principle of customer-centricity suggests they actively gather feedback on AI features – for instance, after interacting with an AI kiosk or chatbot, customers might be surveyed, and those results feed back into AI refinement.
Another aspect is they link this pledge to phases of AI tech – meaning they consider these principles at every phase (design, implementation, use). So, when building a new AI tool, the design must incorporate transparency, privacy, etc., not just after deployment.
For your supplement brand, adopting a similar commitment framework can be very beneficial. You might declare your own set of AI principles – likely overlapping a lot with Walmart’s (they’re broadly applicable). What matters is making them more than words: each principle should tie to concrete actions or policies. For example, if you pledge “fairness,” specify that you will perform a bias audit on any customer-facing AI each quarter. If you pledge “privacy,” commit to an annual external privacy review of AI data usage or to never use certain sensitive data in AI without consent.
Walmart’s example shows that top-level commitment drives action on the ground. The pledge was signed off by leadership (their Chief Counsel for Digital Citizenship spearheaded it), indicating organizational buy-in. So ensure your leadership is on board and possibly even publicly supportive of AI governance efforts – it sets a tone that this is a core value, not a mere checkbox.
In summary, Walmart’s framework is a great checklist for any AI governance plan: be transparent, secure, respect privacy, ensure fairness, maintain human accountability, and focus on customer benefit. This encapsulates much of what we’ve discussed in simpler terms. They demonstrate that even as a retail behemoth adopting advanced AI (from shelf-scanning robots to online personalization), you can do so in a way that is principled and customer-first. And they expect it to be a differentiator – as their leadership noted, by leading in this space they hope to “pave the way for adoption of ethical AI in retail”.
Following their lead, your brand can also position itself as a responsible innovator, which can strengthen customer loyalty and partner relationships.
5.3 Alibaba’s Ethics Committee and Principles
Alibaba, operating in the massive e-commerce market of China, provides an insightful case of integrating governance in a fast-paced, highly competitive environment. In recent years, Alibaba recognized that to sustain long-term growth and public trust, it needed to systematically address AI ethics. Thus, Alibaba’s CTO established a Technology Ethics Committee, bringing together not just internal leaders from its research (DAMO Academy) and legal teams but also independent experts in technology, law, and philosophy.
This mix is powerful – it means their governance isn’t happening in an echo chamber; outside perspectives are included to challenge and guide the company’s approach.
The ethics committee’s mandate is to design and implement an AI ethical framework with regulations and accountability mechanisms focusing on issues like algorithmic governance and privacy.
One can think of this committee as both a rule-setting body and an advisory board. By positioning the CTO as the head, Alibaba signaled that ethics are a tech leadership issue, not just a legal formality.
Alibaba’s committee set out six core guiding principles for AI development
Human-centric: AI should benefit people and respect human dignity.
Inclusiveness and Integrity: AI should be inclusive, serving all groups, and uphold integrity (no malicious behavior).
Safety and Reliability: AI systems must be safe to use and reliable in performance.
Privacy Protection: Strong data protection and user privacy must be ensured.
Trustable and Accountable: AI decisions should be trustworthy, with mechanisms to hold systems (and creators) accountable for outcomes.
Openness and Collaboration: Emphasizes sharing best practices and collaborating on AI governance (perhaps acknowledging that ethics is an ecosystem effort).
These principles closely mirror global AI ethics guidelines (like the EU’s Trustworthy AI’s 7 requirements), showing Alibaba aligned with international thinking while presumably tailoring it to their context.
What’s compelling is how Alibaba put principles into practice specifically to tackle known problems in e-commerce. We mentioned earlier the Matthew Effect issue: Alibaba noticed their recommender was amplifying the advantage of big merchants.
They responded with solutions driven by the principle of fairness/inclusivity. They built features to highlight new products, and used multi-modal AI to identify promising new items to give them a fighting chance.
This is a concrete ethical intervention – sacrificing a bit of short-term click optimization to ensure a more level playing field on their platform. It aligns with long-term platform health and fairness.
Another practice: Alibaba has privacy protection champions in each business unit.
For example, they implemented a system where when customers and couriers communicate on Taobao (their platform), the phone numbers are masked by a private number relay. This came from an ethic of privacy – even something as small as a buyer contacting a seller is protected so personal info isn’t exposed. That kind of design consideration stems from having privacy principles and accountability (teams tasked to enact them).
Additionally, Alibaba’s emphasis on explainable AI is notable. They recognized deep learning is a “black box” and invested in explainability research to allow human intervention. Explainable AI helps their engineers fine-tune systems and also helps in communicating decisions if needed.
For your brand, Alibaba’s case shows the value of a formal governance body (even if small scale) and the importance of tailoring ethical principles to real business scenarios. The composition of their committee is something to emulate at a scale that fits your company. Perhaps you have an advisory relationship with an academic or an industry expert who can give perspective on your AI uses. Including such voices can enhance credibility and foresight.
Also, Alibaba’s approach underlines continuous innovation in governance. They are investing in tech solutions (like algorithmic fairness, privacy tech) to solve ethical challenges rather than seeing ethics as purely a restriction. You too can look for win-win innovations: maybe an AI feature that not only abides by rules, but also differentiates you. (E.g., “shop with confidence – our AI is designed to be fair to all sellers and transparent with customers,” could be a selling point in a marketplace.)
Alibaba operates in a regulatory environment where the government has its own stringent views (China has issued AI ethics guidelines nationally). Alibaba’s proactive stance likely helps shape or at least meet those coming rules, similar to how aligning with EU guidelines prepares companies for the AI Act. It shows that regardless of region, core principles of good AI governance converge: fairness, transparency, privacy, accountability.
In essence, Alibaba demonstrates that ethical AI and business success are compatible – even necessary. By addressing fairness, they keep their platform healthy and diverse. By focusing on privacy, they protect user trust which is crucial for e-commerce. A supplement brand might not have the same scale, but the lessons scale down: have a clear set of principles, involve broad expertise, and translate those principles into actionable solutions in your daily operations (whether that’s adjusting an algorithm or adding a privacy feature).
5.4 Collaborative Frameworks and Industry Initiatives
No company operates in a vacuum, especially when it comes to setting standards for AI. Collaboration and following industry frameworks can bolster your governance strategy significantly. It not only provides guidance and resources but also shows regulators and consumers that you are aligning with accepted best practices.
One key collaborative effort in retail is the National Retail Federation (NRF) in the U.S. which released Principles for the Use of AI in Retail. These principles, developed through the NRF’s Center for Digital Risk & Innovation, offer a sector-specific perspective. They cover four broad areas :
Governance and Risk Management – urging retailers to have strong internal AI governance as the foundation for managing risks and ensuring AI delivers value (exactly what we’re building).
Customer Engagement and Trust – urging transparency about AI use, safeguards against discrimination, and alignment of AI with existing privacy/cybersecurity policies. This resonates with our compliance and ethics focus.
Workforce Applications and Impact – ensuring oversight of AI that affects employees (like HR or scheduling algorithms) and involving employees in AI use.
Business Partner Accountability – making sure your vendors or partners who provide AI tools or data also meet your governance standards. This is crucial – if you use a third-party AI service, you should vet it for compliance and ethics, as their issues can become your issues.
By following the NRF guidelines, you essentially get a checklist tuned to retail concerns. It also harmonizes with what giants like Walmart are doing (no coincidence, Walmart’s pledge aligns well with NRF’s framework, and the NRF noted Walmart’s pledge in their commentary).
Adopting such guidelines can future-proof you because they often anticipate regulatory trends. For instance, NRF’s emphasis on preventing bias against protected classes in AI aligns with what any anti-discrimination law would require.
On the global stage, there are the OECD AI Principles (backed by 40+ countries, including the US, EU, etc.), which cover transparency, fairness, safety, accountability – all principles we’ve integrated. There’s also the Global Partnership on AI (GPAI) and other multi-stakeholder groups that produce research on responsible AI. While as a smaller brand you might not directly engage with them, their reports and tools can be very useful resources for improving governance.
Additionally, companies sometimes band together to form informal information-sharing groups on AI ethics. For example, some might share anonymized incident reports or best practices at industry conferences. Staying plugged into these conversations (via webinars, industry meetups) means you learn without stepping on landmines yourself. It’s the idea of collective learning – if another retailer discovered an issue with an AI tool (say, a vision AI had trouble recognizing certain skin tones when advertising cosmetics), knowing that can help you check your own systems proactively.
Another collaborative front is academic partnerships. Retail and AI intersect in fields that universities study (marketing algorithms, data privacy, etc.). Collaborating on research or pilot projects with academic labs can bring cutting-edge governance ideas to your company. It could be as simple as sponsoring a study on bias in supplement recommendations or participating in a university’s AI ethics challenge with case studies.
Finally, engaging with regulators and standard bodies as they formulate rules can be smart. Large companies often lobby or provide feedback on proposed AI regulations – smaller ones can still make their voice heard through industry associations. Being aware of and contributing to these discussions ensures your governance efforts are aligned with what’s coming and can even influence rules to be practical and innovation-friendly.
In practice, to leverage these frameworks and initiatives:
Download and review the NRF AI principles document, and map your governance policies to it to see if there are gaps.
Adopt language from these frameworks in your own policy documents – it shows alignment. For example, explicitly include “no unlawful discrimination” as a policy for any customer-facing AI, echoing NRF’s point.
Ask your AI vendors about their ethics practices (a simple questionnaire can filter out serious providers who follow standards vs. those who don’t consider them). This covers partner accountability.
Consider signing on to relevant pledges or initiatives if available for your company size. It could be as straightforward as publicly endorsing a principle like “We support the ethical use of AI in retail as outlined by XYZ”.
The benefit of collaboration is credibility and shared strength. It’s reassuring to customers and partners if you can say “Our approach follows guidelines set by the National Retail Federation and global best practices.” It also means you’re less likely to miss important aspects of governance, since these frameworks are developed by diverse experts.
In sum, tap into the collective wisdom. AI governance is a new field; no one has all the answers alone. By aligning with industry and global frameworks, you not only strengthen your program but also contribute to a higher standard in the retail industry. As more brands do this, it elevates the entire sector’s trustworthiness, benefiting everyone.
6. Implementing an AI Governance Framework in Your Organization
6.1 Establish Governance Roles and Teams
The first step in formalizing AI governance is assigning clear ownership. Decide who will be responsible for AI governance in your organization. Depending on your size, this could be an individual or a committee.
For many companies, creating a cross-functional AI governance committee is effective. This committee should include representatives from key areas: IT/data science (for technical insight), legal/compliance (for regulatory perspective), operations or product (who understand how AI is applied in the business), and possibly an executive sponsor (to give it weight). The committee’s mandate is to develop and enforce AI policies, review major AI-related decisions, and serve as the internal authority on AI ethics and risk.
If resources are limited, you might designate an existing leader to wear an “AI Governance hat”. For example, the Chief Technology Officer or Head of Data could take on this role, or a Chief Compliance Officer with tech interest. What’s important is that this person or group has the power to halt or modify AI projects that don’t meet standards and the ear of top leadership to escalate concerns.
Define clear roles within the governance structure. Some possible roles include:
AI Ethics Officer or Lead: Point person coordinating governance efforts, perhaps chairing the committee.
Data Privacy Officer (DPO): If you have one for GDPR compliance, ensure they’re involved in AI projects too (GDPR mandates this integration to some extent).
Model Risk Manager: In banks, they have model risk management; a similar concept in retail could be someone who inventories and tracks risk levels of each AI model. This might be part of an analytics governance team.
Business Unit Liaisons: People in each department using AI who liaise with the central committee (like how Alibaba has privacy/security contacts in each unit).
Also consider an external advisor if feasible. An external expert (perhaps on a retainer or periodic review basis) can provide impartial audits or advice on tricky cases. They might be a professor in AI ethics or a consultant specialized in AI risk.
Once roles are set, define processes: e.g., the AI governance committee will meet quarterly (or more often initially) to review all ongoing AI projects, evaluate any new high-impact AI proposals, and discuss any incidents or emerging issues. They might produce a brief report for senior management summarizing status and any decisions.
It’s crucial to get buy-in from the top. If the CEO or board publicly backs the governance initiative (“we are establishing an AI oversight committee to ensure our AI is trustworthy and in line with our values”), it sends a message to all employees that this is serious. It empowers the committee to enforce rules even if it means slowing down a project for additional checks.
Empowering roles also means giving them resources – time, training, tools. Members of the governance team should be allowed to allocate part of their schedule to these duties. If needed, provide training on AI ethics or send them to relevant workshops so they can stay informed. This is a new responsibility, and you want them to be well-equipped.
A simple but effective practice is to integrate AI governance into the project lifecycle. For example, require that any new AI initiative (say, developing a new algorithm for upselling products) include an “AI Governance Impact Assessment” as part of its project kickoff. The governance lead could co-author this with the project manager, identifying expected data use, potential risks, etc., and then sign-off from the governance committee is needed before full deployment. This way, governance isn’t an afterthought; it’s baked in from the get-go.
Finally, clarify accountability: When an AI-related decision is made (like “go live with this model” or “alter this algorithm due to bias”), who is accountable for that decision? It might be a joint accountability of the committee and the business owner of the AI. State that clearly, so if something goes wrong, it’s addressed constructively and not a blame game. The accountable parties then also handle remediation and communication.
In summary, setting up governance roles and teams gives structure to your AI oversight. It ensures there are dedicated “watchdogs” and facilitators for responsible AI. This organizational step is foundational – without clear responsibility, even the best policies can fall through the cracks. With the right people at the helm, your AI governance plan has champions to drive it forward and stewards to maintain it over time.
6.2 Develop Clear Policies and Training Programs
With roles in place, the next step is to establish the rules of the road for AI – in the form of policies, guidelines, and procedures – and to educate your team about them. Policies translate high-level principles (like fairness, transparency) into concrete expectations and requirements that employees and AI systems must follow.
Start by drafting an AI Ethics and Governance Policy document. This might include sections such as:
Purpose and Scope: e.g., “This policy applies to all AI and automated systems used in XYZ Company’s operations or products.”
Principles: list the key principles (you can borrow those we’ve discussed: compliance with laws, fairness, transparency, privacy, security, accountability, etc., possibly even copy Walmart’s or Alibaba’s with your own wording).
Operational Requirements: For each principle, what actions are required? For example, under fairness: “All AI models that influence customer outcomes shall be tested for potential bias across key demographics. Mitigation plans must be implemented for any identified bias.” Under transparency: “Customers will be informed when they are interacting with an AI system and given access to an explanation of AI-driven decisions wherever feasible.” Under privacy: “AI systems must only use data in accordance with our privacy policy and any applicable regulations (GDPR, CCPA); personal data used in AI must be minimal and, when possible, anonymized.”
Review and Approval Process: Explain that any new AI initiative should go through the governance committee or an AI risk assessment before full deployment. Perhaps require a checklist to be completed and attached to project documentation.
Monitoring and Auditing: State that AI systems will be monitored and periodically audited for compliance with these policies, and describe responsibilities (like “Data Science team will generate quarterly bias reports for each algorithm which the AI committee will review”).
Incident Handling: Outline steps to take if an AI-related issue is discovered (stop model, notify governance lead, etc.), referencing the incident response plan.
Third-Party AI Policy: If using vendors, require due diligence on their practices. For example: “Partners providing AI services must agree to meet our standards on data handling and nondiscrimination” (NRF’s partner accountability principle can guide this).
Once drafted, vet this policy with relevant stakeholders, then get it formally approved by senior management. Publish it internally (and externally if you want to showcase your commitment). Make sure it’s written in accessible language, not just legalese, so employees really grasp it.
After policy comes training. You need to bring the policy to life for your employees. Conduct training sessions tailored to different teams:
For your technical teams (data scientists, developers): focus on how to implement these policies in their work. Teach them about bias testing methods, privacy-preserving techniques, secure coding for AI, etc. Many tech folks haven’t been formally trained in ethics, so provide concrete examples and tools. Perhaps introduce them to fairness toolkits or explainable AI libraries they can use.
For your marketing, product, and sales teams: help them understand transparency and honesty in AI-driven communications. For example, train customer service reps on how to explain an AI decision to a customer if needed. Train marketing on avoiding over-reliance on AI-generated content that could be inaccurate.
For compliance/legal teams: ensure they understand AI enough to assess compliance. Perhaps do cross-training where tech and legal sit together to discuss how to do a DPIA for an AI project.
For executives and managers: give them a high-level overview of what AI governance means and their role in championing it. Maybe run through a scenario with them – e.g., how to respond if an AI issue goes public – to illustrate the importance of support from the top.
To make training engaging, use real or realistic case studies. For instance, craft a scenario about an AI recommending a supplement in a way that might be construed as a medical claim, and walk through with the team how to identify and adjust that. Or show anonymized results of a bias test on your data (if you found any anomalies during development, those make great teaching material).
Interactive workshops are more effective than dry lectures.
Also, incorporate AI ethics and compliance into onboarding for new employees, especially those in roles that will touch AI. This ensures from day one they know your company cares about this.
Supplement training with resources: an internal wiki or toolkit with guidelines like “Checklist before deploying an AI model” and links to tools or contacts (e.g., “contact the AI governance lead for a bias check template”). Posters or quick-reference cards with key principles can also keep awareness up (for example, a small poster by the data science area: “Think F.A.I.R. – Fair, Accountable, Interpretable, Responsible – in every model you build!”).
Encourage open discussion and questions during training. Some employees might fear that governance slows them down; address that by emphasizing long-term benefits and showing support (like tools and help from the governance team) to make it manageable.
Finally, record and track who has undergone training. For high-risk roles, you might require annual refresher courses (similar to how companies do with sexual harassment or safety training). Keeping everyone up-to-date is part of demonstrating due diligence.
In short, policy sets the expectations, training ensures understanding and capability. They go hand-in-hand. A policy sitting on a shelf won’t change anything; it’s the training and daily practice that make governance real. By educating your workforce, you distribute the responsibility and create a culture where people are mindful of AI’s impacts. This investment in people is what truly operationalizes your AI governance framework.
6.3 Integrate Ethics and Compliance into the AI Lifecycle
To be truly effective, AI governance must be woven directly into the lifecycle of AI development and usage – from the initial idea for an algorithm to its retirement. This means that at each phase (design, development, testing, deployment, and maintenance), there are checkpoints or practices ensuring compliance and ethics are addressed, not as an afterthought but as a core part of building the system.
Design Phase: When planning a new AI application, start with ethical and legal considerations. A useful practice is to conduct an Ethical Impact Assessment or AI Canvas at the concept stage. Ask questions: What is the purpose of this AI? Who could be affected and how? What data will it use – is that data sensitive or personal? What are the potential benefits and potential harms? By brainstorming these, you may identify right away if the project needs certain constraints (e.g., “this AI will offer supplement advice – we must ensure it doesn’t cross into medical advice territory”).
Also, involve diverse voices in design. If you have a team with varied backgrounds, they can point out different perspectives (for example, someone might say, “This personalization algorithm might consistently push weight-loss supplements to a certain demographic; how will that be perceived?”). Diversity can catch biases early.
Development Phase: As data is collected and models are built, enforce standards for data quality and privacy. Ensure data used to train AI is representative and as unbiased as possible. For a supplement brand, if you’re training on past sales data, consider if that data itself was biased by marketing only to certain groups; you might augment it to balance perspectives. If using personal data, go through proper privacy vetting – is all data obtained with consent and necessary for the task? Perhaps use techniques like data anonymization or aggregation to reduce privacy risks. If the AI doesn’t need names or IDs, don’t include them in the training set.
Adopt a “privacy by design” approach: for example, if building a feature that recommends supplements based on health quiz responses, maybe design it so that sensitive health info is processed on the user’s device (edge computing) so raw data isn’t stored on your servers, only the insights.
During coding, follow security best practices to avoid vulnerabilities. Use version control and documentation not just for code but for datasets and model parameters, so you have a clear record (this helps accountability and auditing).
Testing Phase: This is where heavy governance checks happen. We have discussed bias testing – implement that now. Evaluate the model’s decisions across categories (gender, age, location, etc., as appropriate) to spot unwanted bias. If biases are found, refine the model or add corrective measures (like adjusted thresholds or additional training data). Conduct a privacy impact assessment if personal data is involved: ensure that the AI isn’t inadvertently exposing info (for instance, if you have an AI that generates reports, make sure it doesn’t spit out individual customer data in a way it shouldn’t).
Also test explainability: use tools to see on what factors the AI is relying. If your product recommendation AI is basing suggestions on something odd (like always recommending a product that was simply on top of a list due to coding quirk), you catch it here. Or you might find the AI places too much weight on one feature (like customer age) which could be a bias proxy.
Perform adversarial testing too: try to break the AI or confuse it. For example, input weird or borderline inputs to a chatbot to see if it says something it shouldn’t. If it does, tweak the chatbot’s training or rules.
Crucially, involve the governance committee or at least the compliance person in the testing review. Before deployment, have a go/no-go checklist that the AI governance lead signs off. That checklist might include items like: “Bias audit completed and acceptable,” “Security review passed,” “Privacy approval obtained,” “Output explanations verified,” etc. If any item is “No,” you don’t launch until resolved.
Deployment Phase: When you deploy the AI into production, implement controls and transparency measures. For example, enable logging of AI decisions (keeping user identities protected) so you have an audit trail. If applicable, deploy with a gradual rollout – maybe test the AI on a small percentage of users and monitor results, before full-scale. This can catch unanticipated issues in the real world without affecting everyone.
Ensure user interfaces incorporate transparency: labeling chatbot interactions, giving opt-outs if legally required (like if content is AI-generated, maybe mark it; or in EU, if a user requests not to be profiled by AI, honor that).
Also, consider setting usage boundaries: like a recommendation AI should not recommend beyond a certain number of items per day per user (to avoid spamming or manipulation). Or a dynamic pricing AI should have caps (not raising prices above X% in a day to avoid gouging scenarios).
Maintenance Phase: Once running, keep feeding back into the governance loop. As discussed, monitor metrics, get user feedback, and periodically retrain models. Apply patches as needed for new threats (like if a vulnerability is found, update immediately).
Additionally, maintain a practice of model review at set intervals. For instance, every 6 months, revisit the model’s assumptions and performance. Document any changes (if you adjust the algorithm, note why – this documentation habit is akin to documenting changes in critical processes, good for accountability).
Plan for sunsetting or major changes responsibly. If you decide to replace an AI model with a new approach, treat it like a new project and assess it. If you discontinue an AI feature because it wasn’t working ethically or business-wise, ensure any data is properly archived or deleted as per policy, and inform users if necessary (“We’ve phased out automated health tips to focus on personalized expert content” – transparency even in removal).
Throughout the lifecycle, engage stakeholders: keep leadership informed at key milestones (design sign-off, pre-deployment sign-off, etc.), and also perhaps communicate to customers what you’re doing (for trust-building, some companies publish blog posts “How we’re using AI to improve your experience – and doing it responsibly”).
By integrating ethics and compliance steps in each phase, you transform governance from a static set of rules into a living practice that accompanies the AI from birth to retirement. This reduces friction because teams won’t feel a big “governance shock” at the end; they’ll be doing the right things all along. It also catches issues early when they’re easier to fix (fixing a bias is much simpler before a system is live and entrenched).
In essence, ethics by design should be as natural as security by design or quality by design. When it’s part of the lifecycle, the resulting AI systems are not only more trustworthy but likely better overall – often, ethical improvements (like more diverse training data) can also make models more robust and accurate for a broader user base.
6.4 Monitor, Audit, and Adapt the Framework
Implementing the framework is a big achievement, but governance is never a one-and-done task. The AI landscape evolves (new technologies, new uses), and so do regulations and societal expectations. Thus, continuous monitoring, auditing, and adaptation of both your AI systems and the governance framework itself is essential to keep everything effective and up-to-date.
Monitor AI Systems Regularly: As covered earlier, you should have monitoring in place for each AI application – tracking performance metrics (accuracy, error rates, business KPIs), fairness metrics, and any incidents or anomalies. If your recommendation engine’s performance dips or starts showing skewed results, you want to know ASAP. If customers are increasingly contacting support after an AI interaction (maybe confused or dissatisfied by it), that’s a signal. Develop a dashboard for AI oversight if possible, where governance folks can see key metrics at a glance.
Some companies establish an AI incident log or register. Encourage teams to log any AI-related issues or near-misses, however small. For example: “Jan 10 – Chatbot misunderstood a customer question about allergies, gave an irrelevant answer; customer was confused.” These logs, while low-level, can be analyzed for patterns. Perhaps you find that the chatbot frequently flubs medical-related queries – that could lead to a retraining with more medical intent data, or a policy to hand off such queries to a human.
Periodic Audits: At set intervals (quarterly, biannually, or annually depending on how fast things change), conduct a formal audit of major AI systems and governance processes. Auditing AI systems involves checking if they are still compliant with policy: Are we still meeting GDPR obligations? Did any new data creep in that shouldn’t be there? Is the model’s bias within acceptable range? One could re-run bias tests on the latest data, check that all required user consents are documented, verify that explanation systems are working, etc.
Also audit usage logs to see if there were any unusual patterns, and ensure that any user opt-out requests were honored by the AI (e.g., if someone opted out of personalized recommendations, confirm the system excluded them properly).
Audit the governance process too. For example, review a sample of AI project documents to see if they all went through the required approvals. If you find a project that launched without the committee’s knowledge, investigate why – maybe the policy wasn’t communicated well to that team, so you’d adapt by re-educating or tightening controls.
Consider bringing in a neutral party for audits occasionally – it could be an internal audit team (if separate from the AI team) or external consultants to review your adherence. They might spot biases or risks you overlooked. External audits also build trust if you ever choose to publicize that you undergo them.
Adaptation based on Audits and Feedback: Use the findings from monitoring and audits to improve. If an audit finds that a certain metric is drifting, adjust the model or process. If employees continuously bypass a certain governance step because it’s cumbersome, perhaps refine that step to be more efficient or provide a better tool.
Stay informed about new regulations and standards. For instance, if (when) the EU AI Act goes into effect, you might need to implement new procedures like notifying an EU authority about your high-risk AI or adjusting transparency measures. If a new ISO standard for AI management gets released, see if adopting it (or parts of it) would strengthen your framework.
Likewise, adapt to technological changes. Suppose you start using a new type of AI, like more advanced deep learning or external AI APIs (like an external GPT-like service to generate content). Each new tech might bring new risks – your governance framework should extend to cover them. For example, using generative AI to create product descriptions might require a new policy clause about fact-checking AI-generated content to ensure it’s accurate and doesn’t make unlawful claims (since generative models can fabricate information).
Feedback loops: Keep communication channels open. Gather feedback from the teams that have to implement governance. Are the tools and checklists working for them? Maybe the data scientists have suggestions to refine the fairness evaluation process. Or the marketing team might say the transparency notice you provided is too jargon-y for customers – then you’d simplify it. Engage with customers indirectly by observing their behavior and feedback. If customers seem satisfied and few complaints come in, that’s a good sign; if complaints about, say, “creepy” recommendations or privacy concerns pop up, that’s a red flag to act on.
Update Training and Policies as Needed: If your audits or external changes reveal gaps, update your policy documents. Version your policies and keep a record of changes and why. For example, “Policy updated March 2025 to include guidelines on generative AI usage and content verification.” Retrain staff on new or changed policies so everyone stays aligned.
Also, celebrate successes and improvements. If your audits show biases reduced from last year or zero major incidents in a period, share that internally. It reinforces the value of governance and motivates teams to keep it up.
Scenario Planning: Occasionally revisit worst-case scenarios: what if a major AI failure happened tomorrow? Are we prepared? Running tabletop exercises with the governance committee for new hypothetical challenges (like “A new law bans a practice our AI uses – how do we adapt?” or “Our AI pricing was accused of being discriminatory on social media – how do we respond?”) can reveal if your framework needs any tweaks or additions, in a low-stakes environment.
Continuous Improvement Philosophy: Ultimately, treat AI governance as a continuous improvement process akin to lean or Six Sigma processes. You measure, you learn, you improve. Over time, you might find the governance tasks that were once manual can be partly automated as well (for instance, automating bias checks in the ML pipeline so they run every time you retrain a model, sending a report to the committee).
By continuously monitoring, auditing, and adapting, your governance framework remains resilient and relevant. This agility means you can handle new challenges gracefully and maintain stakeholder trust. It also engrains a culture of vigilance around AI – everyone knows that it’s not set-and-forget, but a living responsibility. That cultural aspect is perhaps the greatest strength of a well-maintained governance program: it becomes part of “how we do things here”, evolving with the company and external world.
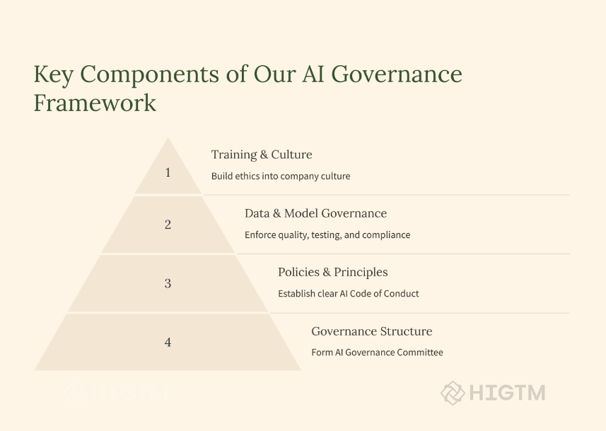
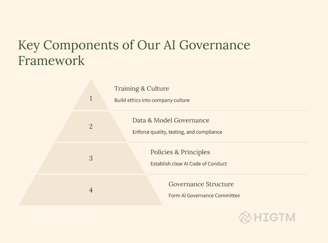
7. Benefits of Proactive AI Governance
7.1 Ensuring Compliance and Avoiding Penalties
One of the most immediate benefits of a strong AI governance framework is regulatory compliance, which in turn means avoiding costly fines, legal battles, and operational disruptions. By design, your framework addresses laws like GDPR, CCPA, and forthcoming regulations (EU AI Act, etc.), so you substantially reduce the risk of violations.
Consider data privacy: a slip-up with customer data can result in multi-million dollar fines under GDPR, not to mention lawsuits or compensation claims. By handling data carefully in AI projects (obtaining consent, limiting use, securing info), you’re less likely to suffer a breach or misuse that lands you in legal hot water. Think of governance as an insurance policy – it might require upfront effort, but it shields the company from far bigger damages down the line.
Moreover, compliance is not just about fines; it’s about business continuity. If you were found using an AI system unlawfully, regulators might force you to halt it immediately. For a supplement e-commerce brand, imagine if you had to suddenly shut off your recommendation engine or your automated marketing because of non-compliance – sales and customer experience could take a hit. Proactive governance prevents such scenarios by making sure everything you deploy is above board.
We’re also on the cusp of new rules like the EU AI Act which could impose penalties or even product bans for non-compliant AI. Getting your governance in shape now is future-proofing. When the AI Act comes, for instance, you may already be doing much of what it requires (like documenting your AI systems’ design and having risk controls) thanks to your framework. So you won’t scramble to comply – you’ll be largely ready, giving you a competitive edge over those who lag and possibly have to pull products or invest heavily to catch up.
Another aspect is improved risk management. A governed approach means you are systematically identifying and mitigating risks early, which reduces the chance of disasters. Fewer disasters means fewer crisis management costs, fewer refunds or legal settlements to customers, and stable operations.
Some companies have also found that proactive compliance can reduce their insurance costs or ease due diligence processes. For example, if you seek cyber insurance or if an investor evaluates your company, being able to demonstrate a robust AI governance program (with compliance records, audit trails, etc.) can make you a safer bet.
In sum, governance saves money and headaches by keeping you on the right side of the law. It’s hard to quantify a disaster that didn’t happen, but those who’ve been through regulatory penalties can attest to the massive distraction and expense they cause. By avoiding those, you keep resources focused on positive business activities.
7.2 Building Customer Trust and Brand Value
In the retail supplement industry, trust is everything. Customers ingest the products you sell – they must trust not only the products, but the recommendations and information you provide. A robust AI governance framework significantly bolsters that trust in the digital realm.
When customers know (or sense) that your AI-driven features are fair, transparent, and respect their privacy, they feel safer engaging with them. Trust can be built explicitly – for example, if you communicate your commitments (like Walmart did with their pledge, or via a privacy notice that clearly says “we use AI, but we never sell your personal data and you can opt out anytime”). Trust also builds implicitly over time through positive experiences: if your AI consistently gives helpful suggestions without ever crossing creepy lines or making them feel profiled, customers will grow comfortable with it.
Consider personalization: poorly done, it can scare customers (“How did they know that about me?”). Rightly done, it feels like good customer service (“They really get what I need!”). Governance ensures personalization remains on the right side. For instance, maybe your framework prevents using highly sensitive data or ensures messaging stays appropriate. The result is customers appreciating the convenience and relevance of AI features without the ick factor.
Brand differentiation is another benefit. As awareness of AI issues grows, consumers might start favoring companies that are ethically responsible with AI. Your brand can leverage this: “Our supplement recommendations are powered by AI with a human touch – carefully governed to be unbiased and privacy-safe.” It’s a selling point. Much like organic or fair-trade became market differentiators, “AI ethics” could well be something consumers care about. Being ahead on governance positions you as a leader, a brand that customers can trust not just for quality products but for respecting them in all interactions.
Trust also feeds into customer loyalty. If a customer trusts your use of AI, they’re more likely to engage more deeply – share data with you (like filling out a wellness quiz honestly because they trust you’ll use it carefully), or try new features (like an AI coaching chatbot, if they believe it’s well-designed and secure). That gives you more opportunity to serve them better, creating a virtuous circle of personalization and loyalty.
On the flip side, lack of governance can shatter trust quickly. One bad headline about a privacy breach or an AI bias incident can alienate customers, especially in health-related retail. People might fear using your site or app if they think your algorithms are manipulative or discriminatory. By avoiding negative incidents through governance, you avoid eroding the trust equity you’ve built.
There’s also a broader brand value angle: reputation and PR. Companies known for ethical practices often enjoy better public sentiment, which can translate into customer preference and even allow premium pricing. If your brand becomes known as “the honest, customer-first innovator” in e-commerce, that’s a huge intangible asset. AI governance contributes by showing you innovate (you use AI) but responsibly (you govern it well).
Additionally, good governance might improve the quality of AI-driven interactions, because often what is ethical – like being fair and transparent – also correlates with what customers perceive as positive. For example, fairness in an AI means it’s not narrowing options in a way that might inadvertently exclude items a customer could like; thus the customer sees more varied, interesting suggestions. Transparency in an AI (like “Why this product for me?” explanations) might educate the customer and build engagement.
In the supplements area, trust is already a delicate matter (people worry about product efficacy, safety, truth of claims). Extending that trust to your digital advice and marketing via AI can set you apart as a truly reliable partner in their health journey, not just a seller. That fosters deeper relationships, possibly turning customers into advocates for your brand.
7.3 Fostering Sustainable Innovation and Competitive Advantage
There’s a misconception that ethics and compliance slow down innovation. In truth, a solid governance framework can enable faster and more ambitious innovation because it creates a stable, secure environment to experiment. When you know you have guardrails, you can drive faster.
By addressing risks proactively, you avoid the fear or indecision that can paralyze teams (“Can we do this or will legal freak out?”). Instead, the team has guidelines and can iterate within them. This encourages them to try new AI features – they know the checklist of what to consider, so they feel confident in pushing boundaries while staying safe. It’s like having good brakes and seatbelts: you’re more willing to drive on the racetrack.
Moreover, if an innovation does raise new ethical questions, your governance body is there as a resource to resolve them. This means novel ideas won’t be killed just because they’re novel; they’ll be evaluated and, if possible, allowed with modifications. That agility is a competitive advantage. Companies without governance might shy away from certain AI uses entirely out of fear of unknown risks, or they charge ahead recklessly and later have to pull back (wasting effort) because they hit a scandal or regulator block. You strike the balance: thoughtful yet bold.
A good governance track record can also attract better partnerships and opportunities. Other businesses (like corporate clients, platforms, or even suppliers) might choose to work with a retailer known for responsibility, because it lowers their risk too. For example, if you were to get into an AI-driven partnership with a health organization (maybe to provide supplement recommendations on their wellness platform), they’ll vet your practices. Having robust governance could be the deciding factor that wins you the deal.
Internally, the culture of responsibility can also attract talent. Many tech professionals, especially younger ones, care about the ethical implications of their work. If your brand is known for taking AI ethics seriously, you might lure mission-driven tech talent who want to innovate without compromising values. That’s a talent advantage.
Sustainable innovation also means long-term viability of AI solutions. Systems built with governance in mind are less likely to face backlash or removal. They can keep evolving and improving. Each iteration of an AI can be refined with feedback and remain aligned with user expectations and norms. This continuity means your AI investments pay off more over time. You’re not rebuilding from scratch after a catastrophe; you’re iterating on a strong foundation.
Think of AI governance as building customer-centric discipline into tech innovation. When innovation is aligned with customer values (privacy, fairness, etc.), it’s more likely to succeed commercially. You might find that focusing on these aspects leads you to develop features that competitors overlooked but that consumers appreciate.
Additionally, being a frontrunner in responsible AI could shape industry standards. If competitors scramble later to meet regulations you already comply with, you have a head start and can even help shape the rules in your favor (being seen as a model company).
In the supplements arena, innovation might include AI nutrition advisors, personalized supplement regimens using machine learning, community AI moderators for forums, etc. With governance, you can implement these confidently and do so earlier than others, because you manage the risks. Early mover advantage with responsible innovation could capture market segments first and set the benchmark others have to match.
Finally, consider resilience: businesses that integrate governance are more resilient to external shocks. For instance, if a big AI scandal hits the industry (like say a competitor’s AI is exposed for bias, causing public distrust of AI in retail), your company can stand out as the reassuring exception due to your track record. You won’t need to pause innovation; you can double down and say, “We ensure our AI treats everyone fairly – here’s how.” You turn what could have been an industry setback into an opportunity to gain trust and market share.
In summary, responsible AI is smart business strategy. It reduces friction with regulators and customers, meaning you can innovate with fewer roadblocks. It aligns tech progress with human values, which is key to any tech’s adoption. And it differentiates you in a crowded market. By embedding governance, you aren’t just preventing negatives; you’re actively cultivating positives – better products, stronger relationships, and a brand that stands the test of time as technology evolves.
8. Conclusion: AI in e-commerce presents both remarkable opportunities
By establishing clear governance structures, developing strong policies, and weaving ethical checks into every stage of AI development, a company can confidently leverage AI to enhance customer experiences and operational efficiency without compromising on trust or values.
Global leaders like Amazon, Walmart, and Alibaba have demonstrated that taking AI governance seriously pays dividends in customer trust and sustainable innovation. They provide real-world blueprints that any brand, regardless of size, can adapt. Importantly, adhering to regulations like GDPR, CCPA, and the forthcoming EU AI Act isn’t viewed as a hurdle, but as a foundation for building consumer confidence and avoiding costly missteps.
For an e-commerce supplement brand, where health and personal well-being are at stake, the bar for trust is especially high. Implementing the strategies discussed – from bias audits to transparent AI interactions and rigorous security – will help ensure that your use of AI is part of what strengthens your brand’s reputation for quality and care. Customers will feel safer sharing their data and following your AI-driven recommendations, knowing you have their best interests safeguarded.
In closing, effective AI governance is a journey, not a one-time project. It requires commitment from the top, cross-team collaboration, and a willingness to continuously learn and improve. But the rewards are clear: regulatory peace of mind, a loyal and trusting customer base, empowered employees, and the freedom to innovate boldly yet responsibly. In a competitive retail market, these advantages set you apart.
By acting now to put a robust AI governance framework in place, you position your supplement brand as a leader in the new era of retail – one that harnesses cutting-edge technology while staying true to ethical principles and customer-centric values. In doing so, you’re not only mitigating risks; you’re building a brand that can proudly say it uses AI for good – to serve customers better, fairly, and transparently. And that is a brand promise that will carry you well into the future.
(If your organization is looking for expert guidance on implementing AI governance effectively, consider reaching out to specialized consultants or industry groups. With the right support and vision, any company can become a role model for responsible AI in retail.)
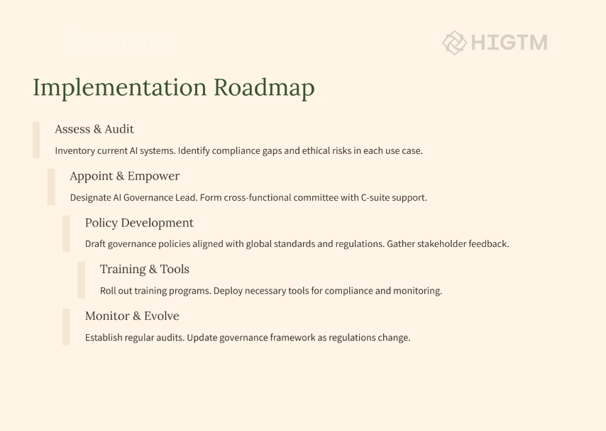
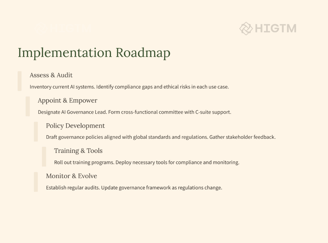


Turn AI into ROI — Win Faster with HIGTM.
Consult with us to discuss how to manage and grow your business operations with AI.
© 2025 HIGTM. All rights reserved.