59. Chapter 2 Wrap-Up – AI Adoption for SMEs: Consolidating Data and Technology Readiness
In Chapter 2: Consolidating Data & Tech Readiness, we explore how building a solid data foundation and upgrading your technology can make or break your AI ambitions. This executive-focused wrap-up highlights real-world insights and practical steps for business leadersThis article explores a real-world case study of a manufacturing SME that successfully implemented predictive maintenance. We will delve into the specific business context of the company, the technologies and vendors they used, how they rolled out the solution, and the tangible business impacts (including ROI). Additionally, we’ll discuss cost considerations relevant to SMBs contemplating a similar move. If you’re a business executive or SMB owner looking to leverage AI-driven predictive maintenance, read on – this case offers valuable insights into both the promise and practicalities of implementation.
Q1: FOUNDATIONS OF AI IN SME MANAGEMENT - CHAPTER 2 (DAYS 32–59): DATA & TECH READINESS
Gary Stoyanov PhD
2/28/20259 min read
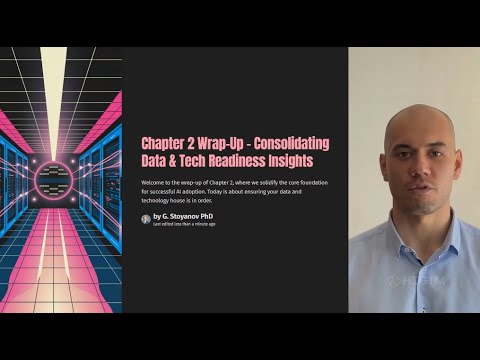
1. Consolidate Data into a Single Source of Truth
1.1 Identify Your Data Silos and Pain Points
Chances are your data is scattered across multiple systems – a CRM, an ERP, various spreadsheets – each acting as an isolated silo. The first step is to pinpoint these silos and the problems they cause. Are sales and marketing using different customer lists? Does finance trust a different figure for “revenue” than operations? Map out where data lives and where discrepancies or duplicate efforts occur. By illuminating these pain points, you can prioritize which silos to integrate first. Target the areas causing the biggest headaches or confusion early on to build momentum with quick wins.
1.2 Integrate Data with the Right Tools and Platforms
Next, work toward a single source of truth – a central database or seamlessly connected system that everyone draws from. Today you don't need to build this from scratch: cloud data warehouses like Snowflake or Google BigQuery and integration tools such as Microsoft Azure Data Factory or AWS Glue can automatically combine data from different sources. The goal is one reliable place for key information. When consolidation is done, no one has to wonder which spreadsheet is correct – every department sees the same up-to-date numbers. This unified data foundation not only improves current reporting but also ensures any AI or analytics will yield trustworthy results because they’re based on consistent data.
2. Ensure Data Quality and Governance from the Start
2.1 Clean and Enrich Data for Reliable Insights
Unifying data also means cleaning it. Duplicate or erroneous entries across systems will undermine your analytics and AI efforts. Before diving into complex analysis, take time to deduplicate records (one customer = one record), correct obvious errors, and fill in any critical gaps. It’s the classic “garbage in, garbage out” rule: if you train an AI model on flawed data, you’ll get flawed outcomes. High-quality, well-prepared data gives AI a solid foundation, so the insights it produces are accurate and credible rather than misleading.
2.2 Establish Governance, Privacy, and Security Measures
As you consolidate, put basic data governance and security measures in place. Decide who is responsible for which data sets and who is allowed to access them. Establish policies to keep data up-to-date (for example, all new sales leads must be entered into the central CRM, not kept in personal files). At the same time, secure your data: when all your critical information is in one repository or cloud environment, it must be protected. Use access controls to ensure employees only see what they need, encrypt sensitive data, and maintain backups. This also helps with compliance (think GDPR or other regulations). By governing and protecting your data from the start, you build trust in your data-driven approach – everyone knows the data is accurate, used properly, and safe from leaks.
3. Assess Your Technology Infrastructure
3.1 Audit Current Systems and Capacity for AI Workloads
Now assess whether your technology environment can support AI workloads. Inventory your hardware and software: are your servers and databases up to the task of handling large datasets or heavy computation?
Can your network handle constant data syncing or cloud connections without slowing down? Also, check integration capabilities – will your current systems allow easy data export or connection to new AI services (for example, via APIs)? Identify any bottlenecks now. It’s better to discover early that an old system or limited bandwidth could choke your AI project, so you can upgrade or work around it, rather than hitting a crisis mid-implementation.
3.2 Upgrade to Scalable Cloud or Hybrid Solutions
In many cases, getting AI-ready means embracing cloud or hybrid infrastructure. Cloud platforms like AWS, Azure, or Google Cloud give you on-demand scalability – you can access huge computing power or storage when you need it, and scale back when you don’t. This is ideal for AI projects that might require intense resources sporadically. You might keep some core systems on-premises for control or compliance, but offload data-heavy processing to the cloud.
Ensure any cloud services you adopt integrate smoothly with your on-site systems so data flows freely. Keep an eye on costs with cloud (use budgets or alerts) but appreciate that this flexibility often comes at a lower cost than buying and maintaining high-end servers for occasional use. A modern, scalable tech stack means you won’t be held back by infrastructure when your AI ambitions grow.
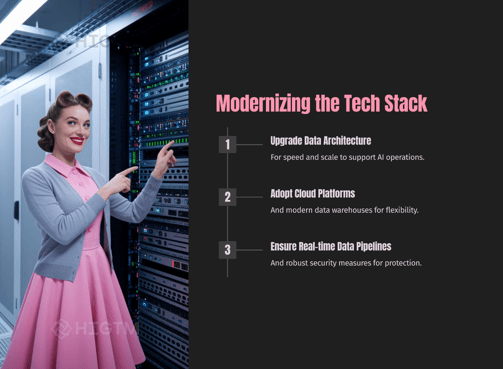
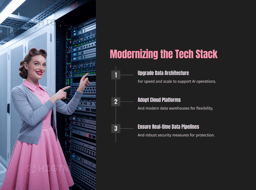
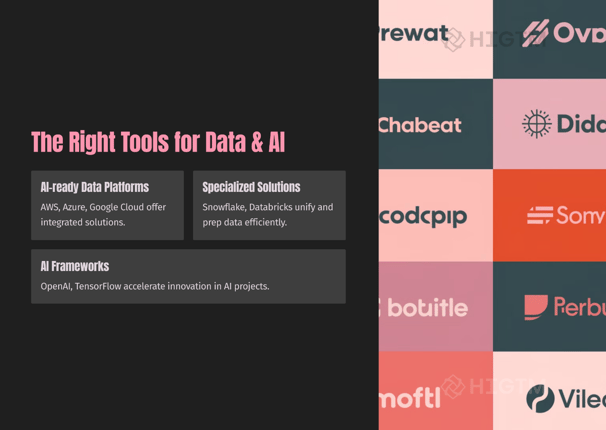
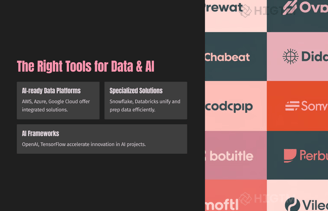
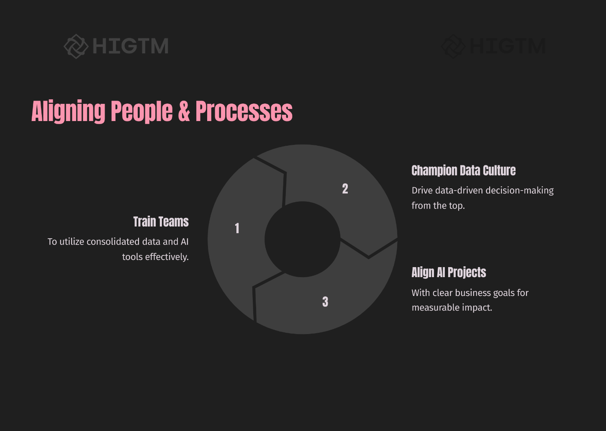
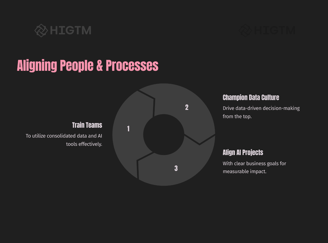
4. Use Leading AI and Data Platform Vendors
4.1 Use Modern Data Management Solutions
Don’t try to reinvent the wheel when it comes to data management – take advantage of the robust solutions major vendors provide. For example, moving your consolidated data into a cloud data warehouse like Snowflake or Amazon Redshift gives you high-performance storage and querying out of the box.
To connect all your different software (CRM, ERP, marketing tools, etc.), you can use integration platforms like MuleSoft or even no-code connectors like Zapier instead of building custom pipelines for each app. If you already use big enterprise systems (say, from SAP or Oracle), leverage their tools to unify data within their ecosystems. Using these proven platforms accelerates your project and provides reliability and security that would be expensive to develop on your own.
4.2 Adopt AI Services and Frameworks Aligned with Your Needs
Similarly, make use of existing AI services and frameworks so you can focus on business solutions rather than low-level algorithms. Cloud providers offer AI building blocks – think Microsoft Azure Cognitive Services, Google Cloud AI APIs, AWS AI tools, or IBM Watson – which let you do things like recognize images, process language, or forecast trends with simple API calls. For many SME needs, these pre-built solutions get you far with minimal development. If you have a specialized use case and skilled developers, you might consider open-source libraries like TensorFlow or PyTorch to create custom models – but remember that requires more effort.
The key is to match the tool to the problem: don’t over-engineer.
For example, use a ready-made chatbot service to handle customer FAQs before deciding to develop an AI from scratch. Start with one or two AI services and see results; you can always expand later. By tapping into these resources, you can deploy advanced capabilities (like an AI recommendation engine or a predictive model) in a fraction of the time it would take to build the AI logic entirely yourself.
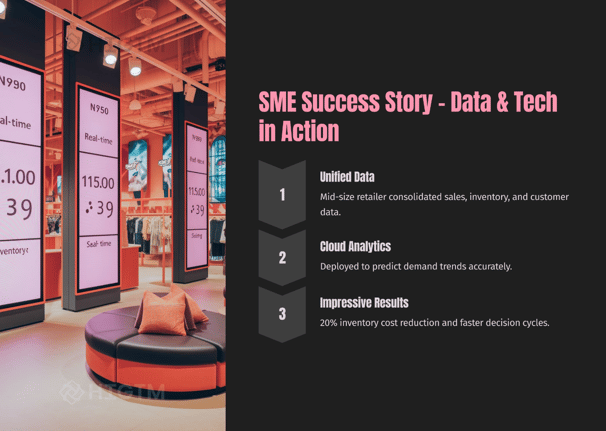
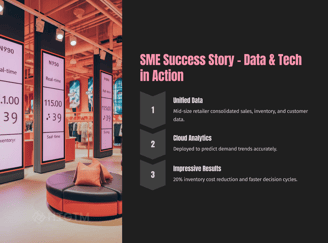
5. Upskill Your Team and Embrace a Data-Driven Culture
5.1 Train Employees on Data Tools and AI Concepts
New technology shines only when people know how to use it. Make sure your team receives training on the data platforms and AI tools you implement. This could be formal (online courses, vendor training sessions, certifications) or informal (workshops, peer training, bringing in an expert for a day).
For instance, if you rolled out a new business intelligence dashboard, train your staff on how to pull reports and interpret them. If you introduced a machine learning-based forecasting tool, explain the basics of how it works and what its outputs mean.
When employees feel confident with the tools, they’re more likely to embrace them in their daily work. Moreover, by investing in your team’s skills, you signal that these technologies are there to augment their abilities – not to replace them. That goes a long way in getting buy-in and enthusiasm for your AI projects.
5.2 Lead a Culture of Evidence-Based Decision Making
Beyond training, cultivating a data-driven culture is a leadership task. Encourage decisions at all levels to be backed by data. In meetings, make it normal to ask “What do the data show?” for any proposal. When results come in, discuss them openly: for example, if an AI-driven marketing campaign performed well, share the numbers and celebrate the team’s analytical approach. If something fails, use the data to understand why and adjust course – this shows that data is a tool for learning, not blame.
Also, be transparent about AI project goals and invite feedback from the people who will use these systems day to day. Maybe set up a cross-functional task force for each new AI project, so IT and business stakeholders collaborate and everyone has a stake. And consistently communicate that AI and analytics are there to help your employees shine by taking over tedious tasks and providing insights – not to replace their jobs.
When the workforce sees leadership relying on facts and supporting them through the change, a culture of evidence-based decision-making will take root naturally.
6. Start with Focused, High-Value AI Use Cases
6.1 Prioritize Projects with Clear ROI and Feasibility
With a solid foundation and team buy-in, it’s time to apply AI to real business problems. Start small and strategic. Pick a use case that addresses a real need and has a clear payoff, but isn’t overly complex. For example, maybe your sales forecasting is often off – an AI model to predict demand more accurately could reduce excess inventory and stockouts.
Or your customer service team is swamped – an AI chatbot might handle common questions and free up humans for more complex issues. List a few candidate projects and rank them by impact and feasibility.
The sweet spot is a project where you have enough data to tackle it, it aligns with your business goals, and a successful outcome would noticeably benefit the company (through cost savings, revenue growth, or efficiency gains). Getting an early win with such a project will build confidence and support for further AI investments.
6.2 Implement Pilot Projects and Iterate
Run your chosen project as a pilot first. Define what success looks like (e.g., “improve forecast accuracy by 20%” or “resolve 30% of support tickets via chatbot in the pilot group”) and a timeframe for evaluation. Deploy the solution on a small scale – maybe for one product line, one regional team, or a subset of customers – and monitor it closely. If the pilot meets or exceeds expectations, you’ll have evidence and momentum to roll it out more broadly. If it falls short, treat it as a learning experience.
Analyze why: perhaps the data feeding the AI wasn’t as clean as anticipated, or users needed more training to work with the new system. Make adjustments and try again, or choose a different approach, applying what you learned. This “start small, learn fast” approach lets you refine the AI solution before committing full-scale. By the time you scale up, you’ll have ironed out the kinks and demonstrated the value, making broader implementation much smoother.
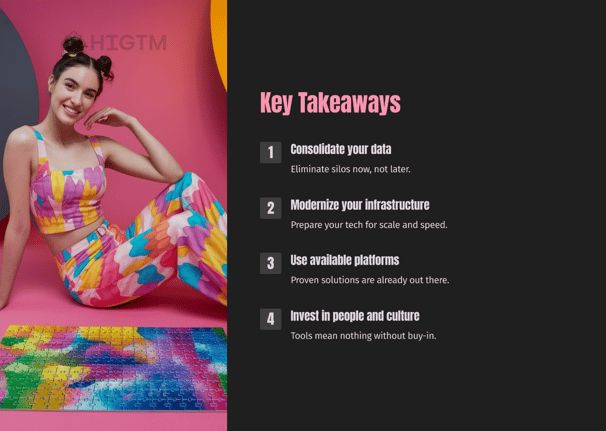
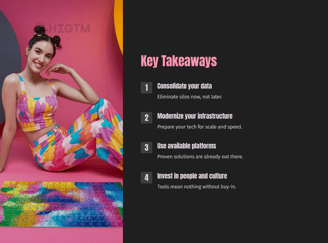
7. Monitor Results and Scale Up Strategically
7.1 Measure Performance and Business Impact
Deploying an AI solution isn’t the end – you need to continuously track how it’s doing. Establish metrics that tell you if the AI is delivering value. Some will be technical (like model accuracy or system uptime), but the most important will be business outcomes. For instance, if you launched an AI forecasting tool, measure its effect on inventory levels, stock-out incidents, or profit margins. If you introduced a recommendation engine on your website, track changes in average order value or conversion rates.
Compare these metrics to the baseline from before the AI was introduced. Regular monitoring not only proves the ROI of your AI initiatives but also alerts you to any issues (maybe the model’s accuracy drifts over time, indicating it needs retraining). Treat AI projects as ongoing operations that get oversight and maintenance, not one-off installs. This vigilance ensures your AI continues to perform as expected and allows you to confidently plan further expansions.
7.2 Refine Your Strategy and Engage Experts if Needed
Finally, use the insights from each project to refine your overall AI strategy. Perhaps an inventory AI was so successful that scaling it company-wide is now a top priority. Or maybe a less successful pilot taught you to focus on a different area with more data. Stay flexible and update your roadmap based on what you learn. Also, recognize when to seek help. As you scale and tackle more sophisticated projects, you might encounter challenges beyond your team’s expertise – and that’s okay.
Consider partnering with external experts or consultants to fill those gaps. For example, you could bring in a data science specialist to help optimize a complex model, or work with a firm like HIGTM that specializes in guiding SMEs through AI adoption.
Getting outside perspective can save time and prevent mistakes, especially for high-stakes or enterprise-wide rollouts. By staying agile and bringing in the right expertise when needed, your SME will steadily build up its AI capabilities – what starts as a tentative experiment can become a core strength of your business.
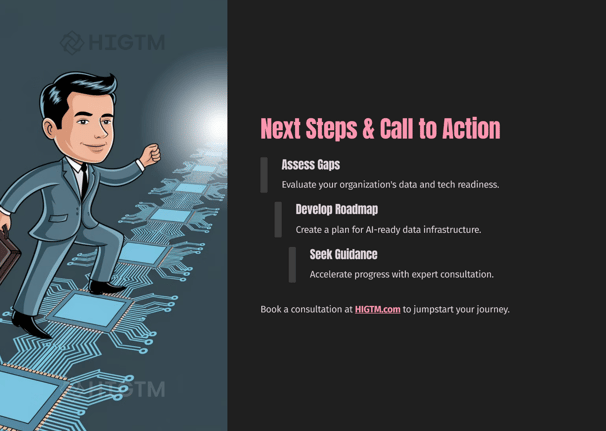
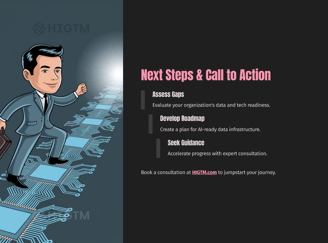
Turn AI into ROI — Win Faster with HIGTM.
Consult with us to discuss how to manage and grow your business operations with AI.
© 2025 HIGTM. All rights reserved.