7. The AI Adoption Curve – Understanding readiness levels
How can an SME leader know if their organization is truly prepared for AI—and what steps must be taken to integrate AI responsibly and profitably? This article unpacks the AI Adoption Curve—a framework that helps businesses determine where they currently stand on the path from initial AI curiosity to mature, enterprise-wide usage. By understanding key stages like awareness, proof of concept, implementation, scaling, and maturity, SMEs can map out actionable plans that respect both budget constraints and long-term strategic goals. We’ll also explore critical readiness factors, typical barriers, and best practices for a smooth AI journey.
Q1: FOUNDATIONS OF AI IN SME MANAGEMENT - CHAPTER 1 (DAYS 1–31): CORE AI CONCEPTS & VALUE PROPOSITION
Gary Stoyanov PhD
1/7/20256 min read
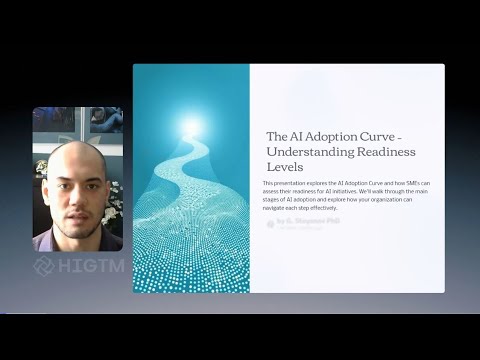
1. Overview of the AI Adoption Curve
The AI Adoption Curve illustrates how organizations progress from exploring AI possibilities to fully weaving AI into daily operations. Much like the Technology Adoption Life Cycle (familiar in other tech contexts), it provides a structured lens through which SME leaders can evaluate their current position and clarify next steps.
Awareness: Basic familiarity, often fueled by headlines or peer success stories.
Proof of Concept (PoC): Small-scale experiments validating feasibility and ROI.
Implementation: Operational integration of successful AI pilots, requiring staff training and governance.
Scaling & Optimization: Broad AI deployment across departments, with continuous performance improvements.
Maturity: AI is embedded in everyday strategy and decision-making, driving innovation consistently.
For an SME, this curve also highlights resource considerations—from minimal initial investments in PoC projects to the more substantial commitments involved in scaling or reaching maturity.
Why It Matters
Strategic Clarity: Knowing where you stand keeps AI initiatives aligned with business goals.
Resource Allocation: Helps leaders plan budgets, staffing, and timelines realistically.
Progress Milestones: Lays out a roadmap for transitioning from pilot successes to enterprise-wide adoption.
2. Key Stages in Adopting AI
Each step of the Adoption Curve presents unique challenges and goals. Recognizing these stages prevents SMEs from jumping in blindly or missing essential prerequisites like data preparedness or executive alignment.
2.1 Awareness
Characteristics
Occasional mentions of AI in meetings.
Leaders might read about AI in industry publications but lack a specific plan.
Staff curiosity: some employees may suggest automation ideas without a formalized approach.
SME Tip: Start small—read case studies from similar-sized companies, attend webinars, and note potential use cases. If you run a boutique retail business, for example, you could explore how AI-driven inventory forecasting saved a peer organization time and money. Such insights feed into initial discussions around feasibility and benefits.
2.2 Proof of Concept (PoC)
Characteristics
Testing AI in a controlled or limited environment, often using minimal data or a small user group.
Clear objectives, like automating a basic process (e.g., data entry) or predicting a specific metric (e.g., sales volume).
Tangible success criteria (accuracy rate, labor hours saved, user satisfaction).
SME Tip: Focus on a single clear objective. A small retailer might pilot an AI chatbot to handle routine customer queries. Or a manufacturing SME might use a predictive maintenance solution on one machine line. Choose a use case that has visible impact, ensuring buy-in from both staff and stakeholders.
PoC Benefits
Validation: Confirms whether the chosen AI approach is viable and beneficial.
Cultural Introduction: Employees get hands-on experience with AI, reducing fear or skepticism.
ROI Measurement: Early results can demonstrate cost savings or revenue improvements, justifying broader expansion.
2.3 Implementation
Characteristics
Moving from pilot to operational integration.
Formal data pipelines, staff training, and performance tracking systems are established.
Cross-functional collaboration ensures departments share relevant data, adopt consistent processes, and avoid silos.
SME Tip: Secure executive buy-in and maintain open communication. AI projects often affect multiple departments—IT, marketing, HR—so a champion at the leadership level can help coordinate tasks and budgeting. Establish basic data governance practices to ensure your data is consistent, secure, and properly utilized.
Implementation Essentials
Data Readiness: Clean, well-structured data is the lifeblood of any AI model.
Governance: Policies outlining responsibilities, ethical guidelines, and compliance measures (e.g., GDPR).
Performance Tracking: Dashboards to monitor metrics like prediction accuracy, error rates, or cost savings.
2.4 Scaling & Optimization
Characteristics
AI tools extend across multiple workflows or departments—marketing, finance, customer support, etc.
Continuous improvements driven by performance metrics and user feedback.
AI starts delivering more consistent ROI and influences strategic decisions.
SME Tip: Document best practices from earlier stages and refine process flows so new teams can quickly get up to speed. Keep a close eye on your Return on Investment (ROI)—if a particular model performs exceptionally, replicate that success in similar contexts.
Challenges
Resource Management: Scaling AI demands more data storage, computing power, and possibly staff training.
Change Management: Larger AI rollouts can trigger resistance, especially if employees worry about job changes or role shifts. Transparency and training remain key.
2.5 Maturity
Characteristics
AI is embedded in day-to-day operations and strategic planning.
New ideas or projects automatically consider AI possibilities.
Regularly updated models and data, cross-functional AI literacy, and strong governance frameworks.
SME Tip: Even at maturity, maintain a culture of continuous learning. Markets, technologies, and consumer behaviors evolve, so recheck your AI models and re-evaluate your infrastructure periodically. Thoughtful leadership in AI fosters ongoing innovation, not complacency.
Defining Features
C-Suite Sponsorship: Executive leadership sets AI strategies at the organizational level.
Scalable Infrastructure: Cloud or on-prem hardware readily expands with new use cases.
Talent Pipeline: Data scientists, machine learning engineers, or upskilled employees handle advanced projects confidently.
3. Assessing AI Readiness for SMEs
Moving along the curve effectively starts with an honest evaluation of readiness—both in terms of technology and people.
3.1 Data Quality & Accessibility
Core Question: Does your organization have clean, relevant, and accessible data sources?
Action Step: Conduct a data audit—spot duplicates, fill missing fields, unify formats. For example, an e-commerce SME might integrate sales data from separate POS systems, ensuring a single source of truth.
3.2 Workforce Competencies
Core Question: Is your team knowledgeable in basic AI/ML concepts? Do you have in-house data skills or a plan to acquire them?
Action Step: Provide training or partner with external consultants. Promoting a data-driven mindset among existing staff can catalyze successful AI adoption.
3.3 Leadership & Culture
Core Question: Are leaders willing to invest in AI initiatives and champion organizational changes?
Action Step: Secure executive sponsorship early, develop clear objectives, and encourage a culture that sees AI as an augmentation of human talent, not a replacement.
3.4 Technology Infrastructure
Core Question: Does your existing software ecosystem support AI workloads, like data pipelines, cloud storage, or real-time analytics?
Action Step: Assess if local hardware suffices or if you need cloud-based solutions. Start with a scalable platform that can expand as your needs grow.
3.5 Budget & ROI Expectations
Core Question: What resources can you realistically allocate, and what ROI do you aim to achieve?
Action Step: Begin with small, impactful projects that justify budget expansions once they demonstrate value. Consider seeking grants, incentives, or subscription-based AI services to mitigate costs.
4. Overcoming Common Barriers
Even with the best readiness plan, SMEs often encounter obstacles—from resource limitations to cultural resistance. Recognizing these pitfalls helps you craft proactive solutions.
Fear of Complexity
Solution: Start with low-risk, high-value use cases. A well-defined pilot is less intimidating and fosters confidence.
Limited Resources
Solution: Explore cloud-based AI tools or partner with consultants. Pay-as-you-go services cut upfront hardware investments.
Resistance to Change
Solution: Communicate benefits to staff. Show how AI offloads mundane tasks, creating space for strategic, human-centric roles. Offer training and celebrate early wins to build momentum.
Data Silos
Solution: Streamline and unify data sources. Break departmental barriers by establishing an overarching governance framework where data flows freely, ensuring AI solutions access complete information.
HIGTM Perspective: Strategically addressing these barriers ensures your AI adoption journey stays on track. Each SME may have a unique mix of challenges, but the underlying principle remains the same: tackle them methodically with careful planning, communication, and measurable steps.
5. Best Practices & Next Steps
A structured approach to AI yields the most consistent results. Here are key tactics to ensure your SME navigates the AI Adoption Curve smoothly:
5.1 Define Clear Objectives
Why: AI projects flounder when goals are unclear. Are you aiming to reduce operational costs, improve customer satisfaction, or enhance product quality?
How: Pinpoint metrics—like error reduction percentages or improved lead conversion rates—and ensure all stakeholders agree on success criteria.
5.2 Measure & Refine
Why: AI models degrade over time if data shifts, and business needs change rapidly.
How: Establish KPIs (e.g., lead response time, forecast accuracy), track them regularly in dashboards, and pivot strategies if results lag behind targets.
5.3 Foster Ongoing Learning
Why: AI evolves quickly; staff upskilling prevents knowledge gaps and fosters innovation.
How: Host lunch-and-learn sessions, send employees to data science conferences, or sponsor eLearning courses. Encourage cross-functional AI task forces.
5.4 Stay Informed
Why: AI trends move fast; new open-source frameworks, vendor offerings, and best practices emerge continuously.
How: Assign a point person or small team to monitor AI developments relevant to your industry. Subscribe to reputable news sources or academic journals. Regularly evaluate if these advancements can replace or upgrade existing solutions.
5.5 Leverage Expert Guidance
Why: Adopting AI in isolation can be time-consuming and prone to missteps.
How: Engage consultants or AI solution providers—like HIGTM—to plan your roadmap, choose fitting technologies, and guide early implementations. External partners often supply specialized expertise you can’t cost-effectively build in-house.
At HIGTM, we specialize in holistic AI adoption strategies for SMEs, ensuring each step—from awareness to maturity—ties into your existing structures, data strengths, and operational ambitions.
By recognizing your place on the curve and methodically pushing forward, your SME can harness AI’s capabilities in incremental, sustainable ways.
Whether you’re automating a single workflow or heading toward enterprise-wide analytics, the AI journey becomes a structured, rewarding endeavor, unlocking new efficiencies and growth opportunities at every turn.
Turn AI into ROI — Win Faster with HIGTM.
Consult with us to discuss how to manage and grow your business operations with AI.
© 2025 HIGTM. All rights reserved.