68. KPIs for AI Implementation in Retail: Tracking ROI & Outcomes
AI promises transformation – but only if we can measure its impact. This article explores how leading retailers in a specific segment (mass-market and grocery retail) track ROI through well-chosen KPIs. We break down key metrics across supply chain, customer experience, marketing, and in-store operations. Decision-makers will learn which KPIs matter, how North America and Europe differ, and best practices to ensure AI delivers financial, operational, and customer-focused results.
Q1: FOUNDATIONS OF AI IN SME MANAGEMENT - CHAPTER 3 (DAYS 60–90): LAYING OPERATIONAL FOUNDATIONS
Gary Stoyanov PhD
3/9/202520 min read

1. The Surge in the Current Landscape
AI adoption in retail is surging, from warehouse robots and predictive analytics to personalized marketing and smart checkouts. Yet one question echoes in boardrooms: “What’s the ROI?” Forward-thinking retailers know that launching AI initiatives without clear KPIs is like flying blind. In this guide, we detail the key performance indicators (KPIs) that top retailers track to ensure their AI implementations drive real ROI. The focus is on mass retail (e.g., grocery and general merchandise), drawing examples from industry leaders like Walmart, Amazon, Carrefour, and Target. We’ll cover supply chain, customer experience, marketing, and in-store automation – highlighting financial, operational, and customer-centric metrics. (Let’s dive straight into the metrics that matter.)
2. Supply Chain & Logistics KPIs for AI ROI
2.1 Inventory Optimization & Forecast Accuracy
Supply chain is often where AI delivers its first big win. Retailers use AI-driven demand forecasting to optimize inventory levels and reduce stockouts. A crucial KPI here is forecast accuracy (or its inverse, forecast error). McKinsey research shows that AI-based forecasting can improve accuracy by 10–20%, reducing errors by up to 50%.
This directly translates to higher ROI: fewer stockouts means more sales captured and less excess means lower holding costs. For example, Danone’s AI demand model led to a 30% reduction in lost sales by better aligning production with actual demand.
Retailers track stock-out rate (percentage of items out-of-stock) and inventory turnover (how quickly stock is sold) as KPIs. An AI that raises forecast accuracy will typically lower stock-out rates and increase turnover, improving both revenue and cash flow.
2.2 Logistics Cost & Efficiency Metrics
AI optimization doesn’t stop at the shelf – it extends through logistics networks. Key KPIs include logistics costs (as % of sales or per unit shipped) and on-time delivery rate. Early AI adopters have seen striking improvements: according to industry data, AI-driven supply chain planning yielded about a 15% reduction in logistics costs on average for front-runners.
These cost savings come from smarter route planning, load optimization, and predictive maintenance on fleets. Another vital KPI is order lead time (time from order to delivery). AI systems that anticipate demand can stage inventory closer to customers and allocate orders optimally, cutting delivery times. Retailers in North America often emphasize speed (same-day delivery rates, etc.), while in Europe cost-efficiency might be equally prized due to complex multi-country supply networks.
2.3 Warehouse Automation & Productivity
Inside the warehouse, AI and robotics drive productivity gains. KPIs such as units picked per hour, order accuracy rate, and warehouse throughput measure the impact. Amazon famously uses robotic automation (like Kiva robots) – these have increased storage density and enabled processing of more orders per hour, contributing to Amazon’s ability to scale during peak periods. While exact figures are proprietary, industry estimates suggest 50% improvements in throughput are achievable in automated warehouses. Retailers also measure labor cost per order – automation should reduce this by handling menial tasks. Walmart, for instance, introduced autonomous cleaners and shelf scanners in stores; a similar principle in warehouses frees workers from repetitive scanning and packing tasks. The ROI is tracked in labor hours saved and reallocated to value-added work. When one major retailer deployed AI robotic systems, they noted they could handle 25% more orders with the same staff, a clear efficiency KPI indicating ROI through output gains.
2.4 Fulfillment & Service Level KPIs
Ultimately, supply chain AI is judged by service levels – the ability to get the right product to the right place on time. A common metric is Order Fill Rate (percentage of customer orders fulfilled completely). Leading retailers target near 98-99% fill rates; AI helps by predicting demand and positioning inventory. A McKinsey study highlighted that companies effectively using AI in supply chain saw service level improvements up to 65%.
This huge jump means far fewer missed sales and stockouts. Retailers will also track OTIF (On-Time, In-Full) delivery as a KPI, ensuring that AI doesn’t just optimize cost at the expense of reliability. The balance of cost vs. service is where AI shines – by analyzing vast data (weather, traffic, trends), AI helps achieve high service at lower cost. By monitoring these KPIs, retailers confirm that their AI investments (like advanced forecasting software or dynamic routing systems) directly contribute to better supply chain performance and ROI.
3. Customer Experience & Engagement KPIs
3.1 Personalization Effectiveness Metrics
In the battle for customer loyalty, AI-powered personalization is a potent weapon. Retailers implement AI recommendation engines and personalization algorithms online and in-app, aiming to boost sales and satisfaction. The key KPIs to watch: conversion rate, average order value (AOV), and customer lifetime value (CLV). When personalization is effective, conversion and AOV go up. For example, HP Tronic (an electronics retailer) used AI to personalize web content and saw a 136% increase in conversion rate for new customers.
Similarly, AI-driven recommendations can increase basket sizes by suggesting relevant add-ons – Amazon credits 35% of its revenue to its recommendation engine, a staggering proof point. Fast-growing retailers gain 40% more revenue from hyper-personalization compared to their slower-growing peers. To track ROI, companies compare sales from personalized campaigns vs. non-personalized (control group testing). Retention rate is another KPI: personalization should make customers feel understood, increasing repeat purchase rates.
3.2 Customer Satisfaction & Loyalty KPIs
AI impacts softer metrics that have hard financial consequences – namely customer satisfaction and loyalty. Retailers monitor CSAT (Customer Satisfaction) scores and NPS (Net Promoter Score) as KPIs to gauge experience quality. AI can improve these by speeding up service and tailoring interactions. One example: chatbots and virtual assistants. Modern AI chatbots provide instant answers and 24/7 support. The KPIs here include First Response Time (often near zero with bots) and Resolution Rate. Best-in-class AI chatbots now achieve 60-90% inquiry resolution without human help. In fact, after optimizing its AI chatbot, one company resolved 96% of interactions via bot with a 97% CSAT – virtually every customer left happy without needing a live agent. These satisfaction metrics tie to ROI because happier customers are loyal customers. Loyalty program engagement is another metric: AI helps personalize loyalty rewards and offers. Carrefour’s AI-driven loyalty gamification (the “Challenge” program) customizes offers for each shopper, aiming to boost engagement. KPIs measured are active loyalty members, redemption rates of offers, and frequency of visits. Early results from such programs show double-digit increases in engagement, which ultimately lead to higher lifetime value.
3.3 Engagement & Retention Metrics
Beyond single transactions, AI influences long-term engagement. Retailers track customer retention rate and churn rate as high-level KPIs. If AI-driven improvements (better recommendations, proactive service via AI, personalized content) are working, retention should increase and churn decrease. For instance, if a retailer’s loyalty app uses AI to send timely personalized deals, they might monitor a cohort’s 90-day repeat purchase rate before vs. after AI implementation. Another engagement KPI is time spent on site/app – relevant for e-commerce – which can rise if AI is making the experience more relevant and enjoyable. Email click-through and conversion can be measured when AI personalization is applied to marketing communications: UK retailer DFS saw a 4.2% conversion lift and 3.9% revenue uptick by using AI to trigger personalized emails based on customer behavior.
All these engagement metrics feed into the ROI story by indicating stronger customer relationships that drive revenue over time. In North America, where customer loyalty can be fickle, retailers place heavy emphasis on these KPIs to ensure AI efforts translate into brand preference. In Europe, engagement is also key, though privacy regulations mean AI initiatives like personalization must be done with careful data consent (which is itself a KPI to monitor – percentage of customers opted in to data sharing).
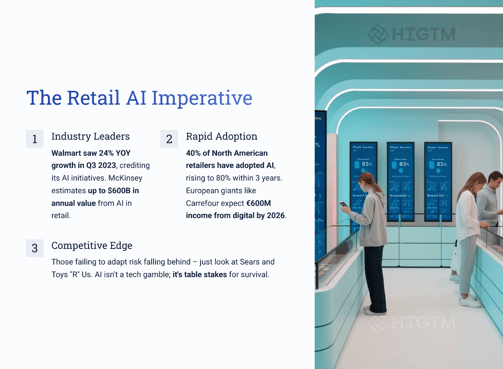
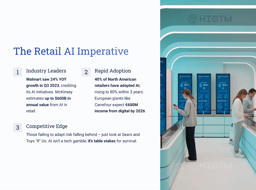
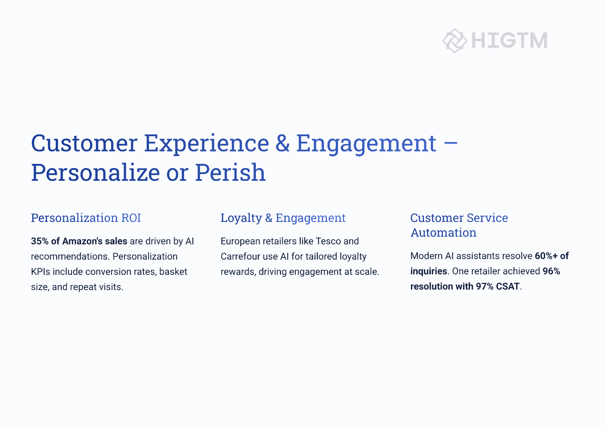
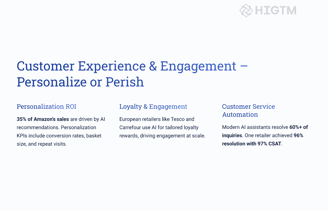
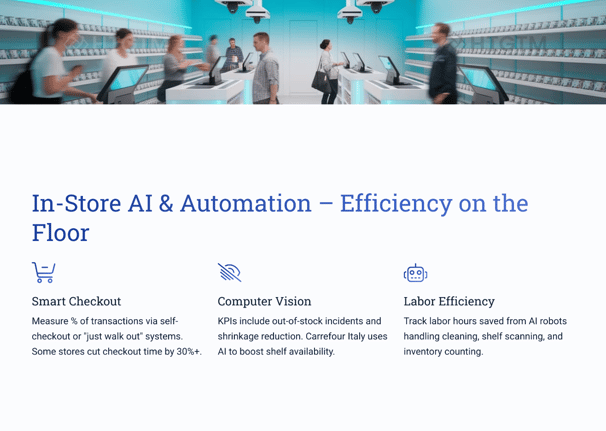
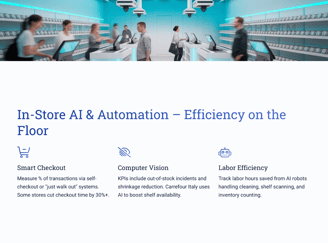
4. Marketing & Sales Performance KPIs
4.1 Campaign Conversion & ROI
Marketing is an area where AI’s impact is immediately quantifiable. Retail marketers leverage AI for customer segmentation, predictive analytics, and even generative content – and they measure outcomes with standard marketing KPIs supercharged. Conversion rate (of campaigns or channels) is primary. AI-driven targeting can dramatically improve conversions by matching offers to likely buyers. We saw earlier that personalization on web and email channels boosts conversion percentages significantly.
Retailers also track Click-Through Rate (CTR) for AI-personalized ads versus generic ads. One case: Rapha (a cycling apparel brand) used AI to personalize Facebook ads and achieved a 31% increase in purchase events from those ads.
That’s a direct lift in campaign effectiveness attributable to AI. Another KPI is Return on Ad Spend (ROAS) or marketing ROI – essentially revenue gained per dollar of marketing spend. AI can optimize media buying and audience targeting to get more bang for the buck. According to McKinsey, companies using advanced personalization get 5 to 8 times the ROI on marketing spend compared to those that don’t personalize well – a huge multiplier that any CMO would celebrate. Tracking ROAS pre- and post-AI implementation is a clear way to see if the AI is making a difference in the bottom line.
4.2 Sales Uplift & Attribution
When AI is used in sales or merchandising (for example, dynamic pricing, product assortment optimization, or guided selling tools), retailers look at overall sales growth and attribution. Year-over-year sales growth in categories where AI is applied is one indicator (like Walmart’s 24% YOY growth in 2023, which leadership partially credited to AI initiatives in personalization and supply chain). But more rigorously, uplift analysis is used: if a predictive model identifies micro-segments and tailors offers, what incremental sales were generated in those segments vs. control groups? AI’s contribution can be isolated via A/B tests or holdout groups. Retailers also employ attribution models that include AI touchpoints (for instance, if an AI chatbot assisted a customer, credit a portion of the sale to that interaction).
A concrete example: Amazon’s famous recommendation engine – by attributing 35% of sales to it, Amazon effectively quantifies the ROI of their AI-driven cross-sell strategy (billions in revenue that likely wouldn’t happen without those algorithms). Brick-and-mortar sales KPIs can also be influenced by AI: think of store conversion rate (visitors who make a purchase). If AI-powered clienteling apps or interactive displays are added in stores, did conversion or average spend increase? These are measured through pilot programs. In Europe, where omni-channel integration is key, retailers measure how AI-driven online recommendations drive in-store sales (and vice versa), requiring robust multi-channel attribution KPIs. Overall, tying specific sales growth to AI initiatives builds the business case for further investment.
4.3 Customer Acquisition Cost & CLV
Two higher-level metrics round out marketing/sales KPIs for AI: Customer Acquisition Cost (CAC) and Customer Lifetime Value (CLV). A successful AI marketing program can lower CAC by targeting the right prospects and automating lead qualification. For example, if AI helps find more lookalike high-value customers, the marketing spend per new customer should drop, or the quality (and thus CLV) of acquired customers should rise. Retailers will watch CAC trendlines after deploying AI in ad buying or personalization – a decline in CAC is a positive ROI signal, meaning less spend for equal or better results.
CLV, on the other hand, should increase if AI is keeping customers more engaged and loyal over time (as discussed in section 3). AI-driven upselling and cross-selling also boost CLV by increasing each customer’s spend. By modeling CLV for cohorts that receive AI personalization vs. those who don’t, retailers can quantify how much more value AI-supported customers are generating. These metrics often resonate with executives: for instance, if AI personalization costs $1M per year and it increases CLV by 10%, that could translate to $50M in extra value from the customer base – a clear ROI win when presented in financial terms. Thus, CAC and CLV act as composite KPIs that reflect the cumulative impact of AI on marketing efficiency and customer loyalty.
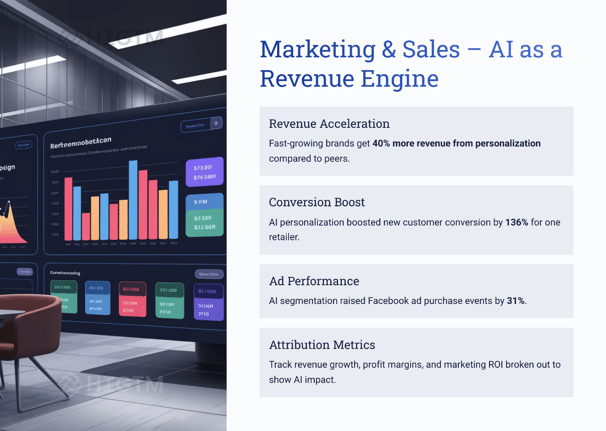
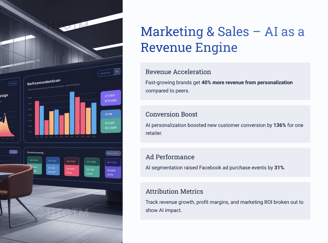
5. In-Store AI & Automation KPIs
5.1 Smart Checkout & Store Efficiency
Physical retail is embracing AI to create smarter stores. One prominent innovation is smart checkout – whether self-checkout kiosks, “scan & go” mobile apps, or fully automated checkout-free stores (like Amazon Go). Key KPIs to gauge ROI here include checkout adoption rate (what % of transactions use the AI-enabled method), average checkout time, and customer wait time. Shorter lines and faster checkout are proven to improve customer satisfaction and can increase sales (customers who get out faster might be willing to shop more often). Retailers have reported significant efficiency gains: for example, when a large retail chain introduced scan-and-go mobile checkout, it observed a reduction in average checkout duration by ~30%, and tracked an uptick in store NPS because customers appreciated the convenience. Another KPI is transaction throughput per hour per lane – AI systems can handle more transactions than human cashiers especially during peak times, which is measurable. Labor cost savings can also be calculated: fewer cashiers needed at peak (redeployed elsewhere in store) means cost per transaction falls. However, it’s important to also monitor shrinkage (loss/theft) in these models, as checkout-free can introduce risks – AI computer vision should keep shrink in check, and that shrink rate is absolutely a KPI to monitor to ensure ROI isn’t lost on the back end.
5.2 Shelf Availability & Merchandising KPIs
AI-powered cameras and robots are increasingly used to monitor shelf stock and compliance in real time. The KPI here is on-shelf availability – the percentage of the time products are available for sale on the shelf. Traditional manual checks might yield 90-95% availability; AI systems aim to push this higher by catching stockouts fast. If an AI camera system alerts staff to restock an item within minutes, the duration of stockout events (another KPI) plummets. Retailers can calculate the sales saved by this improved responsiveness. One European grocer using AI shelf monitoring found that they could reduce stock check labor hours by 60% and improve sales on promoted items (which were often running out) by a significant margin once they ensured shelves stayed full. Planogram compliance (are products displayed as they should be?) is a softer metric that AI can track via image recognition; improved compliance can correlate to sales lift, and some retailers treat this as a KPI for store execution quality. AI also aids dynamic merchandising – e.g., electronic shelf labels that change price based on AI algorithms. The KPIs for those include price change frequency and margin impact (did AI-driven price adjustments increase overall margin or revenue?). In France, Carrefour’s experiments with AI pricing and promotions, in partnership with tech firms, are closely watched with KPIs like promo sell-through rates and margin percent. All these measures help determine if in-store AI tech is delivering ROI through better sales and leaner operations.
5.3 Workforce Productivity & Cost Optimization
In-store AI and automation also affect the workforce. While these technologies can reduce labor needs for certain tasks, leading retailers often reinvest those hours into customer-facing service. Key operational KPIs here include hours of labor saved per week through automation and task productivity (e.g., how many square feet can be cleaned or how many items audited per hour by AI vs by a human).
Walmart’s use of robotic floor cleaners is a good example: each robot can clean store floors nightly without supervision, effectively saving several hours of janitorial work each day – Walmart would track the number of hours saved and the equivalent labor cost. Across hundreds of stores, that becomes a massive efficiency gain. At the same time, customer service metrics might improve if associates are freed up to help shoppers instead of doing routine tasks. So we see a link between productivity KPIs and customer KPIs – for instance, measure if staff-to-customer interaction time increased due to task automation (which could then reflect in CSAT scores). Another financial KPI is store operating cost as % of sales – if AI automation can shave this percentage (via energy management systems, optimized staffing, etc.), it’s captured in quarterly results. Retailers in North America and Europe alike are grappling with labor shortages and cost pressures, so these AI-driven productivity KPIs are critical.
Additionally, as Europe has higher labor costs in many countries, the ROI from labor-saving automation can be even more attractive – a fact that European retailers consider when looking at AI investments. They do so while being mindful of regulations and public sentiment around automation; hence, tracking employee satisfaction alongside productivity can be wise (ensuring the human workforce is augmented, not alienated, by AI). Ultimately, if AI can maintain or improve store performance with fewer or reallocated staff hours, the ROI is captured in both cost savings and potentially improved sales per labor hour – a win-win that the KPI dashboard will make evident.
6. Financial, Operational, and Customer Metrics Alignment
A successful AI implementation in retail doesn’t focus on a single metric; it aligns financial, operational, and customer-centric KPIs to paint a full ROI picture. Let’s break down these categories and how they interlink:
Financial Metrics: These are the end-game for ROI. KPIs include revenue growth, gross profit, operating margin, and cost savings attributed to AI. For instance, if an AI pricing tool improves margins by 0.5% across thousands of items, that’s a direct financial gain to monitor. ROI (%) itself is tracked by comparing the monetary benefits (increased revenue, cost reduction) to the investment and running cost of the AI solution. Many retailers set targets like “AI initiative X must deliver 3x ROI within 2 years.” Financial metrics also extend to capital efficiency – e.g., the reduction in inventory (35% in some cases) frees up cash, improving return on capital. Carrefour’s strategic plan explicitly expects €200M incremental operating income from data & retail media initiatives by 2026 – a financial ROI target for their AI-driven data monetization. These hard dollar metrics ensure the AI strategy is contributing to the bottom line and shareholder value.
Operational Metrics: These KPIs measure how AI improves internal processes and efficiency. They often underlie the financial outcomes. Examples we discussed include forecast error rate, order fulfillment time, employee productivity, automation rate (% of tasks or decisions automated by AI), and supply chain agility. An interesting operational metric is time to decision – how quickly can the business respond to changes or opportunities with AI aid? AI analytics might reduce a merchandising decision cycle from weeks to days, which is a competitive edge. Retailers also measure system uptime and algorithm performance (technical KPIs) for AI systems, ensuring they operate reliably. These operational KPIs are important for North American retailers dealing with huge scale (they need AI to keep operations lean across thousands of stores), and for European retailers aiming for excellence in complex multi-national operations. All operational improvements eventually reflect in cost or service, but tracking them directly helps teams fine-tune AI systems. For example, if an AI picks 99.5% of e-commerce orders correctly (order accuracy KPI), that reduces re-shipping costs and improves customer satisfaction – connecting operations to both financial and customer metrics.
Customer-Centric Metrics: These KPIs evaluate how AI impacts the customer’s experience and relationship with the brand. We’ve covered CSAT, NPS, retention, engagement rates, conversion rates, personalization relevance scores, etc. In ROI terms, these can be translated into customer lifetime value and churn reduction. Many leading retailers correlate an increase in NPS to revenue – for instance, one study suggests a 7% rise in NPS can correlate to 1% revenue growth.
So if AI-driven personalization or service improvements boost NPS by, say, 14 points, a retailer might expect ~2% growth in revenue as an outcome. Customer metrics are particularly vital in saturated markets: North American retailers use them to differentiate on experience (e.g., how much did AI improve our mobile app rating or in-store experience survey scores?), whereas European retailers operate in markets with strong consumer protections and loyalty traditions, so improving customer trust and satisfaction via AI (without being intrusive) is key. An example of a customer-centric metric in Europe could be data privacy trust score – ensuring customers are comfortable with AI usage. Aligning customer KPIs with AI initiatives ensures technology serves people, not just processes. When these metrics move in the right direction, they often herald long-term financial gains.
The magic happens when retailers align all three areas: For example, implementing an AI chatbot might initially be justified operationally (reduce call volume by 40%), but the team should also measure customer impact (CSAT with chatbot vs phone) and financial impact (support cost per contact). If all three metrics show positive results – say costs down 20%, same CSAT, and agents can handle more complex sales calls leading to some revenue uptick – then the AI implementation is a clear winner.
This alignment is at the heart of an effective AI KPI framework, ensuring a balanced scorecard approach. HI-GTM often helps clients map each AI use case to at least one financial, one operational, and one customer KPI to get a 360° view of ROI.
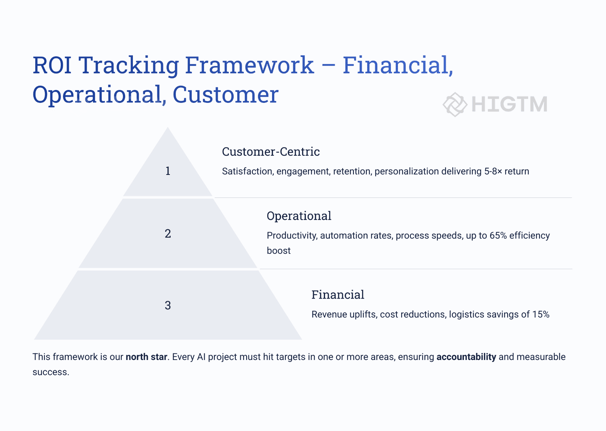
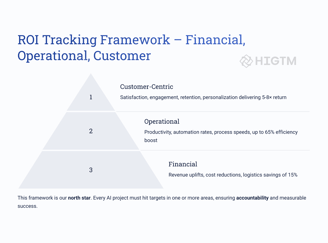
7. Regional Trends and Regulatory Considerations (North America vs. Europe)
Retailers in North America and Europe share the goal of AI-driven ROI, but their approaches can differ due to market conditions and regulatory landscapes. North America – led by the U.S. – has seen aggressive AI adoption in retail, with companies like Amazon and Walmart investing heavily in proprietary AI systems. The trend is towards bold experimentation and scaling what works. Metrics in NA often emphasize growth and efficiency in large, homogenous markets. For instance, U.S. retailers might prioritize same-day delivery coverage or digital sales penetration as KPIs accelerated by AI (Amazon’s logistics AI enables its Prime one-day delivery, a key competitive metric).
The regulatory environment in the U.S. is relatively permissive, allowing extensive use of consumer data, which fuels AI personalization. However, even in NA, there’s increasing attention to AI ethics and data privacy (e.g., California’s CCPA law), so retailers track compliance KPIs – such as percentage of models passing bias tests or adherence to privacy opt-outs – to avoid regulatory or PR issues that could erode ROI.
In Europe, adoption is strong but slightly more cautious. The EU’s GDPR (General Data Protection Regulation) imposes strict rules on personal data usage. This means European retailers must ensure AI systems, especially those dealing with customer data (recommendations, marketing AI, facial recognition in stores, etc.), are compliant. As such, a unique KPI for AI projects in Europe could be GDPR compliance status or number of privacy breaches (aiming for zero).
If an AI initiative cannot guarantee privacy, it might not launch at all. European retailers, like Carrefour and Tesco, focus on AI in areas like supply chain, pricing, and loyalty – where they can leverage first-party data safely. They also prepare for the EU AI Act in the pipeline, which may categorize some retail AI (like biometric identification or credit scoring for financing) as “high risk” requiring special monitoring. KPIs may thus include AI risk assessments performed or explainability scores for AI models (ensuring algorithms can be explained as required by regulators).
Market-wise, European retail often has tighter margins and higher labor costs, making cost-saving AI (automation, optimization) very attractive. A French hypermarket chain might measure the reduction in food waste achieved by AI ordering systems as a KPI – both a cost saver and a sustainability win, which is important in Europe.
North American retailers, while also concerned with efficiency, might lean more into growth metrics – using AI to capture market share through omnichannel services, measuring things like online/offline integration scores or net new customers from AI-driven campaigns. Culturally, European consumers are sometimes more skeptical of AI (e.g., resistance to fully automated stores has been noted), so KPIs around customer acceptance of AI features might be tracked (for example, usage rate of an AI self-checkout vs traditional in a European store, to ensure customers are embracing it).
Despite differences, there’s convergence: both regions celebrate wins like 15% logistics cost reduction, 35% inventory reduction through AI – those seem universal.
Both care about customer-centric metrics – a UK retailer values NPS improvements from AI just as much as a US retailer does. The key is that regional context informs KPI targets and constraints. An AI rollout in Germany will bake in data protection compliance as a success criterion, whereas one in the US might focus more on speed to market. Retailers operating globally actually set KPIs region by region: e.g., tracking AI-driven sales lift in North America vs. Europe separately, to account for different baselines and growth potentials. Overall, the North America vs. Europe nuance ensures that our KPI framework remains flexible and locally relevant – hitting ROI targets while respecting each market’s rules and customer expectations.
8. Best Practices for Tracking AI ROI in Retail
Setting up KPIs is one thing; effectively tracking and acting on them is another. Leading retailers follow several best practices to make sure KPI tracking leads to ROI realization:
Define KPIs from Day 1: Before an AI project kicks off, successful teams define what success looks like in numbers. Is it a 2% sales increase in a category? A $5M cost saving in distribution? By clearly stating KPIs and ROI goals upfront, everyone aligns on purpose. For example, Target, before implementing an AI for supply chain, might set a goal like “reduce excess inventory by $X million in the first year” – a crisp target to aim for.
Baseline and Benchmark: Always capture the baseline performance before AI changes things. If your average forecast error was 25%, and after AI it’s 15%, you have a clear KPI improvement of 10 percentage points due to AI. Benchmarks can also be external – knowing industry averages (say, **60% chatbot deflection rate is world-class) helps set ambitious but realistic KPI targets.
Use Dashboards and Real-Time Monitoring: Retail moves fast, and so should your KPI tracking. The best retailers invest in live dashboards where KPI data from AI systems and traditional systems converge. A merchandising manager can see yesterday’s sales lift from the AI pricing tool, while an operations director can see last week’s fulfillment cost per order. Real-time tracking allows for rapid adjustments. If a KPI isn’t moving as expected, teams can investigate if the AI model needs retraining or if there’s an external factor at play.
Attribution and Causation Analysis: One challenge is ensuring the KPI changes are truly due to AI and not other factors. Best practice is to run controlled experiments (A/B tests, regional rollouts) and use statistical attribution. For instance, roll out an AI recommendation engine on the website for 50% of traffic and compare the conversion rate to the other 50%. If the test group’s KPI is significantly higher, you’ve proven AI’s impact. This rigor strengthens the ROI case to executives. Walmart has been known to pilot AI solutions in a set of stores and compare against control stores to validate KPI improvements before scaling.
Continuous Improvement Cycle: AI doesn’t set-and-forget, and neither does KPI management. As AI models run, feed the KPI results back into improvements. If an AI forecasting model consistently misses the mark on certain items (KPI: forecast error by category), the team refines the model or adds new data sources. This is the virtuous cycle: measure → learn → improve → measure again. Many retailers schedule quarterly AI performance reviews, just like business reviews, focusing on KPI trends and the next tweaks to keep ROI growing.
Cross-Functional Accountability: KPIs should be transparent and discussed across teams. AI ROI tracking isn’t just an IT or data science responsibility – store operations, marketing, supply chain, finance, all stakeholders should see the metrics. Some organizations form an “AI Steering Committee” that reviews KPI dashboards monthly. This ensures accountability (if a metric lags, who will take action?) and also helps surface success stories (for example, the marketing team sees supply chain’s success with AI and gets inspired to apply AI in their domain, and vice versa).
Human in the Loop & Training: An often overlooked practice is training employees to work effectively with AI and interpret KPI results. If store managers get a new dashboard of AI-driven stock recommendations, they need to know how to react to those and also how to report issues. Encouraging feedback from the field (e.g., staff noting if an AI suggestion didn’t work as expected) can be captured as a qualitative KPI (like “% of AI suggestions accepted by stores”). High acceptance can be a success metric; low acceptance might indicate trust issues or model problems. By tracking and addressing this, retailers ensure the AI truly delivers ROI on the ground.
Maintain Customer Trust: This is more of a principle, but it’s measured via some KPIs we discussed (NPS, opt-out rates, etc.). Especially relevant in AI personalization and automation that’s customer-facing: retailers must monitor if any AI usage is inadvertently harming customer perception. For example, if an AI scheduling system overly optimizes staffing and a store ends up under-staffed at times, customer experience might dip. So tying operational KPIs to customer outcomes (perhaps correlating staff hours vs. CSAT) can catch optimizations that go too far. Responsible AI deployment sustains long-term ROI by keeping customers (and employees) supportive of innovation.
By following these best practices, retailers create a robust environment where AI-driven KPIs are continuously tracked and acted upon. It turns KPI measurement from a passive reporting exercise into an active management tool. The payoff is clear: initiatives stay on track, ROI shortfalls are corrected early, and successes are scaled quickly. This discipline in tracking and optimizing is what separates AI leaders from the rest. It’s not that their algorithms are magically better – it’s that they manage them better, with KPIs as the guide.
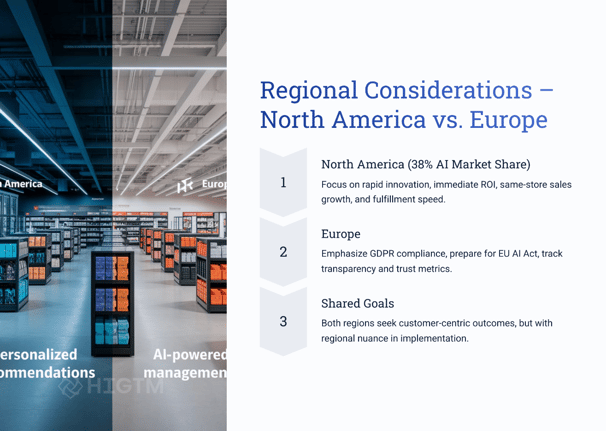
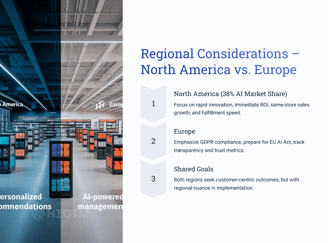
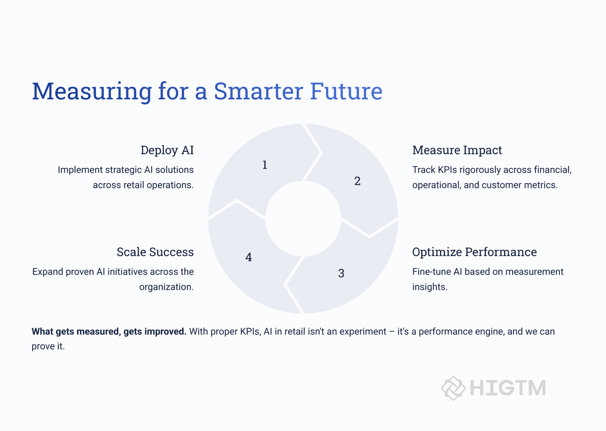
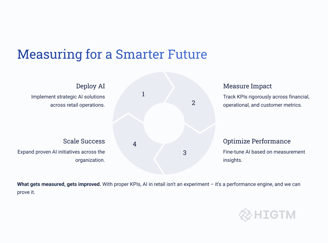
9. Conclusion: AI can deliver substantial ROI
The retail industry segment we explored – large-scale retailers, especially in grocery and general merchandise – demonstrates that AI can deliver substantial ROI across the value chain. But the common thread among successful AI adopters is a relentless focus on KPIs and outcomes. From Walmart’s supply chain triumphs to Amazon’s personalized recommendations, every example shows measured improvements: percentage points off costs, percentage points onto conversion rates, basis points added to profit margins, minutes shaved off processes, and smiles added to customers’ faces. North American and European retailers alike are proving that if you track it, you can improve it.
For retail executives, the takeaway is clear: define what success means in concrete terms before investing in AI. Use a comprehensive set of KPIs spanning financial results, operational efficiency, and customer experience. Monitor those metrics like a heartbeat. In doing so, you ensure that AI isn’t just an experiment or a trendy project – it becomes a performance engine for your business.
In a world of tight competition and fast-changing consumer behavior, this ability to implement AI and immediately see where and how it moves the needle is a true competitive advantage. It brings clarity to decision-making and confidence to double-down on what works. As HI-GTM often advises clients: “Don’t deploy AI for AI’s sake – deploy it for ROI.” And ROI must be evidenced by the numbers.
The coming years will see even more AI in retail – from generative AI designing marketing content to advanced robotics in stores. The specifics will evolve, but the principle of rigorous KPI tracking will remain the compass that keeps these innovations aligned with business goals. Retailers who master this will not only achieve impressive ROI on their AI investments but will also build agile, data-driven cultures ready to adapt to whatever the future holds. In the grand theater of retail, AI is a star – but the spotlight belongs to results. By tracking KPIs diligently, we ensure that every AI initiative ends in an encore of success that we can quantify and celebrate.
Turn AI into ROI — Win Faster with HIGTM.
Consult with us to discuss how to manage and grow your business operations with AI.
© 2025 HIGTM. All rights reserved.