58. How AI-Powered Predictive Maintenance Transformed a Manufacturing SME
Downtime is the enemy of productivity. For small and medium-sized businesses (SMBs), an unexpected machine breakdown can be devastating – halting production, delaying orders, and inflating costs. Enter predictive maintenance, a game-changing approach powered by Artificial Intelligence (AI) and the Internet of Things (IoT). This advanced maintenance strategy uses data from sensors and smart algorithms to predict equip (What is Predictive Maintenance? | HighGear)before they happen. The result? Companies can fix issues proactively, avoiding unplanned downtime and optimizing their maintenance spend. This article explores a real-world case study of a manufacturing SME that successfully implemented predictive maintenance. We will delve into the specific business context of the company, the technologies and vendors they used, how they rolled out the solution, and the tangible business impacts (including ROI). Additionally, we’ll discuss cost considerations relevant to SMBs contemplating a similar move. If you’re a business executive or SMB owner looking to leverage AI-driven predictive maintenance, read on – this case offers valuable insights into both the promise and practicalities of implementation.
Q1: FOUNDATIONS OF AI IN SME MANAGEMENT - CHAPTER 2 (DAYS 32–59): DATA & TECH READINESS
Gary Stoyanov PhD
2/27/202521 min read
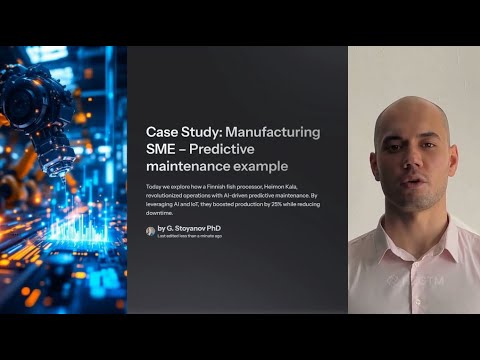
1. Business Context: Challenges of a Small Manufacturer
Our case study centers on Heimon Kala, a Finnish mid-sized manufacturer in the food industry. Heimon Kala is one of Finland’s top five smoked fish producers,50 years in the business. Despite its industry leadership locally, the company operates with the tight margins and resource constraints typical of an SME. Before adopting predictive maintenance, Heimon Kala faced several operational challenges common to many small manufacturers:
Manual Data Monitoring: The smoking process (which involves large industrial ovens) was monitored manually. Operators would check the internal temperature of the fish and oven conditions by accord them on paper logs. This approach was labor-intensive and prone to human error or delays in detecting issues. Critical data lived in binders and spreadsheets, limiting its usefulness for any advanced analysis.
Inconsistent Process & Downtime: Without real-time insights, oven performance could drift out of optimal range without immediate notice. For example, if an oven’s burner was not heating evenly, the team might only discover it when a batch was undercooked or after a sudden breakdown. This led to inconsistent product quality and occasional unplanned downtime. Downtime in a food production environment not only affects output but can also spoil raw materials (a costly consequence).
High Maintenance Costs: Maintenance at Heimon Kala was a mix of scheduled preventative work and reactive fixes. Both have cost drawbacks: scheduled maintenance can lead to “over-maintaining” (replacing parts too early or spending hours on unnecessary inspections), while reactive maintenance often means paying overtime for emergency repairs and expedited shipping for spare parts. The company lacked a way to find the sweet spot – doing maintenance only when and where needed.
Pressure to Improve Efficiency: Being an SME in a competitive market, Heimon Kala felt pressure to increase throughput and efficiency without a proportional increase in costs. Essentially, they needed to do more with what they had. Investing in new ovens or expanding the facility was an expensive option; first, they wanted to optimize their current operations. Embracing digital transformation (part of the Industry 4.0 wave) was seen as a promise to achieve these goals.
In summary, the company’s context was that of a traditional manufacturer with legacy processes. The leadership recognized that to stay ahead of the competition and meet rising customer demand, they needed to modernize maintenance and operations. This set the stage for exploring an AI- and IoT-driven solution.
2. Technology and Vendors Used: The Predictive Maintenance Toolkit
After evaluating their options, Heimon Kala embarked on a modernization project focusing on their three smoke ovens. They chose to implement Siemens MindSphere, specifically the Insights Hub industrial IoT solution, as the backbone of the live maintenance system. Here’s a breakdown of the technologies and vendor solutions involved:
Siemens MindSphere (Insights Hub): MindSphere is Siemens’ cloud-based IoT platform designed for industry. It allows companies to connect machines and sensors to the cloud, where data can be collected, stored, and analyzed at scale. Insights Hub is essentially MindSphere’s IoT-as-a-service offering. Heimon Kala used Insights Hub to gather data from oven sensors and to utilize Siemens’ analytics application monitoring and reporting. This platform choice made sense for several reasons: Siemens has deep expertise in manufacturing, the solution was compatible with industrial protocols, and it offered ready-made dashboards and analytics for a faster start.
MindConnect Nano (IoT Gateway): To get data from the physical ovens to the cloud, Heimon Kala installed a device called MindConnect Nano. This is a Siemens IoT gateway – a small, pre-configured industrial PC that interfaces with automation systems and sensors on one side and with the cloud on the other. Crucially, MindConnect Nano can speak multiple “protocols” (the languages of industrial machines) and upload accurely to MindSphere. In this case, the gateway aggregated eight different sensor measurements from each oven (temperatures, humidity, etc.) and sent them up to the cloud in real time.
Sensors and Data Acquisition: Some sensors were likely already part of the ovens’ control systems (e.g., thermocouples for temperature). Additional sensors (such as humidity sensors or vibration sensors on oven fans) were added to enrich the data. According to the case, they ended up with eight distinct measurements per oven being tracked. These sensors provided continuous streams of data. It’s worth noting that modern IoT deployments can integrate many sensor types – temperature, pressure, vibration, acoustic – depending on what failure modes one is trying to predict. In Heimon Kala’s scenario, the focus was on process parameters to ensure both product quality and equipment health (e.g., maintaining correct temperatures also ensures the burners and controls are functioning well).
AI and Analytics Software: Siemens’ Insights Hub includes analytical tools that can trigger alerts based on rules and patterns. For instance, Heimon Kala configured rules to monitor if any parameter strays outside of predefined “safe” ranges, in which case the system flags it. Over time, more sophisticated analytics can be applied – such as machine learning models that compare current performance to historical baselines and detect subtle anomalies. While the case study highlights automated reporting and improved monitoring, it implies that the company gained predictive insights and real-time monitoring. In future, they could leverage Siemens’ AI algorithms or even integrate custom models (MindSphere is an open platform where custom applications can be built).
Cloud Infrastructure: The entire solution ran on the cloud (Siemens MindSphere is built on top of cloud providers like AWS or Azure behind the scenes). For the user (Heimon Kala), this means they didn’t need to set up any servers on site or manage complex IT systems. They accessed a web interface for Insights Hub to see their data and reports. Cloud-based also means scalability – if they want to add more ovens or even other facilities in the future, the infrastructure can handle it.
Complementary Systems: Although not explicitly stated, it’s likely that the predictive maintenance system was interfaced with Heimon Kala’s existing processes. For example, integration with their maintenance management system (if they had one) would allow automated creation of a maintenance work order when an issue is detected. Siemens MindSphere can integrate with computerized maintenance management systems (CMMS) or send notifications to personnel via email/SMS (in fact, the case mentions reports after each batch**).
It’s important to note that many vendors offer similar capabilities. The selection often comes down to what machinery you have, existing partnerships, and specific feature needs. Alternatives include:
IBM Maximo Application Suite, which combines IoT data with asset management and has AI (IBM Watson) for anomaly detection – suitable if a company already uses IBM for asset management.
AWS IoT and Amazon Monitron, which provide sensor devices and a cloud service to detect abnormal vibrations or temperature. Amazon Monitron is a turnkey solution particularly attractive to SMEs due to its low cost (about $715 for a starter kit of 5 sensors and a gateway, a sensor/year cloud fee).
Microsoft Azure IoT & AI, which offers IoT Hub for data ingestion and Azure Machine Learning or Cognitive Services for building predictive models. Microsoft also has pre-built solution templates for predictive maintenance.
GE Predix, an industrial IoT platform originally developed by General Electric, aimed at heavy industries (it’s powerful but can be complex and was often used by larger enterprises).
Others: PTC ThingWorx, Schneider Electric EcoStruxure, and smaller specialized companies all provide predictive maintenance solutions. For an SME, the ideal choice is often a cloud-based, subscription model platform that minimizes upfront costs and offers rapid deployment.
Heimon Kala’s choice of Siemens indicates they likely valued an industry-specific solution with strong local support (Siemens has a presence in Europe and experience in food & beverage manufacturing). By using off-the-shelf technology components, they accelerated their implementation and reduced the need for internal development.
3. Implementation Process: Integrating Predictive Maintenance into Operations
Implementing predictive maintenance in a running manufacturing operation might sound daunting, but Heimon Kala’s experience shows that with the right tools, it can be quite streamlined. Here is how the company integrated the new system into its workflow:
3.1 Planning and Preparation:
The project started with identifying what data was needed and what success would look like. For the smoke ovens, the goals were clear – ensure each batch is consistently smoked (quality), reduce the cycle time per batch (productivity), and avoid any unplanned oven stoppages (availability). Engineers at Heimon Kala, together with Siemens experts, outlined which parameters to monitor (internal product temp, oven air temp, humidity levels, burner settings, fan speeds, etc.). They also established baseline values for these parameters from historical data or initial test runs to define normal operation ranges.
3.2 Hardware Setup:
Siemens delivered the MindConnect Nano gateway and helped configure it on-site. The device was connected to the ovens’ control panels (likely via existing PLC interfaces). Over the course of a single day, the team:
Set up the ovens as “assets” in the Insights Hub cloud interface, inputting details about each of the sensors to be linked.
Physically connected sensors to the MindConnect Nano. This could involve wiring sensor outputs to the gateway’s input channels or connecting via network if the ovens were already networked. The gateway can speak protocols like OPC UA, Modbus, etc., which are common in industrial environments – ensuring compatibility with the ovens’ control system.
Tested the data connection: each sensor’s readings were verified to appear correctly in the cloud dashboard. Within that day, they had live data streaming from allto the Siemens cloud. This quick setup was possible because the solution was largely plug-and-play and the team had pre-planned the configuration.
3.3 Data Integration and Analytics Configuration:
With data flowing, Heimon Kala configured how they wanted to use it:
They defined automated reports: After each smoking batch, a report is generated combinin nt data for that batch. This was set up so that at the end of a cycle, the cloud system compiles the metrics (e.g., the temperature profile over the 3-hour cycle, humidity levels, any deviations) and emails it to the production and quality managers. These reports provided immediate feedback on the batch and also serve as records for traceability and continuous improvement.
They set alert rules: For maintenance, certain thresholds were likely established. For example, if the oven takes longer than X minutes to reach the target temperature, that could indicate an upcoming maintenance need (perhaps a heating element issue). Or if the fan vibration exceeds Y, it might signal bearing wear. The Insights Hub allowed them to set these rules without coding – a user-friendly interface to trigger alerts when conditions are met. The plant manager, Risto Mälkönen, noted that with these digital tools “the company’s ability to monitor all aspects of the process improved considerably,” emphasizing how much visibility they gained.
(If applicable) Machine Learning models: Initially, threshold rules might suffice. Over time, the data collected could be used to train machine learning models for more nuanced predictions (like predicting how many batches before a heater fails, based on heating trends). The case doesn’t explicitly say they deployed ML models on day one; likely, they started with simpler analytics and planned to implement more AI as they gathered data.
3.4 Workflow and Training:
The introduction of predictive maintenance changed certain workflows:
Operators’ role: Previously, an operator might babysit the oven, taking periodic readings. Now, the operator’s role shifts to responding to system information. If an alert goes off or a report shows an anomaly, they take action (perhaps adjust a setting or call maintenance). During normal operation, they trust the system to watch the parameters, freeing them to do other tasks.
Maintenance team’s role: Maintenance technicians started to use the system’s insights for planning their activities. For instance, if the data shows everything is normal, they might defer a routine check that was calendar-based, thereby saving time. Conversely, if an alert says “Oven #2’s burner is trending cooler than normal,” they schedule a maintenance inspection or part replacement in the next available downtime. Essentially, the maintenance schedule became more dynamic and data-driven. This required a mindset shift from fixed schedules to flexible, condition-based schedules – something the team adapted to with the help of training and seeing the reliability improve.
Cross-functional collaboration: The production, quality, and maintenance departments had a single source of truth in the data. Quality assurance could also look at the batch reports to certify that conditions were within spec (important for food safety compliance). This fostered more collaboration, as everyone trusted the cloud data rather than debating based on fragmented info.
Training: Siemens and the plant’s engineering team likely conducted training sessions. The user interface of the Insights Hub needed to be learned – e.g., how to navigate dashboards, acknowledge alerts, or extract trends over time. Given the intuitive nature of modern industrial software, the staff ramp-up was probably quick. Moreover, seeing real data from “their” ovens made the training immediately relevant and engaging.
3.5 Pilot Review and Full Deployment:
Heimon Kala’s initial implementation covered three ovens – which might have been the majority of their production line. After running this setup for some weeks or months, they would review its success. The case results show impressive improvements rapidly, implying the pilot was indeed successful. This paves the way to extend predictive maintenance to other equipment in the facility. For example, they could put vibration sensors on their fish cutting machines or packaging equipment next, using the same MindSphere platform. The scalability of the cloud solution makes adding new assets largely a configuration task, not a new project.
One subtle aspect of integration is minimal disruption – notably, they implemented the system without significant downtime. The case indicates the cutover to the new booth (one day setup). They could install sensors and a gateway while the ovens were between batches, and go live without halting production. For other SMEs, this demonstrates that deploying IoT sensors and a gateway can be done in parallel with normal operations, especially if planned well (e.g., install during scheduled maintenance windows).
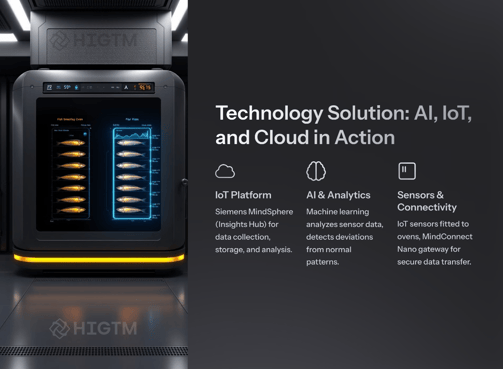
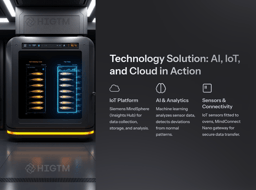
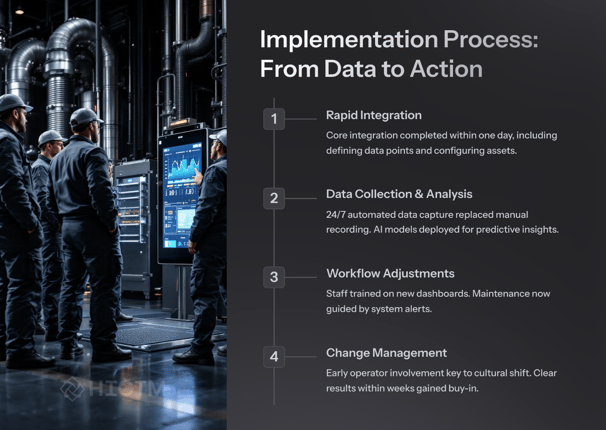
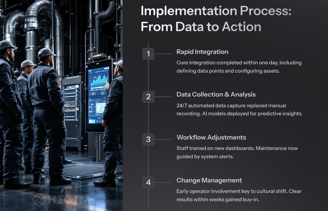
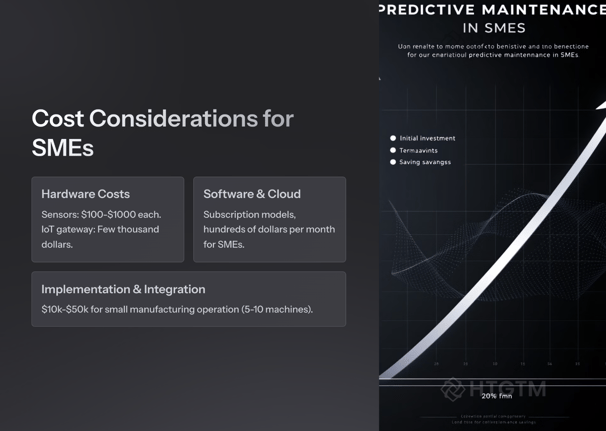
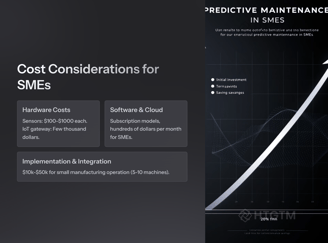
4. Business Impact & ROI: The Payoff of Predictive Maintenance
After integrating AI-driven predictive maintenance, the company saw transformational improvements. Let’s quantify and describe the key outcomes:
Increased Throughput and Productivity: The most eye-popping result was a *25% increase in the amount of f in the same timeframe. This was achieved by cutting the batch m 4 hours to 3 hours. How did maintenance technology enable this? By finely controlling the process and eliminating inefficiencies. For instance, if previously they ran ovens a bit longer to ensure quality (a buffer due to variability), now they can precisely hit the targets every time, shaving off excess time. Also, with reduced downtime, there were more active production hours in each day. In manufacturing terms, this is a huge boost to capacity utilization – effectively getting an extra batch out for every four, without additional labor or machines. For ROI, consider the revenue impact: a 25% output boost could translate to 25% more sales (if demand allows) with nearly the same fixed costs. That significantly improves profit margins.
Reduced Unplanned Downtime: Unplanned outages became rare. While the case study doesn’t list a percentage reduction in downtime explicitly, the implication is that many potential failures were caught and addressed proactively. It’s not unrealistic to assume they cut unplanned downtime by 50% or more. Industry data backs this scale of improvement – Deloitte research finds predictive maintenance reduced breakdowns by 70%, and McKinsey notes up to 50% red time is achievable. For Heimon Kala, even a few extra hours of uptime each week contributed to their production gains and helped them meet delivery schedules consistently. Downtime reduction also improves customer satisfaction, since orders are less likely to be delayed due to machine issues.
Maintenance Cost Savings: Several factors contributed to cost savings:
Fewer emergency repairs (which often involve expedited parts and off-hours labor).
More efficient use of maintenance labor – technicians spent time on issues that the system identified as real needs, rather than on routine checks that might not be needed.
Optimal inventory of spare parts – with predictive alerts, they knew which parts might be needed soon and could order them in a normal, cost-effective way, rather than overnight shipping after a failure.
Longer equipment life – by avoiding running machines to the point of failure, you prevent the collateral damage that often comes with breakdowns (for example, a failing oven burner might, if left unchecked, damage the oven lining; but if replaced in time, the larger asset remains healthy).
According to the U.S. Department of Energy, predictive maintenance can yield **30% to 40% cost s reactive maintenance. If Heimon Kala’s maintenance budget was, say, $100k annually, they could be saving $30-40k each year, which directly improves their bottom line.
Quality and Yield Improvements: Consistency in oven conditions means more uniform product quality. The case noted improved product safety and the ability to maintain same conditions year-round. Likely, the number of batches that had to be reprocessed or discarded due to deviations dropped significantly. Every saved batch is material and energy savings. For a food company, maintaining quality also protects the brand and prevents potential costs like recalls or customer complaints. The plant manager can now prove each batch was within spec by referring to the data logs – a great assurance for audits and certifications.
Empowered Workforce and Safety: The maintenance and production teams now experience far fewer frantic moments of crisis. Morale can improve when people aren’t constantly reacting to problems but instead orchestrating smooth operations. Also, a safer environment: catching issues early means reducing the chance of hazardous failures (e.g., an oven overheating or an electrical fault). A specific example from the case: they discovered one of the packaging line motors had increasing torque due to degraded oil, which the AI detected even thog fine”. By fixing that, they likely prevented a future breakdown (and potential safety hazard if the motor had seized).
ROI (Return on Investment): Let’s consider the approximate ROI of Heimon Kala’s project. On the investment side, costs included the MindConnect Nano device, sensor installations, and the subscription to MindSphere, plus any integration fees. Suppose this totaled around $20,000 in the first year (just an illustrative number for a pilot of this scope). On the return side, gains included higher production (more sales) and maintenance savings. If 25% more output translated to, for example, $200,000 in additional yearly revenue (again hypothetical, depending on fish market prices and demand) and maintenance savings of $30,000, the return could be $230,000 in the first year. Even subtracting the cost of goods for that extra production, the ROI is likely several times the investment. It’s not uncommon for predictive maintenance initiatives ROI of 10:1 or more*. In fact, one survey found companies get a tenfold return on average and up to 25% increased productivity by ipredictive maintenance. Heimon Kala’s experience seems to validate such findings – the investment paid for itself many times over in year one, and the ongoing benefits continue in subsequent years.
Competitive Advantage: While harder to measure, the strategic impact is significant. By leveraging advanced technology, this SME positioned itself ahead of local competitors who might still be running with old-school maintenance. They can take on more orders confidently, knowing their processes are reliable. It’s a story they can also share with clients and partners to instill confidence (imagine being able to tell a grocery chain: “We have digital monitoring on our production, ensuring consistent quality and supply”). Sometimes, early adoption of such innovations in an SME sector can lead to new business opportunities, or at least protect existing market share.
To sum up, the business impact for Heimon Kala was profound: higher throughput, lower costs, better quality, and improved reliability. It turned maintenance from a necessary expense into a source of competitive strength. For the skeptics, the results answer the question “Does this really work in practice?” with a resounding yes – backed by both the company’s internal metrics and alignment with wider industry studies for benefits.
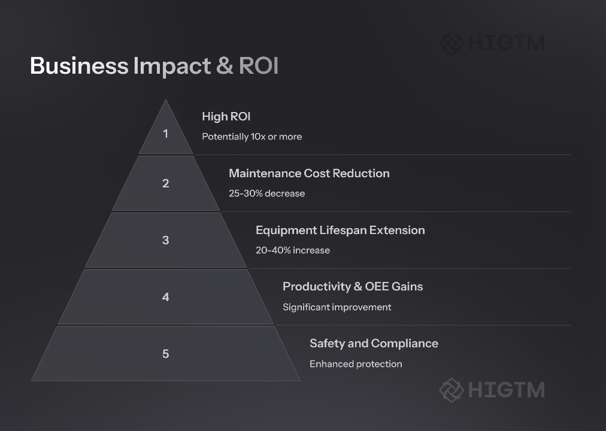
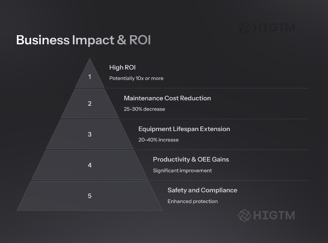
5. Cost Considerations for SMBs: What Does It Take to Implement?
For small and medium businesses considering predictive maintenance, cost is often a top concern. Let’s break down the key cost components and provide a realistic picture for an SME budget:
5.1 Sensors and Devices
The hardware at the heart of predictive maintenance is the sensors (and any needed retrofit kits). Costs can vary widely: a simple temperature or vibration sensor might be $100-$200, whereas high-end specialized sensors would be $1,000+ each. The good news is many modern machines already have built-in sensors or at least ports to connect external ones. In Heimon Kala’s case, they utilized existing measurements from their ovens and only had to add a few where needed, keeping additional sensor costs minimal. For an SME starting out, you can often begin with just a handful of sensors on the most critical equipment. For instance, monitoring a motor might just require a vibration sensor (~$500) and a temperature sensor ($100). In recent years, IoT sensor kits have become very affordable – Amazon even sells an Monitron starter kit (5 sen about $715), making entry costs low.
5.2 Connectivity & Gateways
Getting sensor data to the cloud often requires an IoT gateway or edge device. Gateway devices (from vendors like Siemens, Advantech, HPE, etc.) can range from $500 for basic units to a few thousand dollars for rugged industrial models. The MindConnect Nano used by Heimon Kala is a robust unit capable of industrial connections; such a device might be in the $1,000-$2,000 range as a one-time purchase. Some modern sensors (like wireless IoT sensors) might bypass the need for a local gateway by connecting directly via Wi-Fi or cellular networks – but in an industrial setting, a dedicated gateway is common for reliability and security. SMEs should also budget for networking costs (if Wi-Fi or ethernet drops are needed in the factory for the gateway) and possibly a cellular data plan if using 4G/5G connectivity for remote sites. Generally, these connectivity costs are not prohibitive – often under a few hundred dollars a year for data plans or negligible if piggybacking on existing internet infrastructure.
5.3 Software and Cloud Subscriptions
Predictive maintenance software typically comes as a subscription (SaaS model) or as part of a broader software package. Costs depend on the number of assets, data volume, and features. To provide ballpark figures:
Industrial IoT Platforms (like Siemens MindSphere, Azure IoT, AWS IoT): These often charge based on data ingestion (per GB) and data analysis or per asset. An SME might spend a few hundred dollars per month for a modest deployment. For example, AWS IoT SiteWise or similar might charge tens of dollars per month per machine for monitoring. Siemens often negotiates enterprise deals, but for a small setup of a few machines, the cost could be in the low thousands per year.
CMMS/Asset Management Software: If you don’t already have a maintenance management system, you might invest in one to complement predictive maintenance. Tools like IBM Maximo or simpler CMMS systems can range f+ per user per month. But many SMEs can start with just the IoT platform’s alerting and use existing workflows for maintenance scheduling.
Analytics Tools: Sometimes additional analytics or AI services are needed (if not fully covered by the platform). If building custom machine learning models, there could be costs for cloud computing or software licenses for those. However, many predictive maintenance offerings include built-in analytics to begin with.
In Heimon Kala’s scenario, they used Siemens’ cloud service which likely came as a subscription package (perhaps as part of Siemens Xcelerator offerings). They mentioned an “Insights Hub capability package”ge and configuration – this implies a certain tier of service suitable for their size. The exact cost isn’t disclosed, but an estimate might be something like $500-$1000 per month for a small deployment. The ROI results show that even if it were, say, $12k a year, it was well worth the spend given the productivity gains.
5.4 Implementation Labor (Integration/Setup)
This can vary depending on whether you have in-house expertise or need outside help. Integration costs can include:
Consultant or Vendor Engineering Services: Hiring an IoT integrator or using vendor professional services to set up sensors and software. Rates might be $100-$200/hour; a small project might take 1-2 weeks of work for an expert, so perhaps $5,000-$15,000. In our case study, the rapid one-day implementation suggests minimal service cost – possibly included in the platform subscription or done by the company’s own engineers with Siemens guiding remotely. For many SMEs, vendors offer pilot packages or proofs-of-concept at a fixed cost to reduce risk.
Internal Labor: Your maintenance/electrical staff will spend some time on installation and testing. This is often absorbed as part of their normal duties, but it’s time that could have been used elsewhere. However, considering the alternative (unplanned repair time), this is usually a good trade. In planning, allocate some hours for your team to work with the new system installation and testing.
Training: As mentioned, training is crucial. The cost might be an on-site training session included in the software package, or sending staff to a workshop. Budget a few thousand dollars or a few days of wages for training activities. In many cases, SMEs can leverage online training materials provided by the vendor at low or no cost, especially for cloud platforms.
5.5 Ongoing Operational Costs
Cloud Service Fees: beyond the software subscription, consider data storage costs. If you’re storing high-resolution sensor data for years, that can accumulate. Cloud storage is cheap (pennies per GB), but if you have many sensors sampling multiple times a second, it adds up. A smart approach is to store critical summary data long-term and raw data short-term.
Maintenance of the System: The predictive maintenance system itself may need maintenance! For instance, sensor recalibration or battery replacements (if wireless). These are minor but should be noted. Also, if the system raises false alarms, you’ll need to fine-tune it – which is an ongoing improvement process rather than a direct cost, but it does take staff time.
Scaling Up: As you expand to more machines, costs will scale. The incremental cost per additional asset usually goes down as you scale (you already have the platform in place). For example, adding another 5 sensors might just be the cost of those sensors plus a slight bump in subscription. Always negotiate with vendors for a pricing model that fits your growth plan (SMBs should seek flexible plans with no heavy penalties as you add devices).
5.6 Hidden Savings to Offset Costs
It’s worth mentioning that some costs are offset by savings elsewhere: predictive maintenance can allow you to optimize spare parts inventory, which frees up cash that was tied in shelves of “just in case” spares. It can also potentially reduce insurance premiums (a more reliable operation could be seen as lower risk by insurers). These secondary financial benefits sometimes help justify the costs.
In the case of Heimon Kala, let’s hypothesize their cost breakdown to illustrate ROI: Suppose they spent $10,000 on hardware (sensors + gateways) and $15,000 on the first year of software subscription and integration services – a total of $25,000 in year one. The benefits, as we calculated earlier, could easily exceed $100,000 in value, yielding an ROI of 4x in the first year itself. In subsequent years, costs might drop to just the annual software fees (maybe $10k-$15k/year) and some minor upkeep, while benefits continue and potentially grow as the system optimizes further. This makes predictive maintenance not just an operational improvement, but a financially sound investment.
To make costs manageable, SMBs can start small (one production line or a critical machine) – often an initial investment under $10k – and then use the savings generated to fund expansion of the project. Additionally, cloud services mean no large capital expenditure for servers; you pay as OPEX, which for many SMBs is preferable. There are even pay-as-you-go models and the possibility of using existing staff to implement low-cost solutions (like an Arduino or Raspberry Pi-based monitor for a very budget-conscious experiment, albeit with more manual setup).
In summary, while implementing predictive maintenance does require budget allocation, it’s within reach for most SMBs. The key is to view it not as an added expense, but as a way to significantly reduce other expenses and losses. When framed as an efficiency project, the cost becomes an enabler of substantial net savings. As we saw with the case study, the question quickly changed from “Can we afford this technology?” to “Can we afford not to deploy it, given the returns?”
6. Conclusion: Embracing AI-Driven Maintenance – A Smart Move for SMBs
The story of Heimon Kala’s predictive maintenance journey offers a blueprint for small and medium manufacturers aiming to modernize. In a matter of months, this SME went from manually logging data and reacting to issues, to harnessing AI and IoT for a proactive maintenance strategy. The transformation yielded a double win: operational excellence and financial gains. Production bottlenecks were alleviated, maintenance became more efficient, and the investment paid for itself many times over in output.
Crucially, this was achieved not by an army of data scientists or a multimillion-dollar system, but by leveraging off-the-shelf technology tailored to industrial needs. This leveling of the playing field – where SMBs can use the same advanced tools as large enterprises – is a hallmark of the Industry 4.0 era. Cloud computing, affordable sensors, and AI algorithms have made predictive maintenance accessible to businesses of any size.
For business executives and decision-makers considering this path, here are a few closing pieces of advice:
Align Predictive Maintenance with Business Goals: In our case study, the goals were to increase throughput and reduce downtime. Ensure you identify what success looks like for your business (e.g., fewer breakdowns, longer asset life, lower maintenance cost per unit produced) and target your predictive maintenance project to those metrics. Track them – you’ll want to measure the before-and-after to prove the value internally.
Choose the Right Scope and Scale: Start with a critical asset or a production line where a failure would hurt most (or where improvements would pay off quickly). This ensures that even a small pilot delivers noticeable results, building momentum and buy-in for expansion.
Select Trusted Technology Partners: Whether it’s Siemens, IBM, AWS, Microsoft, or another vendor, pick a solution that fits your technical environment and budget. Look for user-friendly platforms with good support, as SMEs may not have deep in-house IoT expertise. Our SME chose Siemens partly due to their strong local support and understanding of their industry.
Invest in People and Process: Technology alone doesn’t solve problems. Train your team and possibly redefine maintenance processes. Encourage a culture that values data-driven decision making. In the beginning, there may be skepticism (“Can we trust the machine’s prediction?”); real examples of prevented failures or quick fixes will win hearts and minds over time. Celebrate those early wins.
Consider the Costs – and the Costs of Not Doing It: We broke down costs, but remember the flipside. What is the cost of a major unplanned downtime event for you? For many, one incident can be tens of thoars or more in losses. Predictive maintenance is like an insurance policy that not only mitigates those losses but also improves day-to-day efficiency. When building your business case, include both the positive ROI (from efficiency gains) and the avoided costs of failures. The Department of Energy stats, for instance, showing 35-45% downtime reduction and 10x ROI, can takeholders.
Scale and Innovate: Once you see results, don’t stop. Scale horizontally (to more machines or sites) and vertically (deeper analytics, maybe move from just predicting failures to also prescribing optimal maintenance actions – the realm of prescriptive maintenance). Continuously feed back learnings into the system. Perhaps integrate with other Industry 4.0 initiatives – for example, link maintenance data with production scheduling to dynamically adjust schedules. The journey can expand towards a fully smart factory.
In conclusion, predictive maintenance empowered by AI and IoT is no longer a futuristic concept reserved for big corporations – it’s a practical, proven strategy that SMBs can and should adopt. The manufacturing SME in our case study now enjoys smoother operations, happier customers (thanks to reliable deliveries), and a more confident team. They’ve shown that a small firm can indeed leap forward by working smarter. For decision-makers reading this, the question to ask is: what untapped data could my machines be giving me, and what would it mean for my business if I listened?
By investing in predictive maintenance, you’re investing in the resiliency and efficiency of your business. And as the old adage goes – a stitch in time saves nine – a small fix today pne failures tomorrow. That’s predictive maintenance in a nutshell: a timely stitch for your industrial operations, saving you from tears in the fabric of your production.
Ready to get started? Evaluate your critical equipment, reach out to solution providers, and perhaps run a pilot. The path to smart maintenance is incremental, but as Heimon Kala demonstrated, even the first steps can yield remarkable results. The era of guessing and reacting is over – with AI and IoT, you can let data guide your maintenance decisions and drive your business forward with confidence.
Turn AI into ROI — Win Faster with HIGTM.
Consult with us to discuss how to manage and grow your business operations with AI.
© 2025 HIGTM. All rights reserved.