69. Real-Time Performance Dashboards in Fashion E-Commerce
Recently, real-time performance dashboards have become the secret weapon of industry leaders. These dashboards aggregate live data across supply chains, online platforms, and physical stores, translating it into actionable insights and alerts. Below, we explore how fashion retailers are leveraging AI-driven dashboards to optimize every facet of their operations – from inventory and supply chain to customer engagement and omnichannel integration – and why staying ahead in real time is now mission-critical. We also delve into case studies from global giants and examine regional nuances, all to provide a clear roadmap for maximizing ROI through real-time monitoring.
Q1: FOUNDATIONS OF AI IN SME MANAGEMENT - CHAPTER 3 (DAYS 60–90): LAYING OPERATIONAL FOUNDATIONS
Gary Stoyanov PhD
3/10/202540 min read
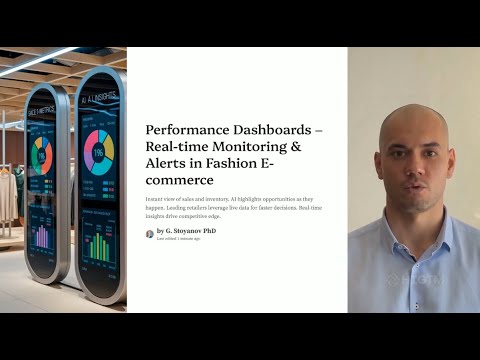
1. The Rise of Real-Time Dashboards in Fashion Retail
Modern fashion retailers are abandoning static weekly reports in favor of real-time dashboards that update every second with fresh metrics. These systems act as a live “mission control,” enabling brands to respond instantly to opportunities or issues. Unlike traditional business intelligence, which looks backward, real-time dashboards provide a constantly up-to-date forward view. In an industry where a trend can explode overnight on social media, this immediacy can make the difference between capitalizing on a craze or missing out. Crucially, AI analytics are embedded in these dashboards to not only display data but also interpret it – detecting patterns, anomalies, and emerging trends that human managers might miss until it’s too late.
Leading fashion companies have heavily invested in such capabilities. For instance, Zara (part of Inditex) is known for its vertically integrated supply chain and use of technology to achieve fast reactions. Zara’s stores feed sales data to a central system in real time; managers see which items are selling out by size or region and can communicate needs to production instantly. A hallmark of Inditex’s approach was its rollout of RFID tracking in over 700 stores, which allows item-level inventory visibility at all times. The dashboard shows exactly where each product is – on the shelf, in the stockroom, or sold – and flags what needs restocking during the twice-weekly shipment cycles. This real-time inventory intelligence has enabled Zara to achieve very high inventory turnover and minimize “out of stock” scenarios.
Another pioneer is Shein, the Chinese ultra-fast-fashion upstart. Analysts have dubbed Shein’s model “real-time retail” because of how quickly it can identify a trend, design a product, and have it available for purchase. Shein’s secret is a dashboard-driven supply chain: it uses AI to scrape fashion trend data from social media, search queries, and its own platform behavior, instantly recognizing patterns in what styles or keywords are gaining traction. This live trend data feeds into design and production planning. Shein can create new designs in as little as 3 days based on data insights, far outpacing traditional retailers’ calendars. Its dashboard doesn’t just track internal metrics – it’s also monitoring the external zeitgeist in real time. The result is an assortment that’s hyper-responsive to consumer preferences as they evolve day by day.
Moreover, real-time dashboards have proven critical for managing massive e-commerce events. Alibaba’s Singles’ Day (11.11) is a prime example of scale: Alibaba’s systems handle record-breaking order volumes (peaking at hundreds of thousands of orders per second) and display live sales tallies. Internally, their AI-driven dashboards help coordinate logistics and customer service during the sale frenzy. The ability to see transactions, inventory levels, and delivery fleet statuses second-by-second is essential to keeping the machine running smoothly on the year’s busiest shopping day. While not every brand faces Alibaba-level scale, the principle holds: live dashboards ensure you’re never flying blind, no matter how intense the traffic surge.
Key Takeaway: The shift to real-time, AI-enhanced dashboards is transforming fashion retail from reactive to proactive. Companies adopting these tools gain a time advantage – they spot what’s happening now (or likely to happen soon) and adjust immediately. This sets the stage for improvements across all critical KPI areas, which we explore next.
2. Supply Chain & Inventory Optimization (Live KPI Monitoring)
A fashion retailer’s supply chain is its lifeblood, and performance dashboards have become the stethoscope for supply chain health. Traditionally, metrics like inventory turnover, sell-through rate, or order fulfillment time were looked at after the fact. Now, with IoT sensors and integrated systems, brands monitor these KPIs in real time and use AI to optimize them continuously.
2.1 Inventory Turnover & Sell-Through
Inventory turnover measures how many times stock is sold and replaced in a period. High turnover is usually good – it means you’re not sitting on products for too long. Dashboards track daily (even hourly) sales against inventory levels by SKU. For example, if a particular dress style is selling extremely fast in Week 1 of launch, the dashboard will reflect a rapid depletion of stock. A high turnover or sell-through percentage triggers actions: planners may initiate a replenishment order immediately or shift inventory from stores where it’s slower-selling. Inditex excels here; its system reportedly can detect by midday if a new item is a hit or a flop. If it’s a hit, manufacturing orders are increased the same day to restock in a week’s time; if it’s a flop, items might be marked for early discount or not re-ordered. This agility keeps Zara’s inventory turnover high and piles of unsold stock low.
2.2 Production Cycle & Lead Times
The production cycle – design to manufacturing to distribution – is also tracked. A real-time project dashboard can monitor each step of the cycle for each collection. Any delays in fabric delivery or factory production are visible as they occur. AI can compare current progress vs. plan and estimate if shipping dates will be met, alerting managers to expedite or reroute tasks. Fast fashion brands target very short cycle times (2-4 weeks), so every day saved or lost matters. With live tracking, if a batch of product finishes early, the retailer can decide to launch it online immediately rather than waiting, thereby capturing sales sooner. Conversely, if a delay is spotted, marketing might adjust by not promoting that item yet to avoid stock-outs. This synchronization is only possible through integrated dashboards where supply chain data flows freely.
2.3 Demand Forecast Accuracy
One of the biggest challenges is predicting demand accurately – order too much and you have excess, order too little and you miss sales. AI-driven dashboards improve forecast accuracy by constantly updating predictions with the latest data. Traditional forecasts might be monthly; an AI system recalculates daily, factoring in recent trends, weather changes, social media buzz, etc. Nike, for example, leverages demand-sensing analytics (bolstered by its acquisition of Celect, a predictive analytics firm) to adjust inventory allocation. By analyzing historical sales and real-time store data, AI fine-tunes forecasts store-by-store.
The dashboard shows a “forecast vs actual” comparison in real time. If actual sales are exceeding the forecast significantly, the system learns from it and updates future predictions while also sending an alert to increase replenishment. The continuous learning loop has led to much improved accuracy for those using such systems – meaning fewer stockouts and less markdown waste. In effect, the forecast is never static; it’s a living number on the dashboard.
2.4 Return Rates & Reverse Logistics
Fashion e-commerce sees return rates anywhere from 20% to 40%. Dashboards monitor returns as a critical KPI, not an afterthought. A spike in returns for a particular item triggers investigation – perhaps a sizing issue or a product defect. AI can text-mine return reasons or customer feedback in real time to pinpoint problems (e.g., “runs small” appearing frequently). By catching this early, the retailer might update the product description with better sizing info or adjust the fit in the next production batch. Efficient reverse logistics (getting items back and re-entering them to inventory or disposing) is also tracked. Metrics like average time to process a return, or recovery rate of returned stock (how much can be resold), appear on operations dashboards. Some fashion players are using AI to reduce returns proactively: for instance, Amazon introduced an AI-based “Fit Insight” tool to help customers choose the right size and thus avoid returns.
When such tools are employed, their impact (did return rates drop?) is immediately visible in the dashboard data. Lower returns directly improve profitability and customer satisfaction, so this KPI is vital to manage in real time.
2.5 Auto-Replenishment & Low-Stock Alerts
Perhaps the most tangible supply chain benefit of real-time dashboards is automated stock replenishment. AI monitors inventory levels at each node (warehouse, store, website) and knows the threshold at which new stock should be ordered or reallocated. When an SKU’s inventory falls below the threshold, an alert might pop up: “Item X in EU warehouse down to 50 units – 2 days cover left.” Some systems go further and automatically create a purchase order or transfer request when such conditions are met. Nike’s supply chain AI, for example, is noted to send automated low-stock alerts to ensure timely restocking.
These alerts can also account for demand surges – e.g., if an item is trending and selling faster than usual, the AI dynamically raises the threshold (knowing that “2 days cover” in a surge is more critical than 2 days in normal times). On the dashboard, planners get a clear list of items requiring attention ranked by urgency. This kind of dynamic restocking was historically done by rule of thumb; now it’s data-driven and much more precise.
To illustrate the ROI: when LVMH implemented AI in parts of its supply chain, it achieved a more accurate demand forecast and noticeably reduced incidences of stockouts and overstocks.
Less stockouts mean more sales captured; less overstock means less discounting. Likewise, JD.com (a massive Chinese e-commerce player) integrated real-time inventory algorithms in its warehouses and was able to fulfill 90% of orders within 24 hours – a service level that earns customer loyalty. JD’s system optimizes inventory allocation across its network by analyzing orders and co-purchase data to stock the right products at local warehouses, and it provides clear, actionable insights on inventory management via its dashboard. This level of efficiency is unattainable without live data and smart automation.
Key Takeaway: Real-time dashboards supercharge supply chain and inventory KPIs by keeping every stakeholder informed up to the minute and letting AI take on the heavy lifting of predictions and routine decisions. The result is a leaner, faster supply chain – one that keeps shelves full of what customers want and minimizes both shortages and excess.
3. E-Commerce & Customer Engagement Metrics in Real Time
On the customer-facing side of fashion e-commerce, attention spans are short and competition is fierce. Real-time dashboards for customer engagement ensure that online retailers can deliver a responsive and personalized shopping experience. Here we look at the key digital metrics tracked and optimized continuously.
3.1 Real-Time Website Traffic & Conversion Funnel
E-commerce teams keep a close eye on how many visitors are on the site, which pages they’re viewing, and how far they get in the purchase funnel. A dashboard might show active users on the site right now, add-to-cart rate, checkout conversion rate, and sales per minute. This is like a heartbeat monitor for the online store. If traffic suddenly spikes (perhaps an influencer mentioned the brand or a flash sale email went out), the team sees it on the dashboard and can ensure the site can handle the load. If conversion rate drops at a certain step (say many people add to cart but don’t check out in the last 10 minutes), that’s a red flag – maybe a payment gateway is down or a promo code isn’t working. Real-time monitoring means issues can be detected and fixed within minutes, reducing lost sales. It’s the difference between discovering a checkout bug after an hour (when hundreds of customers might have given up) versus after a day (thousands lost). This agility in monitoring has improved uptime and conversion metrics for many retailers. For example, fashion retailer ASOS has a “trading dashboard” that teams watch hourly to track sales against targets and key site metrics, enabling them to react quickly if something looks off (though specific internal details are proprietary, the practice is common in the industry).
3.2 Personalized Recommendation Performance
Personalization is a major lever in online fashion – showing each shopper products they are likely to love. AI recommendation engines are typically integrated into dashboards to report their performance: click-through rates on recommended items, conversion of those clicks to purchases, and overall contribution to revenue. These stats can be viewed in real time or near-real-time. Farfetch, for instance, uses an AI engine (built on Vespa.ai) for real-time personalized recommendations to its millions of users.
On Farfetch’s side, the team can see how those recommendations are driving engagement at any moment. If the recommendation module on the homepage is underperforming (maybe due to a bad model update or stale data), it will show up as a dip in click-through on the dashboard, prompting an immediate review. Conversely, if certain recommendations are getting unusual traction (e.g., a lot of people are clicking on a particular sneaker being recommended), that insight feeds back to merchandising – perhaps stock more of that sneaker or feature it in marketing. Some systems even allow A/B testing of recommendation strategies on the fly, with the dashboard updating results in real time. The ASOS data science team has noted that real-time tracking of individual behavior allows them to scale personalized recommendations quickly to all users whenever they deploy a new algorithm.
The effectiveness of these recommendations (in terms of engagement and sales lift) is transparently measured, which reinforces investment in AI personalization.
3.3 Cart Abandonment & Recovery
Cart abandonment – when a customer adds items to their online cart but leaves without purchasing – is a metric of doom for e-commerce but also an opportunity. Dashboards track the number of active carts, the abandonment rate, and even identify who is abandoning (loyal customer vs. new visitor, etc.). With real-time data, the moment a cart is abandoned, it can trigger an automated recovery workflow: maybe an email is sent after an hour, or a push notification reminds the shopper “Your items are almost sold out, complete your purchase!” Some advanced systems use chatbots or live chat prompts if they detect hesitation. The performance of these tactics is visible on the dashboard – e.g., how many abandoned carts were recovered in the last hour, and what that added to revenue. If recovery rates dip, teams can experiment with different incentives (maybe a slightly bigger discount code) and watch the impact live. The goal is to minimize lost carts. A study might show that real-time intervention (like an exit-intent popup offering help or a promo) can recapture a significant percentage of would-be abandons. Retailers like Amazon and Nordstrom have honed such systems; smaller fashion players now have tools to do this too. The dashboard ensures that these micro-conversions are optimized continuously. Over time, even a 5% improvement in cart recovery can equate to millions in revenue for a large retailer, which justifies the real-time focus.
3.4 Social Media Engagement & Influencer Impact
Fashion trends live and die on social media. Dashboards increasingly incorporate social listening data alongside web analytics. For example, a brand’s dashboard might show volume of brand mentions on Twitter, sentiment analysis of comments, or engagement metrics of a new Instagram post – all updated frequently. If a campaign or an influencer collaboration is underway, the dashboard helps teams track the live impact. Suppose a famous influencer posts about a handbag at 3 PM. The social media panel of the dashboard shows a spike in mentions and traffic sources coming from that influencer’s link, while the sales panel shows handbag orders shooting up within the next hour. This correlation, shown in real time, not only proves the ROI of influencer marketing but also alerts the supply chain to a potential stock issue (better ensure that handbag doesn’t sell out too fast, or if it will, prepare a waitlist or fast restock).
Brands like Fashion Nova (known for influencer-driven success) reportedly keep a very close watch on how every post translates to site traffic and sales, adjusting their social strategy accordingly. Additionally, sentiment analysis running on tweets or reviews can feed into alerts – e.g., if sentiment drops (maybe due to a quality issue going viral), the PR team is immediately informed to take action. In sum, the dashboard bridges marketing and commerce in a live feedback loop. It ensures that virality is caught and leveraged, and any negative waves are managed swiftly.
3.5 Dynamic Pricing & Promotions
While not as common in fashion as in, say, airline tickets, dynamic pricing is emerging in retail. AI can help adjust prices or promotions in real time based on demand, inventory, or competitor prices. Dashboards that include a pricing module will show when such changes occur and what effect they have. For example, an AI might drop the price of a slow-moving item by 10% and the dashboard would then show if its sales velocity improves over the next few hours. If the item starts selling faster (and this is tracked live), the margin trade-off vs. inventory reduction is calculated to see if it was worth it. Conversely, if an item is selling too fast (running out too quickly), some retailers might even raise the price slightly to manage demand – the real-time elasticity can be tested. Intelligence Node, a retail analytics firm, notes that retailers can set rules to always stay, say, 5% below a competitor’s price in real time.
These rules are executed by AI and the outcome (market share gain, profit impact) is monitored. Fashion retailers also use dynamic promotions – like flash sales or limited-time offers that are launched on short notice. With dashboards, they can gauge the immediate lift from such promos and decide whether to extend or tweak them. The speed of feedback is crucial; if a flash sale at noon isn’t drawing enough interest by 1 PM, they might amplify it via additional channels by 1:05 PM. Live data makes marketing truly agile.
A concrete example on promotions: ASOS is known for frequent promotional tweaks. If a certain country’s sales are lagging target by mid-day, ASOS might deploy a localized promo code and then watch in real time if it closes the gap by end of day. This granular control wasn’t possible before – you’d see results next day or next week. Now it’s like steering a race car versus driving a truck; quick adjustments to keep performance on track.
Key Takeaway: In e-commerce, a real-time dashboard is like having a conversation with your customers and your website as it happens. You can see what they’re doing, how they’re responding, and adjust your approach in that very moment. The payoff is higher engagement, more conversions, and a smoother customer experience – all of which drive revenue. Brands that master this can significantly outperform those that rely on stale data or gut instinct for their digital strategy.
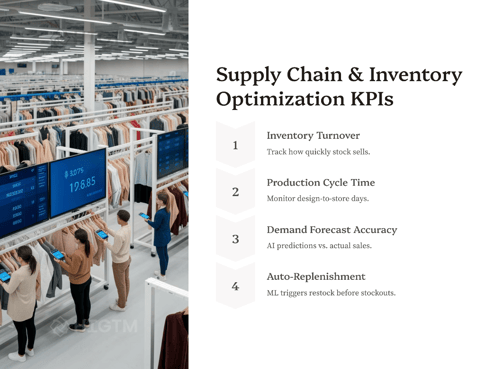
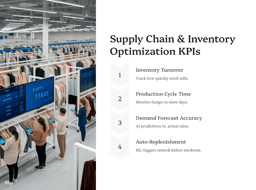
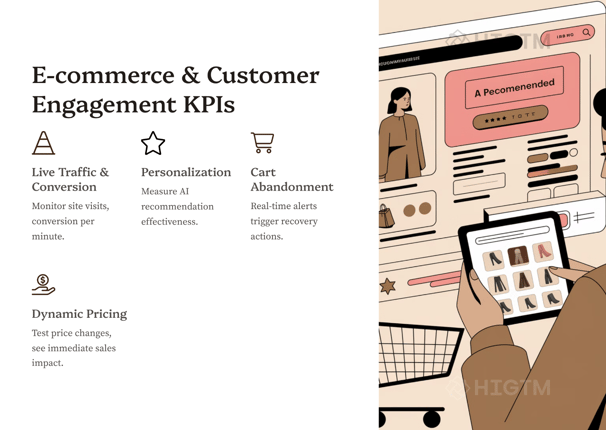
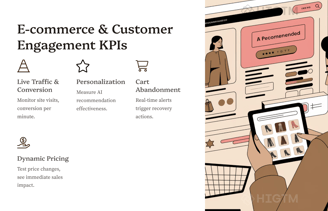
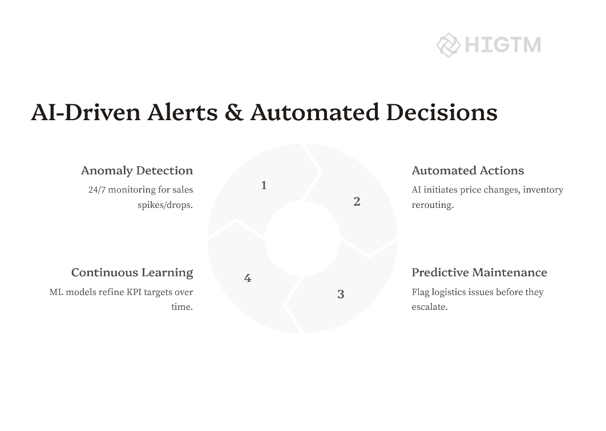
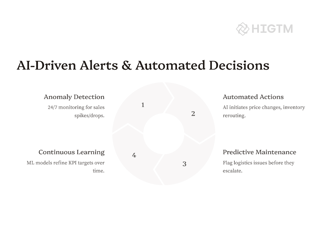
4. Omnichannel & In-Store Integration: Bridging Physical and Digital
Despite the surge in online shopping, physical stores remain vital in fashion. However, the lines between online and offline have blurred – customers expect a seamless experience. Real-time dashboards help retailers manage omnichannel KPIs and ensure that stores and e-commerce operate in lockstep, augmented by AI analytics in both realms.
4.1 Foot Traffic & Conversion (Physical Stores)
Just as we monitor website visits and conversion, savvy retailers monitor store foot traffic and conversion (the percentage of visitors who make a purchase) in real time. Technologies like people counters at doors, Wi-Fi tracking of smartphones, or computer vision can feed live footfall data to a dashboard. Managers at HQ or regionally can see, for example, how many people have visited each store so far today versus last week. AI can also predict by noon how many will likely visit by end of day, based on trends and external factors (weather, events). If foot traffic is unexpectedly high in a location, the dashboard could alert: maybe deploy extra staff from the backroom to the floor to assist customers (preventing missed sales due to long queues). If conversion in store is down, that’s a flag to investigate – are fitting room wait times too long? Is a popular item out of stock in that store? This overlaps with in-store inventory tracking; if foot traffic is there but conversion is low, an AI might correlate it with inventory data and find, for instance, that many people scanned for a particular product that was unavailable. AI-driven foot traffic analysis also helps with store layouts and marketing: heatmaps showing where customers dwell can be part of the dashboard, guiding visual merchandising (e.g., place new arrivals in that hotspot). Over time, optimizing these metrics means stores become more productive – a key goal as brick-and-mortar faces pressure.
4.2 RFID-Based Inventory & Stock Alerts
As mentioned earlier, RFID technology has been a game-changer for in-store inventory visibility. With RFID readers, stores can do cycle counts of all inventory very frequently (even daily). These readings feed into the central inventory dashboard. The benefit is that the same system that shows e-commerce stock also shows store stock in near real time. This underpins omnichannel services like Buy-Online-Pickup-In-Store (BOPIS) or ship-from-store. For example, a customer orders a jacket online for pickup, the dashboard immediately flags the store to set it aside, and inventory counts update so another channel doesn’t sell it. If a store’s RFID scan shows an item count is low, it triggers a restock from the stockroom or a shipment from a distribution center. Inditex’s RFID deployment allowed them to know instantaneously if a size M black dress is available in a specific store or even a nearby store, which greatly improves customer service (sales associates can check availability via the system and not lose a sale).
Real-time stock availability is also exposed to customers (store inventory lookup on websites) which is only reliable thanks to these dashboards. When it comes to alerts, a common setup is that if an item’s inventory goes below a threshold in any store, the managers get an alert to replenish from back stock or request more. This can be prioritized by importance (core products vs. less important ones). The cumulative effect is higher in-store sales (products are on the shelf when people want to buy) and fewer disappointed customers. It also reduces safety stock levels because the precision is better – you don’t need as much buffer when you trust the real-time data accuracy.
4.3 Smart Fitting Rooms and Mirrors
Many innovative retailers have tested smart mirrors or interactive fitting rooms that allow customers to request sizes, see outfit recommendations, or even try augmented reality overlays of different colors or styles. These devices generate engagement data – e.g., how many people used the mirror, which items were virtually tried on most, how many requested different sizes. All this can funnel into a dashboard. A store manager’s dashboard might show: “Fitting Room 3 had 50 sessions today, 30% resulted in a purchase, 5-star average rating on mirror experience.” If smart mirrors show that a lot of people virtually “tried” a certain item but didn’t end up buying, that could indicate the item looked better virtually than in reality (or price was a barrier). It’s a new type of data, but highly valuable. Nike’s recent use of AR mirrors in its NYC store (via Snapchat’s platform) is an example – these not only entertained shoppers but provided Nike with data on what people engaged with and what drove them to buy.
The dashboards capturing these metrics help justify the ROI of such tech and guide future deployments. They also feed into personalization – if a customer logged in and tried on outfits virtually, that data can sync with their online profile (so next time they’re online, the recommendations reflect what they tried in store). It’s the embodiment of omnichannel: store and online data blending to give a 360° view of customer behavior.
4.4 Cross-Channel Conversion & Fulfillment
Dashboards track how effectively channels are working together. One key metric is BOPIS usage and conversion – how many online orders are picked up in store, and does that customer buy anything extra when they come in? A bump in units per transaction for BOPIS customers could be caught and attributed to, say, a strategy of offering an in-store coupon at pickup. If data shows 20% of BOPIS customers make an additional purchase in store (and that figure is live on the dashboard), the retailer might quickly roll out this practice chain-wide. Another metric is online orders fulfilled by stores (store fulfillment can reduce shipping time and balance inventory).
The dashboard will show if stores are meeting the fulfillment SLA (e.g., ship within 24 hours of order) and if any store is overwhelmed with orders, at which point load balancing might kick in (route some orders to a nearby store or warehouse). AI can optimize this: deciding in real time which location should fulfill an order to minimize delivery time and cost, based on inventory and capacity. The performance of that algorithm is reflected in KPIs like fulfillment cost per order or average delivery time. Farfetch provides an interesting case as a platform: it links 1,400 boutiques’ inventories and uses AI to present a unified stock to customers.
Their dashboard (and those they provide to partners) allows monitoring of inventory across the network and facilitates services like in-store returns for online purchases. Retailers partnering with Farfetch can see, in real time, what inventory is selling globally and adjust their stock accordingly. This kind of network-wide visibility is an omnichannel ideal – it ensures customers can get the product through any channel seamlessly.
4.5 Loss Prevention & Security
Physical stores deal with shrink (theft or loss), and AI is increasingly part of the security approach. A dashboard might integrate data from smart CCTV cameras or POS transaction monitoring to detect suspicious patterns. For example, if a high-value item’s RFID tag exits the store without a corresponding sale in the system, an alert can pop up (maybe someone slipped it past the cashier). Or if a certain store shows abnormal inventory count discrepancies at day’s end, it’s flagged for investigation.
AI can analyze video to count how many items went into a fitting room vs. out, alerting staff if something may have been concealed. These events, once processed by AI, appear on a security dashboard in real time so that loss prevention personnel can respond immediately (e.g., intercept at the door, or review footage while the customer is still on premises). Additionally, credit card fraud patterns (like multiple high-value online orders to the same address using different cards) can be spotted and shown on an e-com fraud dashboard, prompting cancellation of suspicious orders before they ship. The real-time nature here saves money – stopping fraud or theft in the act rather than after an audit weeks later. It also creates safer store environments and protects inventory investment.
Case in point: LVMH and other luxury retailers, who face theft and fraud attempts, have been investing in AI security systems. While details are kept confidential, it’s known that using data (from transaction logs, cameras, etc.) in real time can reduce shrink significantly. Even a few percentage points improvement translates to millions of dollars saved when dealing with expensive goods.
Key Takeaway: Omnichannel real-time dashboards ensure that physical stores are as data-informed as online operations. They break down silos between channels – inventory moves fluidly, customers transition from online to offline with continuity, and every touchpoint is optimized. In-store analytics enriches the picture with qualitative insights (like engagement with AR mirrors or foot traffic flows) that online alone can’t provide. Retailers who unify their data across channels can deliver a truly integrated experience – something consumers are beginning to expect as standard.
Real-world example: Nike’s NYC flagship store integrated an AR “smart mirror” that let shoppers virtually try on products and unlock exclusive offers, feeding data back to Nike’s dashboard. By merging digital and physical experiences, Nike sets an example of using real-time tech to boost engagement and bridge online and in-store shopping.
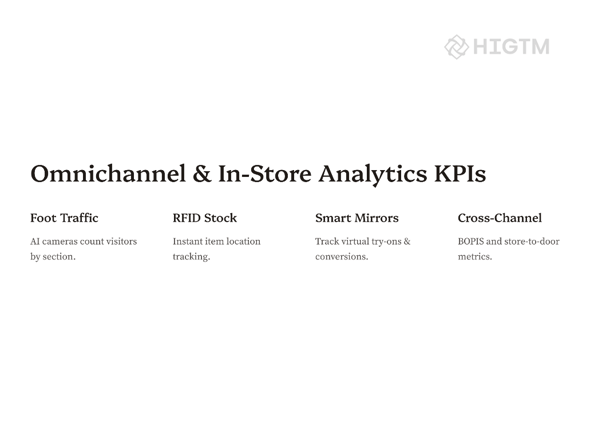
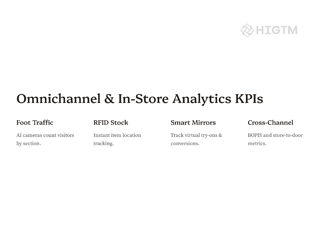
5. AI-Driven Alerts and Automated Decision-Making
One of the defining features of next-gen dashboards is the inclusion of AI alerts and automation, moving from merely descriptive dashboards to prescriptive and autonomous systems. In fashion retail, this can cover a lot of ground: from merchandising decisions to operational fixes, handled or suggested by AI in real time.
5.1 Anomaly Detection Alerts
AI excels at understanding what “normal” looks like in your data and flagging when something deviates sharply. Retailers set up anomaly detection on metrics like sales velocity, website traffic, average basket value, inventory variances, etc. For example, if a typical Wednesday sees $500k in sales by noon, but today it’s only $300k by noon, the AI will flag a potential issue – maybe a site glitch or a supply issue. Conversely, if a particular product is selling 5 times faster than usual (spike), it flags that too – a positive anomaly that still requires attention (ensure inventory can support it, investigate why it’s spiking – maybe an influencer mention). These alerts appear on dashboards as colored warnings or push notifications to relevant managers. The key is immediacy: rather than waiting for an end-of-day report or someone to notice, the AI is watching 24/7. Many retailers tie these alerts to mobile apps so on-duty managers get pinged. For mission-critical systems, an alert could even trigger a halt – e.g., anomaly detection on checkout errors might automatically page the IT team and switch the payment gateway if it suspects a problem. By addressing anomalies quickly, retailers prevent small issues from becoming big losses. For instance, catching a sudden conversion drop might reveal a promo code error that can be fixed in minutes, saving countless sales that would have been lost in hours of dysfunction.
5.2 Automated Price and Markdown Decisions
We touched on dynamic pricing; here we highlight how AI can automate it within bounds set by management. Fashion retailers often need to markdown items as they age to clear stock. AI can decide the optimal discount and timing based on real-time sales performance, inventory, and even weather (sunny weekend might increase store footfall, so maybe hold off markdown until next week if rain is expected). These decisions, once made by planners in meetings, can now happen daily. A dashboard might list items that AI marked down today along with rationale (e.g., “Item ABC: lowered price 15% – stock > 8 weeks cover, below target sales”). Some brands, especially in fast fashion, empower algorithms to run these micro-markdowns frequently to maximize sell-through and margin. This is done carefully to avoid brand damage, but when done right, it’s very effective in squeezing out more revenue. Mango, as an example (not in our list, but relevant industry peer), uses AI for markdown optimization – their system suggested price changes that improved sell-through with minimal margin sacrifice. In luxury, dynamic pricing is less used (brand value control), but even they use AI for localized pricing and inventory-driven adjustments (like identifying when to quietly offer a perk or free shipping on a high-end item that’s not selling in a particular region). All these AI decisions are reflected on dashboards for transparency and override if needed.
5.3 Inventory Allocation & Transfer Automation
In supply chain, AI can decide how to distribute products across various warehouses or stores. Historically, allocation was done pre-season with little adjustment. Now, as data comes in, AI might say: “We expected Dress X to sell evenly, but it’s selling more in urban stores than suburban. Let’s re-route remaining stock accordingly.” This can be automated: transfer orders get created and appear on the logistics dashboard. JD.com’s award-winning inventory optimization algorithm is a case in point – it integrates demand forecasting and iterative optimization to continually deliver allocation plans that maximize product availability with minimal cost.
The system gives clear insights and also essentially runs the allocation show, which contributed to JD fulfilling 90% of orders within 24 hours.
In practice, for a fashion retailer, this might mean an AI suggests or automatically moves stock between stores when one store has excess and another is selling out. The dashboard would highlight these transfers and their outcomes (e.g., how many potential lost sales were saved by the move). This also extends to automated replenishment from central to stores: AI decides the quantities each store gets in the next shipment, not just based on a fixed plan but the latest trends. It’s a rolling, ever-adjusting process – essentially real-time allocation versus set-it-and-forget-it.
5.4 Customer Engagement Automation
On the customer side, AI chatbots and personalized marketing can be triggered automatically by dashboard events. For example, if a customer is browsing for 10 minutes appearing indecisive, an AI chatbot might proactively pop up to assist (increasing conversion chances). If a high-value customer hasn’t purchased in a while, an AI-driven campaign might send them a personalized offer – and this trigger could be a rule on the CRM dashboard. LVMH implemented a sophisticated internal AI called MaIA to help support staff with content and customer queries.
While that’s more for internal use, customer-facing AIs like chatbots are widely used by fashion retailers to handle common queries (order status, return policy, product info) instantly. The real-time aspect is that these bots are “on” 24/7, and their performance (# of queries solved, CSAT scores) shows up on service dashboards continuously. If the bot encounters something it can’t handle (negative sentiment or complex issue), it can alert a human agent to step in – blending AI efficiency with human touch at the right moment. Retailers also use AI for real-time content personalization: banners on the website changing to show a category a user has shown interest in, or emails triggered by actions (abandoned cart, browse abandonment) that are completely automated. The coordination of these is visible to marketing teams via dashboards (so they don’t accidentally overlap communications or over-contact). Essentially, the AI is doing multi-tasking that a whole team of marketers and service reps might do manually, and the dashboard is where it’s orchestrated and supervised.
5.5 Continuous Learning & KPI Optimization
A powerful advantage of AI is that it can learn from new data. The models driving all the above (forecasting, recommendations, anomaly thresholds, etc.) get better over time by training on the latest info. The dashboard often includes tracking of model accuracy or impact. For instance, a forecast accuracy KPI might show it improved from 85% to 90% after the latest algorithm update – demonstrating learning.
Some retailers even deploy AI A/B tests and show results on dashboards: e.g., one algorithm vs another for recommendations – which yields higher revenue per user? Based on the live experiment, the better model is chosen. Over a year, this iterative approach might significantly elevate performance benchmarks. One concrete example is in pricing: an AI might start with a rule-based approach, learn price elasticity from data, and evolve into a more nuanced model that yields higher margins. The dashboard closes the loop by displaying the key outcome metrics that these models aim to improve (sales, margin, sell-through, NPS, etc.), so it’s always clear whether the AI is adding value. If not, tweaks are made.
Retailers like Stitch Fix (though not listed, known for AI) famously use algorithms in many decisions and constantly refine them. Traditional retailers are catching up, embedding AI to assist merchants and planners. The phrase “Quiet AI” used by LVMH’s CDAO refers to AI subtly advising human decision-makers without being intrusive – for example, a suggestion in the dashboard “This bag is trending in Asia, consider reallocating stock.” The human can review and approve, but the suggestion comes from data. This hybrid approach is becoming common: AI does the heavy analysis and proposes actions; humans oversee brand alignment and final calls.
Key Takeaway: AI-driven dashboards don’t just inform you about what is happening; they increasingly decide what to do about it, or at least give you a very strong nudge. By automating routine decisions and highlighting urgent exceptions, they free up human managers to focus on strategy and creativity. The result is a business that’s not only always aware, but always acting and adjusting – a living, breathing operation tuned to the market in real time. Fashion is an industry of constant change, and AI automation ensures the company changes in sync with the market, not behind it.
6. Global Insights: Regional Adaptations and Compliance
Fashion is a global business, but operating real-time AI dashboards worldwide requires understanding regional differences – both in consumer behavior and in regulatory environments. What works in one market may need tweaking in another. Additionally, the surge in data use has drawn regulatory attention (privacy, AI ethics), so any global dashboard initiative must bake in compliance from day one.
6.1 North America & Europe – Personalization vs. Privacy
Western markets have consumers who expect personalized experiences but also fiercely protect their privacy. Dashboards in these regions often incorporate sophisticated customer data platforms (CDPs) that unify data with privacy controls. For example, a European retailer’s dashboard might show rich customer segments and behavior, but everything is pseudonymized or aggregated unless the customer consented to personal data use (per GDPR). Consent dashboards are even a thing – showing what percentage of users opted in to tracking, etc., which can hint how reliable certain personalization analytics are. Under GDPR and similar laws, any real-time triggers that use personal data have to be justifiable and secure. This has led companies like LVMH to emphasize a “value exchange” – they found that consumers are more willing to share data if they get a better experience.
So their dashboards measure not just engagement, but also how consent rates and personalization correlate to sales, proving that respecting privacy can still yield insights. In the U.S., regulations are a bit less strict federally (though California’s CCPA is similar to GDPR in spirit). U.S. retailers use extensive loyalty data to fuel dashboards, but even they are preparing for a cookieless, more consent-driven world. The key is designing dashboards that can do a lot with a little data (when limits apply). AI helps by generalizing patterns without needing to identify individuals until they choose to identify (like logging in). On the flip side, these regions have strong omnichannel expectations – customers want inventory visibility online for stores, easy returns, etc. Real-time dashboards in NA and EU often put a big focus on cross-channel KPIs (as we detailed) to deliver on convenience. Culturally, Western firms might also be more cautious about letting AI run wild. There’s often a layer of human approval built in – AI recommendations go to a planner’s dashboard for review, rather than auto-executing. This is partly habit and partly risk management.
6.2 Asia – Scale, Speed, and Superapps
In Asia, particularly China, the scale of e-commerce and the digital ecosystem (think superapps like WeChat, or Alibaba’s empire) means dashboards there handle massive, diverse data streams. Chinese consumers have basically married social media, shopping, payments, and more into single platforms, so a dashboard might integrate all of that. For example, a brand on Tmall (Alibaba’s platform) can see not only sales data but also live chat inquiries, live-stream views, and influencer campaigns in one console. Alibaba itself offers analytics dashboards to merchants, providing real-time reporting on sales, customer demographics, and even benchmarking against category trends.
Chinese retailers are highly data-savvy and often more willing to experiment with AI. It’s common to see things like AI fashion assistants (Alibaba had a FashionAI concept store that recommended outfits in smart mirrors back in 2018), or facial recognition for VIP customers in stores, or dynamic in-app pricing and coupons that vary by user activity. Shein, although global, epitomizes an Asian approach: extreme responsiveness and iteration. It reportedly uses a “test and scale” model – launching very small batches of thousands of products, then using real-time sales data to decide which designs to mass-produce.
This is only feasible with a robust dashboard that can handle thousands of microproduct experiments simultaneously and an AI that can pluck winners and losers by analyzing the early sales signals. In Japan and South Korea, while data usage is high, there’s also attention to detail and quality – dashboards are tuned to monitor customer feedback closely (since brand reputation is paramount). In India and Southeast Asia, companies like Reliance (AJIO) or Lazada have looked to Chinese models for inspiration, implementing AI chatbots or recommendation engines to handle large multilingual, diverse markets in real time.
6.3 Localization of KPIs and Features
Regional differences also reflect in which KPIs matter more. In Europe, for luxury brands, maybe boutique appointment bookings or personal shopper interactions are tracked in real time (e.g., how many slots booked vs. available). In North America, holiday season metrics (Black Friday live sales, Cyber Monday site performance) might get special dashboards due to their huge importance. In Asia, live-stream commerce metrics (viewers, engagement, conversion from stream) are key – an area less prominent in the West until recently. So a global chain like Nike will have core common dashboards, but also local modules: their China team might have a dashboard section for monitoring sales coming from WeChat mini-programs; the Europe team might focus on multi-country inventory allocations and compliance metrics. The technology allows tailoring without losing the unified data model.
On the compliance side, data residency is a consideration – China’s laws require data about Chinese users to stay in China, for example. That might mean the global dashboard has federated pieces: the China data is processed on local servers and only aggregated insights flow up to global level. Similarly, for EU, some data might be stored and processed in EU regions to comply with GDPR. Designing a real-time system that still feels “single pane of glass” but respects these boundaries is non-trivial, but achievable with cloud infrastructure (many use multi-region cloud setups for this reason).
6.4 Regulatory & Ethical AI Monitoring
With AI making more decisions, regulators are starting to ask for accountability. In the EU, the upcoming AI Act will likely require transparency on AI decisions (especially if they impact consumers significantly). Retailers are preparing by logging AI decision rationales – and some dashboards include an “AI audit” tab showing what the AI did (e.g., “Algorithm changed price of item X at 3 PM, reason: low demand, rule in pricing policy”). This kind of logging can be crucial if later someone questions, “Why did you recommend this at that price to that customer?” Data privacy regulators also might audit how customer data is used in real time profiling. A robust system will allow toggling certain data usage off if a law says so (for example, not using location data in Europe without explicit consent – the dashboard would exclude it in EU views).
Another consideration is cybersecurity: real-time data is great, but it must be secure. Breaches of live customer data or even business performance data can be damaging (financially or reputationally). So global retailers incorporate security alerts into their dashboards – attempts of intrusion, system health, etc., because an outage or hack can affect all those lovely KPIs we discussed. Some companies even simulate what-if scenarios (disaster drills) and dashboards are used to run these – like a sudden supplier shutdown and seeing impact on inventory in real time to test resilience.
6.5 Adapting to Market Maturity
Not all regions have the same level of infrastructure. In some emerging markets, real-time might be hampered by patchy data collection. Fashion retailers expanding to such markets need to upgrade store tech (maybe introduce RFID or better POS) to get real-time data. A phased approach is common – start with online data (which is inherently real-time), then gradually plug in stores as they modernize. The dashboard becomes a carrot for modernization: once country managers see how useful live data is, they push to get their stores on board. This is a global rollout challenge that many companies manage by pilot programs. A positive peer pressure can happen: if Europe region shows off improvement due to a fancy dashboard, APAC region will want the same and invest in needed tech locally.
Key Takeaway: Implementing real-time, AI-driven dashboards is not a copy-paste job across markets. It requires understanding local consumer behavior (what to monitor), complying with local laws (how to monitor), and fitting into local tech ecosystems. The best companies use a global platform with local customization – ensuring everyone has the core benefits of real-time insight, but no one is violating norms or rules. By treating compliance and localization as features, not burdens, retailers actually build trust with consumers and regulators while still reaping the performance gains of AI analytics
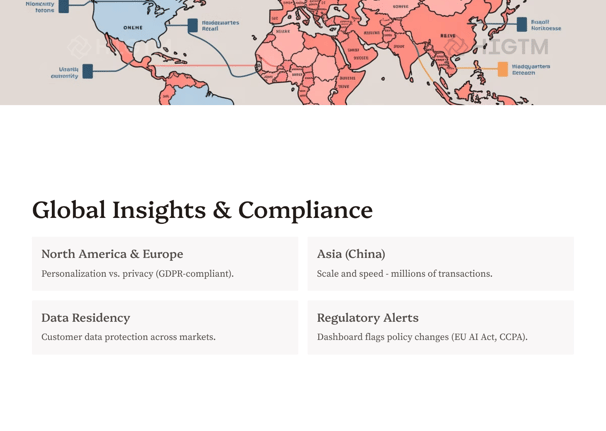
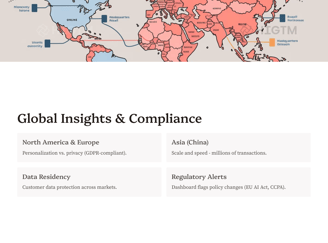
7. Case Studies of AI-Powered Dashboards in Fashion Retail
To ground all these concepts, let’s examine a few mini case studies highlighting how leading fashion e-commerce players have applied real-time dashboards and the tangible results achieved.
7.1 Inditex (Zara) – Fast Fashion Nerve Center
Zara’s parent company Inditex built an integrated central dashboard that links store sales, online sales, and inventory. Every store manager has a handheld device that feeds sales and inventory data to HQ in real time. Twice a week, Zara’s fulfillment centers use dashboard data to decide what each store should receive in the next shipment.
The decision is largely automated: the system knows what sold since the last shipment and calculates what each store needs to maximize sales (while factoring in new collections). If a certain design is red-hot in one country, the dashboard will show low stock there and available stock elsewhere, prompting a reallocation or rapid production push. Conversely, if a product isn’t moving, the dashboard signals to not send more and instead perhaps start markdowns. This tight feedback loop, enabled by real-time data, is credited with Zara’s ability to only have ~10% of its inventory as unsold at season’s end (significantly lower than industry average). Zara also connected its online and offline inventory early on – a customer in New York might order online, and if the local Zara store has it, the order can be fulfilled from there, shown by the system.
By 2018, Zara enabled all its stores to fulfill online orders thanks to RFID and dashboard visibility. A side benefit: Zara’s model of modest initial supply for new items and then rapid replenishment based on actual demand is essentially a live experiment. They’ve had situations where a bold style unexpectedly became a hit; their dashboard caught the sales spike within a day or two, and they quadrupled production orders immediately, riding the wave to millions in sales that a slower system would have missed. This real-time demand sensing is a cornerstone of Inditex’s success.
7.2 ASOS – Data Culture and Personalization
ASOS, a UK-based online fashion giant, attributes a lot of its growth to being highly data-driven. They built an in-house data science team and a suite of tools (surfaced in dashboards) to optimize the customer journey. Two notable features: a recommendation engine and a visual search tool. The recommendation engine at ASOS uses what they call “cognitive analytics” to match products to individual customers in real time, updating suggestions as a person browses.
The effectiveness of this is seen in their engagement metrics – ASOS tracks how many recommended products are clicked and what percentage convert to purchase. As they refined their algorithms, they saw continuous improvement in those KPIs, which they have publicly hinted contributes significantly to conversion rate and basket size. On the ops side, ASOS also closely monitors things like delivery performance and returns by region in real time. In 2020, ASOS faced a spike in demand during lockdowns; their dashboard alerted them that certain categories (like loungewear) were surging l, while formalwear tanked. They quickly pivoted their marketing and stock – pushing comfy clothes and dialing back orders of suits. Because they saw the trend early (daily data feeds), they aligned their offering with the new normal faster than some competitors, resulting in market share gains. ASOS’s culture is such that every morning “trading meetings” revolve around the latest dashboard numbers, making decisions for the day on assortment, site content, and marketing spend allocation. It’s like a sports team huddling to review the scoreboard and adjust their play in real time.
7.3 Alibaba & Tmall – Merchant Dashboards and AI Recommendations
Alibaba isn’t a single fashion retailer, but through Tmall it hosts many. Alibaba provides powerful dashboards to brands selling on its platform. These include real-time sales, traffic sources (showing the impact of the on-site promotions or Tmall’s own campaigns), and customer insight reports. One impressive AI-driven feature is Alibaba’s recommendation system which all Tmall retailers benefit from – often displayed as “You may also like” or on the Tmall homepage tailored to each user. The algorithms update product rankings in real time based on what’s trending platform-wide.
During Singles’ Day, Alibaba’s systems even do real-time streaming analytics: they have an AI that recomposes the shopping homepage for each user on the fly depending on what deals are hot and relevant in that moment (like if athletic shoes are suddenly spiking in popularity, more shoe deals show up for shoppers interested in that category). Alibaba has claimed that its personalized recommendations and content placements significantly boost conversion and average spend, and they share some of this data with merchants via dashboards so they can see the lift in sales due to these AI features. Additionally, Alibaba’s logistics arm Cainiao uses a global dashboard to manage warehouse and delivery operations – it famously can show the journey of every package in real time.
When you’re shipping billions of items, only an AI-driven dashboard can pinpoint exceptions (like a bottleneck at one distribution center, which they then alleviate by routing new orders elsewhere). This logistics excellence ensures even with huge volume, delivery times keep improving – a critical part of customer satisfaction.
7.4 Farfetch – Luxury Omnichannel Analytics
Farfetch operates both an online marketplace and physical retail boutiques (like Browns in the UK). They’ve leaned into tech to unify these. Farfetch’s store of the future concept equips sales associates with an app that shows a customer’s online wish list and past purchases as soon as they check in or even just walk into the store (if they opt in). This is powered by a customer data dashboard that merges online and offline profiles. The associate can then personalize the store visit, pulling items that the customer has liked online. Farfetch also integrated inventory systems: a customer on Farfetch’s site can see if an item is available in a boutique near them and reserve it. All of this requires instantaneous data sync.
Farfetch’s AI capabilities also extend to a stylistic algorithm – they had a pilot using AI to create outfit combinations (which might later be recommended by human stylists). The success of such suggestions is tracked in their dashboards through engagement rates. Being a luxury platform, Farfetch is careful with AI – it’s more “assistive” than in-your-face automation. Nonetheless, by using AI to crunch numbers on what sells where, they provide their boutique partners with insights that many smaller boutiques never had access to (like, “hey, neon green bags are trending in searches this week” – so perhaps adjust store displays accordingly). This helps the whole network be more reactive to trends. Farfetch reported higher conversion rates when they rolled out these data-informed services, partly because customers feel the platform “just knows” what they like, and partly because stock is always accessible somewhere, eliminating lost sales.
7.5 Nike – “Consumer Direct” and Supply Chain AI
Nike in recent years has focused on direct-to-consumer (DTC) sales, which means mastering data from their own stores and site (as opposed to relying on wholesale partners’ data). Nike invested in advanced analytics, acquiring companies like Celect (for demand prediction) and hiring teams to build dashboards for everything from design to retail performance. One outcome is Nike’s ability to run limited drops (e.g., new sneakers on the SNKRS app) and manage the hype vs. supply carefully. Their dashboards monitor sign-ups, launch engagement, and purchasing in real time during these drops. They often sell out in minutes, but Nike captures all the data to inform the next launch – including which geographies had more demand than supply (perhaps next time allocate more there). On the supply chain front, as earlier mentioned, Nike’s AI keeps track of inventory across stores and warehouses and sends low-stock alerts.
A concrete example: during the pandemic, supply chain disruptions were rampant. Nike’s analytics allowed it to identify issues and reroute products. In one earnings call, Nike executives mentioned using “express lanes” in manufacturing to quickly replenish trending products – those decisions were data-led, focusing resources on what was hot and pulling back on what wasn’t, in near real time. They improved their full-price sell-through and reduced inventory by using data to not overproduce styles that wouldn’t sell. Moreover, Nike uses AI at the design and merchandising stage – analyzing social media, sales trends, and even weather to decide what products to make for a region. These insights are aggregated in dashboards for product teams. The result has been a reduction in products (Nike cut about 20% of its styles to focus on winners) but an increase in revenue – essentially using data to do more with less. The ability to do that cut came from identifying, through data, which long-tail products weren’t adding value and which core ones deserved more focus.
7.6 LVMH – Luxury Data Lake and Clienteling
LVMH, spanning many luxury brands, created a group-wide data platform (often referred to as a “Luxury Data Lake”) to centralize insights. One use case is clienteling: equipping sales advisors with a 360° view of a high-end client’s preferences and purchase history across brands. An advisor at Louis Vuitton could know that this client also buys Moët champagne and Dior cosmetics, informing how they approach the service. This is sensitive data, so it’s heavily consent-based and anonymized across brands, but within a brand, they have rich CRM dashboards. These dashboards in real time might show, for example, that a VIP just entered the store (via an app check-in or beacon); immediately their profile comes up on an iPad, with AI-generated suggestions of what items or even what type of conversation (style preferences, upcoming birthdays, etc.) to engage with. LVMH also leverages AI in manufacturing and supply (as noted: demand forecasting, etc.) – one case was using machine learning to optimize production scheduling for perfumes at Guerlain, which increased efficiency and cut lead times. By centralizing AI development (with the help of platforms like Dataiku), LVMH scaled these use cases across brands.
A testament to their success is that even traditionally “handcraft” oriented maisons have embraced data when they see it can uphold their luxury standards (e.g., reducing a client’s wait for a personalized product by having supply chain anticipate the order). Their Chief Data Officer has indicated that they already have 200+ AI projects in production across the group, showing how pervasive data has become even in luxury. The dashboards tying all this together ensure that from C-suite down to store manager, everyone has a pulse on performance and can say they are combining the “art” of luxury with the “science” of data.
Key Takeaway: These case studies reinforce that real-time dashboards, empowered by AI, are not theoretical – they are delivering value in practice. Companies see improvements like higher conversion rates, faster inventory turns, better customer loyalty (through personalization), and more efficient operations. Common success factors include: having the right data infrastructure (sometimes needing IoT like RFID), a willingness to let AI assist or make decisions, and an organizational culture that trusts data insights. Challenges do exist (integration, change management, data quality), but the direction is clear – those who have invested are reaping significant ROI.
8. Implementing Real-Time AI Dashboards: Best Practices & ROI Focus
For fashion retailers ready to embark on (or refine) this journey, a few best practices can ensure that the implementation of real-time dashboards is smooth and yields the expected returns:
8.1 Start with Clear KPI Targets
Before getting lost in tech, define what success looks like. Is it reducing stockouts by half? Cutting online checkout abandonment by 5%? Increasing in-season full-price sell-through? Set specific goals for key metrics. This will guide the design of the dashboard (what to prioritize) and the AI features needed (forecasting, recommendations, etc.). It also establishes a baseline to measure ROI. For example, if currently inventory accuracy is 85% and that causes 100 lost sales a week, aim to hit 98% accuracy with RFID and real-time tracking, and recoup maybe 80 of those sales. Put numbers to it. When everyone agrees on KPIs and targets, the dashboard project has direction and urgency.
8.2 Ensure Data Quality and Integration
Real-time is unforgiving – if your data is bad or siloed, a dashboard will just broadcast those issues faster. Invest in cleaning data and integrating systems: POS, e-commerce platform, warehouse management, social listening tools, etc. This might mean upgrading legacy systems or using middleware to stream data. Modern cloud data warehouses or lakes are often the backbone, with tools like Kafka for streaming data. Some retailers choose an all-in-one solution or a suite (like Salesforce Commerce Cloud + Store Cloud, which come with dashboards), others build custom. Either way, integration is key. A practical tip is to phase the integration: maybe start by linking e-commerce and warehouse data (common for BOPIS), then add store inventory, then add marketing data. At each phase, validate that the numbers make sense (e.g., reconcile daily totals with the finance system). It’s crucial to get frontline employee buy-in by showing them the dashboard numbers reflect reality – otherwise they won’t trust the alerts. Inditex’s success with RFID came after they ensured nearly 100% tag read accuracy; anything less and store managers would ignore the system as “unreliable”.
8.3 User-Friendly Design and Training
A dashboard is only as good as its adoption. Design the interface for the actual users – be it executives, planners, store managers, etc. Often this means multiple views of the data: a high-level summary for leadership, and detailed operational views for analysts. Keep it visual and intuitive – use charts, color-coded alerts, and drill-down capability. Avoid clutter; people should immediately grasp what needs attention. Once built, invest in training. Host workshops to explain each widget on the dashboard, perhaps simulate scenarios (“if you see this alert, here’s what to do”). Create an environment where employees feel the dashboard makes their job easier, not just watches over them. For instance, show store managers that a footfall counter + staffing suggestion actually leads to smoother operations on a busy Saturday – they’ll become advocates. Some companies assign “data champions” in each team to encourage use and gather feedback. Additionally, maintain documentation and an FAQ, as well as support (maybe a Slack channel for questions about the dashboard). The goal is to embed the dashboard into daily routines: the morning huddle starts with it, or a store lead checks it on an iPad every few hours to plan restocks.
8.4 Phased Automation – Trusting AI Step by Step
It’s wise not to flip the switch to full automation on day one. First, let the AI observe and recommend while humans still make the final call (“human in the loop”). For example, an AI might flag 5 products for markdown this week and suggest percentages; the merchandising team reviews those suggestions in the dashboard and approves or tweaks them. Track the outcomes. If over time the team sees the AI is right 9 out of 10 times, their confidence builds. Then you can automate the simpler decisions (maybe AI can auto-markdown items under a certain price or quantity threshold, while humans handle bigger decisions). This gradual approach reduces risk and builds trust. The dashboard can facilitate this by showing AI’s “reasoning” – like annotations on alerts (“Predicted stockout in 3 days based on 4-week sales trend”). When people understand why, they’re more likely to agree. Also, put safety nets: set boundaries for AI actions (e.g., don’t discount beyond 30% without approval, or don’t transfer more than X units between stores). As comfort grows, these can be adjusted. Remember, the goal is not to replace human judgment but to enhance it and free it for higher-level strategic thinking. Over time, teams often realize the AI frees them from firefighting mundane issues so they can focus on product development, marketing creativity, supplier relationships, etc. Highlighting those wins in internal communication will further drive adoption.
8.5 Monitor ROI and Iterate
After implementation, continuously measure against the baseline. Did checkout conversion improve? Did average fulfillment time shorten? Calculate the financial impact: e.g., 3% conversion increase = $Y million more sales a month. Compare that to costs of running the system. Often, initial ROI can be achieved in months if a glaring issue was fixed (like preventing stockouts of a popular item). Other benefits are cumulative – fewer markdowns over a season = improved margins, which show up in quarterly results. Present these wins to stakeholders to keep momentum (and funding) going. But also examine where things didn’t move as expected. Maybe the AI forecasts are still off for certain product categories – investigate why (need another data input? model tweak?). Use the dashboard’s own data to improve itself: e.g., if users frequently ignore certain alerts, find out if those alerts are not useful or if they’re being dispatched to the wrong people/time. Essentially, treat the dashboard as a product that evolves. Retail is dynamic; KPIs might change (a new omni-channel initiative might require adding “Instagram Live conversions” as a metric). Be ready to update the system. Many companies do quarterly reviews of their dashboards – adding/removing metrics, refining thresholds, and checking alignment with business goals. It’s also smart to stay informed on new tech: perhaps integrating a new AI tool for image-based trend detection or a new data source (like a fashion trend index) could further enrich the insights. The best implementations are living programs, not one-off IT projects.
8.6 Partner with Experts (if needed)
Not every retailer has a big data science team. This is where consultants or vendor solutions come in (like HI-GTM, for example, as an expert consultant in our context). Engaging experts can accelerate the process and avoid pitfalls, because they bring cross-industry learnings. They can provide pre-built KPI models tailored for fashion, and help customize them to the retailer’s unique needs. They also assist in change management, which is often the hardest part. The cost of bringing in expertise is usually far outweighed by faster ROI and fewer mistakes. Many top retailers use a mix: in-house teams plus external specialists for certain components (like a specialized AI for sizing recommendations, or a plug-in for social analytics). The key is to ensure all parts integrate into the unified dashboard so users don’t have to jump between tools.
Finally, let’s talk ROI to convince any skeptics: When done correctly, real-time AI dashboards have proven to increase sales 5-15% through better conversion and fewer stock issues, improve margins 2-10% via smarter pricing and reduced markdowns, and enhance customer retention (often measured via NPS or repeat purchase rate) thanks to more personalized service. They also can reduce operating costs by optimizing labor scheduling (matching staff to peak times), lowering expedited shipping needs (because stockouts are prevented), and automating tasks that took manual hours (reports, basic analysis). These are significant numbers in retail, where net profit margins are usually thin – meaning these improvements directly bolster the bottom line or provide reinvestment capital. Perhaps equally important, they future-proof the business. In a world where consumer expectations and market conditions can flip overnight, having a responsive, data-driven culture isn’t just advantageous – it’s increasingly necessary for survival.
In Conclusion: Real-time performance dashboards powered by AI are revolutionizing how fashion retailers operate, from high-level strategy down to daily tweaks. They bring clarity, speed, and intelligence to decision-making, aligning everyone in the organization to act on the most current information. The leading fashion companies we’ve discussed are already enjoying these benefits – reacting to trends faster, delighting customers with timely offerings, and squeezing efficiency out of every process. The roadmap is clear for those who wish to follow: integrate your data, define your key metrics, infuse AI where it counts, respect regional nuances, and foster a company culture that embraces data. Do this, and the payoff will be seen in both your financial metrics and customer loyalty. In the fast fashion (and luxury) world, the proverb “time is money” has never been more literal – real-time insight is now directly convertible to revenue and profit. The tools are ready; it’s up to retailers to use them and stitch data into the fabric of their business success.
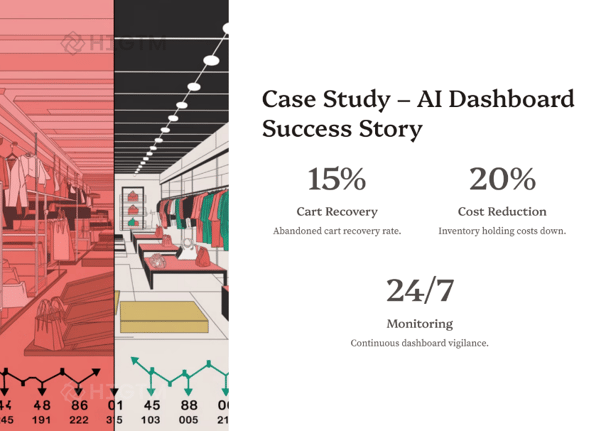
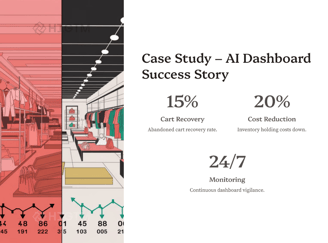
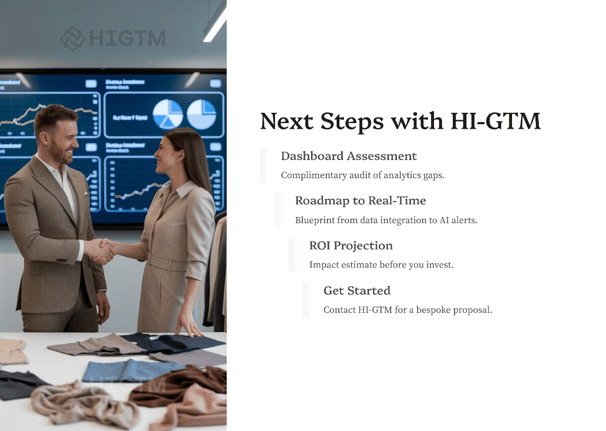
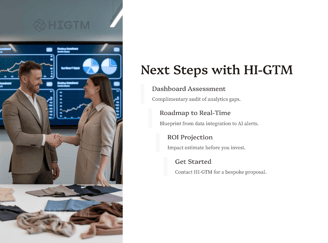
Turn AI into ROI — Win Faster with HIGTM.
Consult with us to discuss how to manage and grow your business operations with AI.
© 2025 HIGTM. All rights reserved.