57. 10 Success Criteria for AI Adoption in Retail SMEs (in 3-6 Months)
Implementing artificial intelligence in a retail small/medium enterprise can feel daunting, but it doesn’t have to take years or millions in budget. With focus and the right approach, an SME (around 50+ employees) can start reaping benefits of AI in as little as 3-6 months. The key is to define clear success criteria from the outset. Below, we outline 10 critical success criteria – think of these as benchmarks and guiding principles – to ensure your AI adoption journey is on the right track. Follow these, and you’ll be on your way to quick, tangible wins with AI.
Q1: FOUNDATIONS OF AI IN SME MANAGEMENT - CHAPTER 2 (DAYS 32–59): DATA & TECH READINESS
Gary Stoyanov PhD
2/26/202527 min read
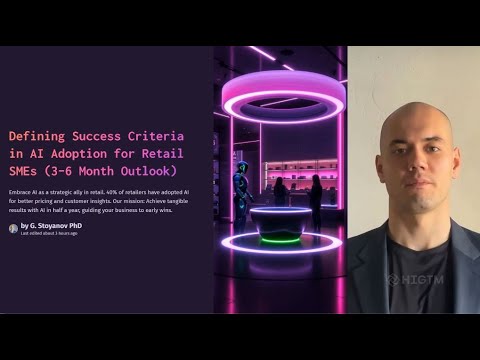
1. Clear Business Objective and KPI Definition
Success Criterion: Every AI initiative must have a well-defined business goal and a measurable KPI.
The first criterion for success is knowing exactly what you want to achieve. Define a clear objective for your AI project that ties directly to a business outcome. This could be increasing online sales conversion rate by 5%, reducing stockouts by 30%, or improving customer satisfaction scores by 1 point in 6 months. Alongside the objective, identify the Key Performance Indicators (KPIs) that will measure progress toward that goal. For example: conversion rate, stockout frequency, or Net Promoter Score (NPS).
Why it matters: Without a clear target, you won’t know if your AI adoption is succeeding. Vague goals like “we want to use AI to improve our business” lead to meandering projects. In fact, research warns that many AI projects fail at thhe pilot stage due to lack of clear goals and success criteria. To avoid being part of that statistic, treat goal-setting as step zero of your journey.
Subpoints / How to implement:
Align with Business Pain-Points: Ensure the objective addresses a real pain-point or opportunity in your retail business (e.g., long checkout lines, unpredictable demand, customer churn). If the AI project solves a pressing problem, its value will be evident quickly.
Make KPIs Quantitative: “Improve inventory management” is not as good as “cut excess inventory by 15% in value.” Numbers focus the mind. Establish a baseline for your KPI (where you are today) and set a realistic yet ambitious target for 3-6 months out.
Write a Success Statement: Formulate a one-sentence success statement, such as “Success = AI-powered recommendation system increases average basket size from $30 to $33 by end of Q2.” This clarity will guide every decision in the project and keep the team aligned.
Avoid Vanity Metrics: Pick KPIs that reflect genuine business impact. For example, model accuracy or number of AI features deployed are less meaningful if they don’t translate to cost savings or revenue. Focus on outcomes, not outputs.
By having a crystal-clear objective and KPI from day one, you set a strong foundation. At the 3-month mark, you should already see movement in your chosen metric; at 6 months, you’ll know if you’ve hit the target or how close you came.
2. Executive Sponsorship and Team Alignment
Success Criterion: Leadership is visibly committed to the AI initiative, and a cross-functional team is in place and aligned on the project’s importance.
AI adoption is as much about people as technology. A critical success factor is getting executive buy-in and assembling the right team. This means a senior sponsor (e.g., CEO or COO of the retail SME) who actively supports the project, allocates budget, and clears obstacles. It also means all relevant departments (IT, sales, operations, marketing, store managers, etc.) are aware of and aligned with the AI project’s goals.
Why it matters: In an SME, resources are limited and people wear multiple hats. If leadership isn’t vocally behind a project, day-to-day firefighting will always take priority over the new AI experiment. Conversely, when the owner or GM says “this is a top priority for our growth,” teams will make time for it. Strong leadership signals also help overcome resistance – employees see that AI is part of the company’s future, not just a fad.
Subpoints / How to implement:
Appoint an Executive Champion: This person will champion the AI effort at the highest level, keep it aligned with business strategy, and ensure it gets the necessary resources. They’ll also be the one to communicate its importance across the organization, for example by mentioning it in company meetings or memos (“Improving our inventory with AI is a key initiative this quarter”).
Build a Cross-Functional Task Force: Include members from IT/data, the business unit that will use the AI (e.g., e-commerce manager for a recommendation engine project), and perhaps customer-facing staff if it impacts store operations or customer service. This diverse team ensures all perspectives are covered – technical feasibility, business need, and user experience. It also fosters buy-in because each department feels represented.
Set Regular Check-ins: Have bi-weekly or monthly project meetings where the sponsor and team review progress. Early on, these check-ins maintain momentum and accountability. If an executive is in the loop and asking “how are we progressing, do you need help?”, the team stays motivated and roadblocks get removed faster.
Unified Vision and Messaging: Make sure everyone on the team can articulate the project’s goal (from Criterion #1) in the same way. If you ask five team members what the AI project is aiming to do, you should hear a consistent answer. This unity will show in coordinated efforts and clear external communication (e.g., to the rest of the company or even to customers if needed).
Success at 3-6 months will partly be measured by team engagement: Is the executive sponsor still actively involved? Do team members report that collaboration is strong? High team morale and support indicate that the AI adoption is a shared mission, not an isolated experiment. When leadership and team are in sync, the project has the wind at its back.
3. Focused Pilot with High Impact (Start Small, Win Big)
Success Criterion: Launch a small-scale pilot targeting a high-impact use case, and achieve a measurable “quick win” by the 3-6 month mark.
Rather than attempting a massive, do-everything-at-once AI transformation, successful SMEs start with a focused pilot project. The idea is to choose one use case that is feasible to implement quickly and can deliver noticeable benefits. By limiting scope, you ensure speed; by picking a high-impact area, you ensure the result is meaningful. For a retail SME, this might be something like: “Implement an AI-driven product recommendation section on our website” or “Use AI to automate the ordering of top 50 products each week.”
Why it matters: A focused pilot avoids analysis paralysis and endless planning. It turns AI from theory into practice within weeks. Importantly, it provides a proof point – a successful pilot builds confidence, teaches lessons, and generates momentum for broader adoption. According to experts, starting with high-impact complexity AI projects is key to seeing positive returns quickly. You want an early victory in that 3-6 month window to justify further investment.
Subpoints / How to implement:
Identify Candidate Use Cases: Look for processes that are painful or inefficient, or opportunities you’re not capitalizing on because of manual limitations. Common retail examples: demand forecasting, personalized marketing, pricing optimization, customer service automation. Brainstorm a list, then score them on two axes: impact on business and ease of implementation. Target the sweet spot: high impact, relatively easier to implement (doesn’t require unreal data or huge tech overhaul).
Leverage Existing Solutions: To move fast, plan to use existing AI services or off-the-shelf solutions for your pilot (more on that in Criterion #5). If, for example, your pilot is a chatbot for customer service, you can use a ready platform like Dialogflow or Zendesk’s Answer Bot rather than developing NLP models from scratch. This dramatically cuts down implementation time.
Time-Box the Pilot: Set a tight timeline: e.g., “We will develop for 8 weeks, then go live and test for 4 weeks.” By month 3 or so, something is live and being used in the real world. Time-boxing forces the team to trim nice-to-have features and focus on core functionality that delivers the value.
Define Pilot Scope Clearly: Be very clear on what’s in and out. If you’re piloting AI for inventory on one product category, don’t suddenly add other categories mid-pilot. If the pilot is online recommendations for a subset of users, stick to that plan. Controlling scope is vital to avoid delays. Remember, expansion comes after proving the concept.
Measure the Win: Before you start, decide what outcome will constitute a successful pilot. For example, “In the 4-week test, the AI recommendations should increase click-through rate by at least 15% compared to control.” Or “The AI ordering system should reduce excess stock of the pilot items by 20% this quarter.” These become your mini success criteria within the pilot. When you hit them, publicize it internally – “we aimed for 15% lift and got 18%!” That’s your quick win.
By month 6, you ideally want to have completed a pilot and collected data on its performance. If it achieved the targeted improvement (or even came close), you have a concrete success story. Even if it fell short, you’ll have learned invaluable information to refine the approach. A completed pilot with clear outcomes is a success criterion in itself – it means you’ve moved from concept to reality rapidly, which is a huge psychological and practical win.
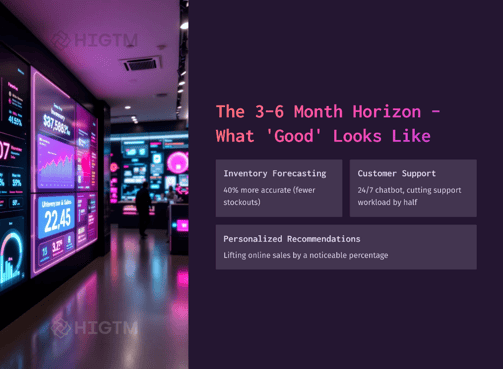
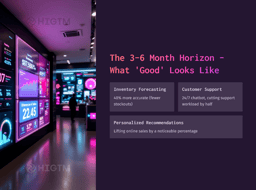
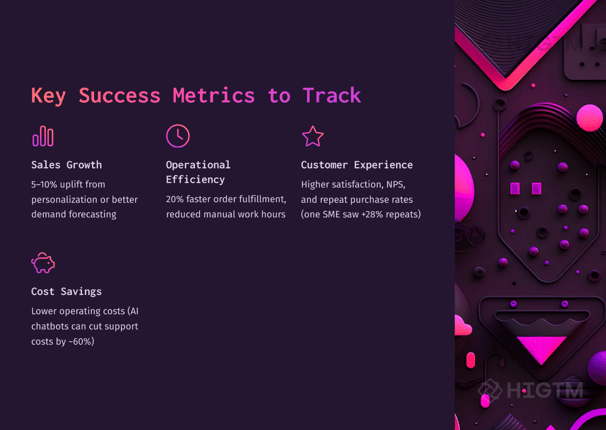
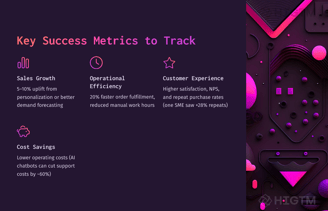
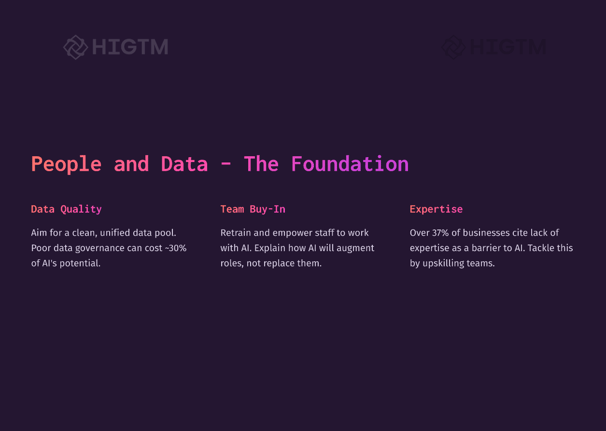
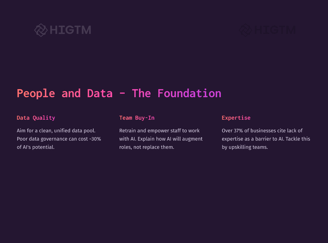
4. Data Readiness and Quality Management
Success Criterion: Necessary data for the AI project is identified, gathered, and cleaned to a sufficient level at the start, with ongoing improvements in data quality throughout the project.
Data is the fuel of AI. Even the best AI model won’t deliver results if it’s fed poor or irrelevant data. For success, an SME must ensure that early in the project, it has secured the data sources needed and addressed glaring data quality issues. Being “data-ready” doesn’t mean perfect data (no one has that), but it means data is not a showstopper. If your AI is a car, you need enough gasoline in the tank to drive – you can refine the fuel as you go, but you must avoid running on empty or dirty fuel that clogs the engine.
Why it matters: Retail SMEs often have data scattered across POS systems, Excel sheets, maybe a small ERP, and so on. If you dive into implementation without consolidating data, you’ll hit delays or, worse, the AI will give faulty outputs. For example, training a sales forecast AI on wrong sales data will obviously yield nonsense. On the other hand, taking time (a few weeks at project start) for a data audit and cleanup can set you up for smooth sailing. As one guide suggests, you don't need perfect data to begin, but could have a plan for continuous improvement of data quality. That sums it up well.
Subpoints / How to implement:
Identify Data Needs: Based on your use case, list what data is required. If doing product recommendations: you need product info, transaction history, maybe web analytics data. If doing demand forecasting: sales history, seasonality data, inventory levels, etc. Map out where this data currently resides.
Data Audit: Quickly assess the condition of that data. Are there a lot of missing entries, duplicates, or errors? Is data from different sources (e.g., online sales vs in-store sales) siloed and in incompatible formats? An audit doesn’t have to be exhaustive, but do enough to know the major issues.
Fix Critical Issues First: Tackle the “blockers” – e.g., if product IDs don’t match between the sales system and the web store database, create a key to join them. If 20% of customer ages are missing and your use case needs age demographics, maybe fill those with an average or flag them as unknown rather than dropping them (a data scientist can help with strategies). Standardize obvious inconsistencies (like “CA” vs “California” in addresses, or different date formats). These are one-time fixes that yield a cleaner dataset for AI to munch on.
Implement Data Pipeline & Governance (if needed): Set up how data will flow into the AI system. Do you need a simple export from your POS weekly, or a real-time integration via API? Implement that pipeline early so when your AI model is ready, the data is flowing. Simultaneously, put basic data governance in place: assign someone to oversee data updates, ensure new data is captured correctly, and document what data is used and how. This could be as simple as a spreadsheet of data sources and owners.
Plan for Continuous Improvement: Accept that some data issues will persist, but have a plan to improve them over time. For instance, if addresses are messy in customer data, you might not fix it all at project start, but you decide to implement a new form validation in your e-commerce checkout to improve new data going forward. Or schedule a monthly cleanup of outliers and errors as they are discovered by the AI. The key is not to let data quality stagnate. Over 3-6 months, your data should actually become better thanks to these efforts, which in turn improves AI performance.
Measuring success at 3-6 months: One indicator is that the AI system has had consistent access to the data it needs (no major gaps or downtime due to data issues). Another indicator: data quality metrics have improved (e.g., completeness went from 85% to 95%, duplicates reduced, etc., if you track those). If your AI is producing credible results that team members trust, that’s a sign your data foundation is solid. Remember, data quality is directly linked to AI output quality, so treating this criterion seriously will show in the success of the whole project.
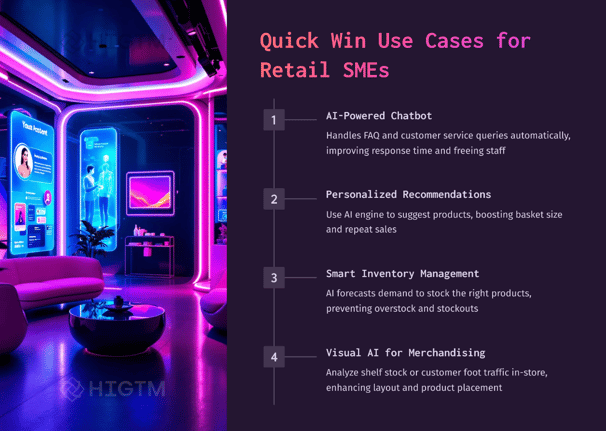
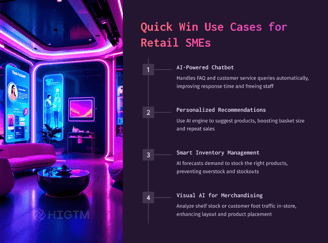
5. Use of Proven AI Tools and Expertise
Success Criterion: Leverage existing AI platforms, frameworks, or expert partnerships to accelerate implementation, rather than reinventing the wheel.
A mid-sized retailer doesn’t need to create a cutting-edge AI algorithm from scratch to succeed – in fact, doing so could jeopardize your 6-month timeline. Instead, tap into the rich ecosystem of proven AI tools and services. Success means smart “buy or borrow” decisions: using cloud AI services, open-source libraries, or hiring external experts/consultants to fill skill gaps. This criterion is about being resourceful and pragmatic.
Why it matters: There are many AI solution providers (big names and startups) that have already developed what you need – be it a recommendation engine, a computer vision tool for recognizing products, or a pre-trained model for natural language. By using them, you save development time and benefit from their reliability. For example, major vendors like AWS, Google Cloud, Microsoft Azure, IBM, and Salesforce have AI offerings tailored to retail needs. These services are battle-tested and scalable. As an SME, exploiting these means you can stand on the shoulders of giants and get results faster. Similarly, if your team lacks data science experience, bringing in a consultant or partner (even short-term) can prevent costly trial-and-error. The success criterion here is that by 6 months, you didn’t waste time solving solved problems; you focused on configuring solutions to your business.
Subpoints / How to implement:
Survey Available Solutions: Once you have your use case, research what solutions exist. E.g., for demand forecasting: Amazon Forecast or Google’s AI platform might have ready solutions. For image recognition (maybe you want AI to analyze in-store camera feeds), look at Azure Cognitive Services or Google Vision API. Check if there are specialized retail AI startups with a plug-in product. Often, a quick search or consulting a tech advisor can map the landscape of tools for you.
Build vs Buy Analysis: For each component of your AI project, decide whether to build in-house or use external tools. Key factors: time to implement, cost, and strategic differentiation. If the component isn’t core to your unique value, lean towards using an external solution. For example, if you want a chatbot, using IBM Watson Assistant or Google Dialogflow is typically faster and cheaper than building a custom NLP model. Save your custom development for things that give you a unique edge and can’t be bought.
Use Open Source and Cloud Frameworks: Even if you do some custom work, you don’t start from zero. Libraries like TensorFlow, PyTorch, or scikit-learn (for machine learning) are free and well-documented. Likewise, cloud platforms offer pre-built models and also infrastructure to train/deploy your own models. Using these can cut development time massively. Many cloud AI services can be deployed in days, not months.
Engage Experts: Recognize your team’s limits. If no one on your staff has built an AI model or integrated one, it can help to bring in an expert – whether it’s a freelancer, a consultant from firms like HIGTM (😉), or even tapping into vendor professional services. They can guide initial setup, share best practices, and train your team on maintenance. The cost of an expert’s guidance often pays for itself by preventing mistakes and accelerating progress. Even a few weeks of an expert’s time during the project’s early phase can keep you on the right track.
Plan Knowledge Transfer: If you use external help or tools, ensure your team learns to use them. Part of success is that by the end of the pilot, your internal team can operate the AI solution (or at least understand it enough to work with it). So, have the consultant document what they did, or have your developers shadow them. If using a cloud service, perhaps get someone on your team certified in that service (cloud providers offer lots of training). This way, you’re not permanently dependent on outside help and you’ve grown your team’s capabilities through the project.
Meeting this success criterion means that by the 3-6 month point, the technology choice is validated: you have a working solution largely thanks to leveraging existing tech and know-how. You didn’t stall out trying to build a spaceship from scratch in your garage – you assembled one using high-quality parts available on the market and expert assembly instructions. This pragmatic approach is often what separates AI projects that deliver on time from those that drown in complexity.
6. Employee Training and Change Management
Success Criterion: End-users and stakeholders are trained and comfortable with the new AI-driven processes, and the organization has embraced the change (no significant resistance).
For AI to truly be adopted, the people in your company must adopt it. This means training employees, adjusting workflows, and managing the change so that the AI tool is actually used and trusted. A project could hit all technical marks but still fail if, say, your sales staff refuse to follow the AI’s pricing recommendations, or your inventory managers “ignore the system” because they don’t understand it. Thus, an essential criterion is that by the end of the initial phase (3-6 months), your team is on board: they know how to use the AI solution, and they want to use it because they see its value.
Why it matters: SMEs often have employees who have done things the same way for years. Introducing AI can cause anxiety (“Is this replacing my job?”) or skepticism (“This computer doesn’t know our customers like I do”). Without proper change management, the AI system might be technically live but practically unused or undermined by manual overrides. On the flip side, with good training and communication, your staff can become AI’s biggest advocates. They’ll provide feedback to improve it and ensure its outputs translate into real business actions. Remember, AI is a tool for humans, not a magic box. Success is when humans + AI together are performing better than either would alone.
Subpoints / How to implement:
Early Communication: Even before the AI tool goes live, talk to the people who will be affected. Explain what the project is, why the company is doing it, and how it will benefit both the business and hopefully make their work easier. Address the elephant in the room about job security – emphasize that the AI is there to assist, not replace (assuming that’s the case). Highlight that by taking over mundane tasks, it frees them for more valuable work. Employees are more receptive when they understand the intent and see leadership acknowledging their concerns.
Tailored Training Programs: Once the system is nearly ready, conduct training sessions. These might be workshops, hands-on demos, or one-on-one coaching, depending on the complexity and who needs to use it. Training should be role-specific. For example, if it’s a chatbot AI, customer service reps need to know how/when it hands off chats to them, and managers need training on reading the chatbot analytics. If it’s a forecasting tool, the inventory planners need to learn the interface and how to interpret the forecasts. Provide cheat-sheets or quick reference guides post-training so they can refresh their memory.
User-Friendly Design: Work with your implementation team to ensure the AI system’s user interface (if there is one) is as intuitive as possible. Sometimes a lot of training issues can be mitigated by good UX. If your employees find the tool easy to use and aligned to their usual workflows (e.g., accessible through systems they already use), adoption will be smoother.
Change Champions: Identify a few tech-savvy or enthusiastic employees and make them “change champions”. They can be the go-to persons for their peers when using the new AI tool. They often help informally on the floor, easing the transition. Also, they can gather feedback and issues to report back to the project team. This creates a bridge between the implementation team and the end-users.
Feedback and Iteration: Encourage feedback once people start using the AI. Perhaps schedule a review meeting a month into use: “How is it going? What problems are you facing? Any suggestions?” Take this feedback seriously and, if possible, address quick wins (like maybe the AI tool needs a tweak or more training data because it gave some odd suggestions in week 1). When employees see their feedback leading to improvements, they feel a sense of ownership. It turns them from passive users into active contributors, which is great for long-term adoption.
Signs of success by 3-6 months: Your staff is using the AI tool regularly as part of their job, without constant prompting. They understand its outputs and trust them (at least to a reasonable degree). You might even have quotes or anecdotes, like a salesperson saying, “The new recommendation engine suggested a combo I wouldn’t have thought of – and it’s selling well!” or a support rep saying, “The AI chatbot takes care of the easy questions, so I only deal with the complex issues now – it’s a relief.” If the majority of targeted end-users have a positive or at least neutral attitude (versus negative) about the AI by the six-month mark, consider this criterion met.
7. Performance Tracking and Early ROI Measurement
Success Criterion: A system is in place to track the AI’s performance, and initial ROI or improvements against baseline metrics are demonstrable within 3-6 months.
When your AI solution goes live, the work isn’t over – now it’s about measuring outcomes. A successful adoption means you set up mechanisms to continuously track the KPIs defined in Criterion #1 and any other relevant performance indicators. By the 3-6 month point, you should be able to see and report on how the AI is moving those numbers. Even if full ROI (return on investment) isn’t achieved yet, there should be clear trends or partial returns that indicate you’re on the right path. Essentially, you want to show, with data, that “this AI thing is working.”
Why it matters: In business, if it’s not measured, it’s not managed. Stakeholders – whether that’s your CEO, your investors, or just your own peace of mind – will want to know if the AI project is paying off. Having tracking in place allows you to answer that with evidence. It also helps catch issues: maybe the AI isn’t performing as expected, and you need to know that ASAP to make adjustments. Moreover, early success data is what you’ll use to justify scaling the project or investing in more AI initiatives. Many companies calculate things like time saved, cost reduced, revenue added to see how quickly the project “pays for itself.” Most businesses see initial results within 3-6 months for quick-win AI projects, so it’s realistic to expect measurable progress in this timeframe.
Subpoints / How to implement:
Establish Metrics Dashboards: Set up a simple dashboard or report that the project team and stakeholders can view regularly. For example, if you introduced an AI recommendation engine, have a dashboard showing sales from recommendations, click-through rates, average order value, etc., compared to pre-AI baseline. If it’s an inventory AI, track stock levels, stock turnover rates, write-offs, etc., vs. before. Many cloud tools have analytics built-in; if not, use whatever business intelligence tool you have (even Excel or Google Sheets can suffice initially) to aggregate and display the data.
Baseline and Benchmark: Ensure you recorded the “before AI” baseline of your metrics. E.g., last quarter’s sales, last year’s average monthly stockout count, customer service average response time, etc. This baseline is your comparison point. Also, if available, use industry benchmarks. For instance, if AI chatbots typically deflect 30% of queries, how is yours doing? Such context helps evaluate performance.
Monitor Key Metrics Weekly/Monthly: In the initial months, watch the numbers frequently. Is the metric trending in the right direction? For instance, by month 2 post-deployment, you might see shopping cart size creeping up if recommendations are working, or customer wait times dropping if an AI scheduling tool was implemented. Some metrics might have natural volatility (seasonality in sales, etc.), so account for that in your analysis.
Calculate ROI Components: ROI = (Gains from AI – Cost of AI) / Cost of AI. By 6 months, you may not have full annualized figures, but you can estimate: “We invested $50k in this project so far, and it’s generated an estimated $40k in incremental revenue and $20k worth of cost savings = net $10k gain, which is a 20% ROI in half a year.” Or perhaps “We’re still slightly net negative but trending to break even by month 9.” Don’t ignore the cost side – include software fees, consulting, man-hours spent, etc., to honestly assess when you’ll any AI projects aim for a positive ROI within the first year, with some quick-win cases hitting it in 6 months.
Identify Intangible or Qualitative Benefits: Not everything shows up immediately in the financials. Maybe the AI improved customer satisfaction or employee morale. If you have any data or anecdotes, note them. For example, “Store managers report spending 4 hours less per week on manual ordering (time reallocated to merchandising).” Or “Customer emails praising the faster response have increased.” These qualitative wins often precede quantitative ones and are worth highlighting.
By the 6-month mark, you should prepare a brief report for your leadership (and team) on the performance. If you can say, for instance: “Our AI-powered marketing campaign optimization led to a 12% increase in email click-through rates that led $30,000 in additional sales this quarter,” that’s a clear, data-backed success. Or if the results are mixed, you can say, “We’re halfway to our go to out-of-stock incidents by 40% – currently at 20% reduction – and here’s our plan to reach the full target.” The key is, you know where you stand and can quantify the impact to date. That’s success: the AI isn’t a black box; it’s a measurable contributor to your business.
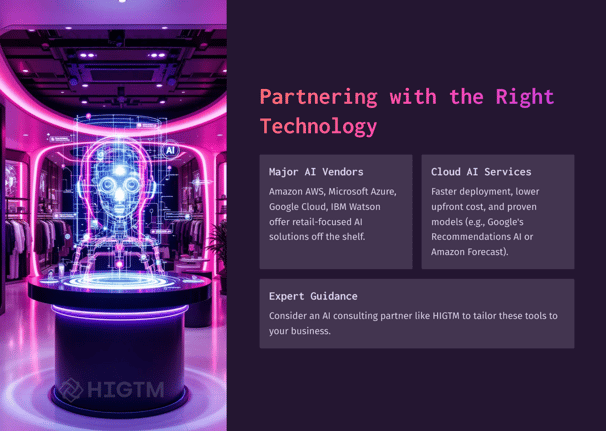
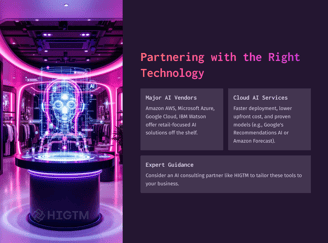
8. Visible Improvement in Key Retail Metrics
Success Criterion: By 3-6 months, the AI initiative has led to noticeable improvements in at least one core retail metric (sales, margin, customer satisfaction, efficiency), directly tied to the success criteria defined.
This might seem like a restatement of the above, but it’s emphasizing the outcome itself. Beyond tracking, the criterion is that something important got better, and it’s attributable in part to the AI. For a retail SME, core metrics include things like total sales, gross margin, average transaction value, customer footfall, same-store sales growth, online traffic, conversion rates, customer retention, inventory turnover, shrinkage, etc. Not all will move in a few months, but one or two should if the AI is doing its job. Essentially, success is when you can say: “Because of our AI project, [metric X] has improved by Y% compared to before.”
Why it matters: This is the crux – if after 6 months nothing has improved, it’s hard to label the project a success. The whole reason for adopting AI is to drive better results. The improvement doesn’t have to be huge (especially if your baseline was already good), but it should be statistically or operationally significant. For example, even a 3% increase in sales can be significant if normally your sales were flat, or a 20% reduction in return rate due to better recommendations can save a lot of money. Moreover, a visible improvement is a morale boost and a green light to continue investing in AI. It proves the value to any skeptics as well.
Subpoints / examples of improvements:
Sales Increase: Perhaps your AI-driven upselling tool online contributed to monthly e-commerce sales going up from $100k to $108k (an 8% lift) after its introduction, while other traffic factors remained steady. That’s a clear improvement. Salesforce research found 87% of SMBs it helps them scale operations and 86% saw improved margins, showing that top-line and bottom-line gains are a common outcome when AI is applied smartly.
Cost Savings: Maybe your AI inventory optimizer reduced overstock and you can quantify $10k lower inventory holding costs and spoilage in 3 months. Or an AI scheduling system reduced overtime hours needed by making shifts more efficient, cutting labor cost by 5%. These savings often drop straight to the bottom line.
Customer Experience: This could be measured via NPS, CSAT surveys, online reviews, or repeat purchase rates. For instance, your NPS might have risen from 30 to 40 after implementing an AI chatbot that improved service responsiveness an average +12 point NPS improvement with AI in some cases). Or you notice a bump in repeat customers since launching personalized promotions with AI.
Efficiency/Productivity: Perhaps previously it took your team 5 days to analyze sales data and reorder products each cycle, and now an AI does the analysis overnight, so reorders happen 2 days faster – resulting in less stockout time and fresher stock. Or your marketing team can produce 4 campaigns a month instead of 2 because AI automates segmenting and content suggestions. Time saved can be translated to either cost saved or more output with the same resources (efficiency gain).
Accuracy/Quality: In areas like forecasting or data entry, AI might reduce errors. For example, a manual process had 10% error rate, and the AI-driven process has 2% – leading to fewer customer complaints or returns. Or demand forecasts are now accurate within 5% error, vs 15% before, leading to better stock allocation. Those improvements show up in other metrics (sales, costs) but are worth noting independently.
Tying to criteria: Importantly, link the metric improvement back to the success criteria you set. If your goal was a 5% sales increase in 6 months, did you hit it? If yes, success! If you exceeded it, even better – highlight that. If you fell slightly short, but still improved significantly, that’s still a positive outcome, though it might indicate room for iteration. For example: “Our target was a 10% lift in online conversion; we achieved 7%. This is a solid improvement, and we have identified tweaks in product recommendations to push this closer to 10% in the next quarter.”
By making an explicit connection between the AI project and the metric improvement, you strengthen the case that AI caused or at least facilitated the success. Sometimes multiple factors affect a metric, so be fair in assessment. If there was a major marketing push or an external trend, acknowledge it while showing that areas influenced by the AI (like products where the recommendation engine was active) outperformed others. In any case, by month 6 you want a headline result to communicate: e.g., “AI pilot yields 15% reduction in excess inventory” or “AI boosts email click rates by 2x, contributing to revenue growth.” That’s the kind of tangible result that spells success.
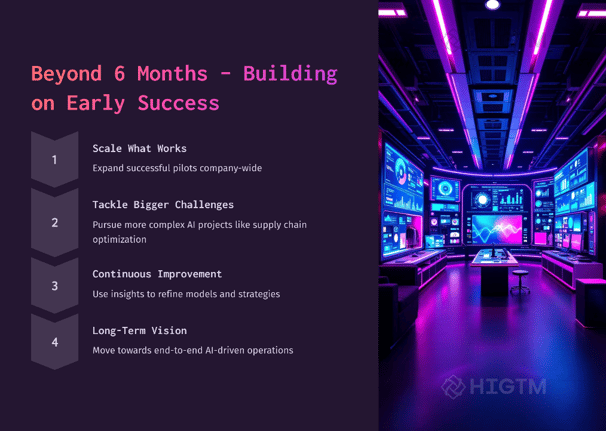
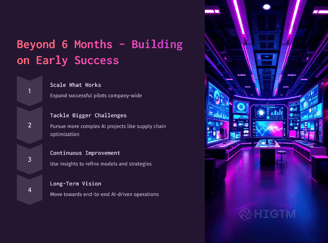
9. Stakeholder Buy-In for Expansion
Success Criterion: Key stakeholders (management, board, etc.) are convinced of the AI project’s value and are ready to support its continuation or expansion beyond the initial 3-6 month pilot.
You know an AI adoption has been successful not just by past metrics, but by what everyone wants to do next. If after seeing the 3-6 month results your company’s leadership and stakeholders say, “This is great – how can we do more?”, that is a huge success marker. It means the project earned confidence and people are willing to invest further. This could be in the form of budget approval for phase 2, greenlighting AI for other use cases, or even just public praise and excitement from decision-makers internally. Essentially, the narrative shifts from “should we do AI?” to “how do we do more with AI?”
Why it matters: SMEs often operate with tight budgets and cautious strategies. A pilot might be given limited support until it “proves itself.” If by 6 months you’ve turned skeptics into believers, you’ve cleared one of the hardest hurdles. With genuine buy-in, subsequent AI projects or scaling the current one will face less friction. Also, broader buy-in might mean more departments want to collaborate, seeing the success. For instance, if a pilot was in online sales, now the retail operations team might say “we want AI for in-store promotions too!” That eagerness is a sign of cultural adoption of AI at the organization level.
Subpoints / How to gauge and foster this:
Present Results and Learnings: Don’t assume stakeholders know the good news. Prepare a compelling summary of what the pilot achieved (using the data from criteria 7 and 8). Include hard numbers, lessons learned, and testimonials or feedback from staff/customers. Present this to management in a dedicated session. Basically, tell the success story of the project. If the numbers are good, this will naturally generate positive responses.
Recommendation for Next Steps: In that presentation or report, recommend what to do next. People often need a nudge on how to capitalize on success. For example: “We recommend expanding the AI recommendation engine to our entire product catalog (it was on 30% of products in pilot) which we estimate can double the revenue impact. Additionally, we see an opportunity to use a similar approach in our physical stores via clienteling apps.” Laying out a vision for expansion helps stakeholders see the path forward.
Address Remaining Concerns: Stakeholders might have lingering questions: What about cost for scaling? Are there any risks or things we need to watch (compliance, etc.)? Show that you have thought about these. For instance, “To scale this AI, we’ll need to upgrade our cloud subscription – cost $X – but the projected ROI remains strong at Y%. We’ll also implement a formal data governance policy as we integrate more data sources, to ensure quality and compliance.” This thoroughness builds trust.
Get Testimonials from Stakeholders: If a store manager or a department head was initially unsure but now is enthusiastic because they saw benefits, encourage them to share that with higher-ups. An email or a comment like “This tool has really helped my team, I’d love to have it in more stores” coming from an operational leader can greatly influence executive perception. It’s not just the AI team patting themselves on the back; it’s real end-users advocating.
Visualize the Future: Paint a picture of what broader AI adoption could do. Maybe show a roadmap of next 6-12 months if you continue: multiple use cases, integration, transformation. This gets people excited that the pilot was just the beginning. If your brand has a strategic plan, tie the AI success to strategic goals (e.g., “This supports our vision to be a data-driven retailer” or “this will help us scale without equivalent headcount increases, fueling growth”).
Signs of success: The CEO allocates more budget for AI in next quarter’s plan. The board mentions the AI project in a positive light in meetings. Other department heads approach you or the project team saying, “I heard this went well – can we also get involved or do something similar?” Essentially, momentum is building. If instead you get lukewarm responses or “let’s hold off on doing more,” then perhaps stakeholders aren’t convinced – which means the success wasn’t clear enough or communicated well enough. But assuming you met criteria 1-8, you likely have a great case. When the top brass and broader org say “Yes, let’s double down,” you’ve achieved a pivotal success: organizational buy-in.
10. Plan for Scaling and Sustainability
Success Criterion: A clear plan is in place by month 6 to scale the AI solution (or extend to new use cases), and the necessary resources and infrastructure for long-term sustainability are identified.
The final marker of success in this initial adoption phase is forward-looking. By the end of 6 months, you shouldn’t be scrambling or wondering “now what?” Instead, you should have a roadmap for the future of AI in the organization. This includes scaling the existing solution to full deployment (if it was a pilot) or rolling out to more stores/users, and potentially tackling the next AI project with the insights gained. It also means ensuring the AI solution is maintainable: who will own it going forward, how will it be monitored, how will models be updated or retrained (if applicable), and is the IT infrastructure ready for sustained use? Essentially, success is not a one-off win that fades – it’s setting yourself up to continuously derive value from AI.
Why it matters: Many projects succeed in pilot and then flounder in the gap before full implementation. You want to avoid the prototype graveyard. Having a plan and commitment to scale turns a temporary victory into lasting transformation. It also means the ROI can multiply as you extend AI’s reach. From a strategic view, this criterion ensures that AI becomes part of your business process, not just an experiment. Organizations that treat AI as an ongoing capability – refining and expanding it – get far more value than those that stop at a pilot. So, even as we celebrate short-term success, we plan the next horizon.
Subpoints / components of the plan:
Rollout Strategy: If your pilot was limited (geography, product line, etc.), detail how you’ll roll it out company-wide. For example, “In the next 3 months, deploy the AI recommendation engine to 100% of the website’s product pages” or “Expand the inventory algorithm to all 10 stores after refining it with pilot feedback.” Set a timeline and steps (and who’s responsible for each). If the pilot uncovered some weaknesses, plan to address those before/during scale-up.
Resource Allocation: Identify what resources are needed for scale. Do you need additional budget for software licenses, or perhaps to hire a data analyst to manage the AI system full-time? Maybe the pilot was manageable with a small team, but full deployment needs more hands or different skill sets (like integrating with another system, or managing a larger dataset). Get those requirements on paper and, if possible, approved by management (ties back to Criterion #9 – if they’re bought in, they’ll likely approve resources).
Ownership and Governance: Decide who “owns” the AI going forward. Will it be the IT department, a newly formed data team, or a business unit? Define roles: who monitors the AI’s performance weekly, who handles issues if the AI outputs seem off, who maintains the data pipeline, etc. Establish governance for model updates – e.g., “We will retrain the product recommendation model every 3 months with the latest data” or “The pricing AI’s rules will be reviewed quarterly by the pricing manager and data scientist together.” Having this governance means the AI solution stays accurate and relevant over time (no set-and-forget).
Integration into Business Processes: Ensure the AI process is baked into standard operating procedures. For instance, if previously store managers decided orders manually and now the AI suggests orders, update the SOP: “Each Monday, store managers review AI-generated order proposals, adjust if needed, then approve.” This cements the AI’s role. If you treat the AI outcomes as just advice that can be ignored, over time usage may lapse. So institutionalize how and when it’s used.
Continuous Improvement: The plan should also include how you’ll gather ongoing feedback and improve the system. Perhaps set up a quarterly review of AI performance and user feedback. As your business evolves (new products, new stores, seasonal changes), the AI might need tweaking. Have a mechanism to catch drift or new requirements. Some companies even set up an AI/analytics council to oversee all such projects. That might be overkill for an SME, but at least assign a point person to keep an eye on things.
Next Use Cases: Finally, list potential next AI projects, prioritized by impact/feasibility, based on what you learned. Maybe during the pilot you realized, “We could also use AI to optimize pricing” or “Customers really responded to personalization; what if we do AI-driven email campaigns?” You don’t need to start them immediately, but having a vision for the next 6-12 months of AI helps keep the momentum. It signals that AI adoption is not a one-shot initiative but a journey of ongoing innovation.
By meeting this criterion, you demonstrate that the initial success was not a lucky one-off, but the first step in a broader transformation. In 3-6 months, you’ve not only delivered results, but also prepared the organization to scale those results exponentially. When you have both short-term wins and long-term plans, that’s the hallmark of a truly successful AI adoption in your SME.
11. Conclusion: Success is not the end - but a springboard!
Adopting AI in a retail SME within a short timeframe is entirely achievable – as long as you define what success looks like and take strategic steps to reach it. These 10 success criteria serve as checkpoints on your AI journey. From setting clear goals and securing leadership support, through executing a focused pilot with quality data and the right tools, to training your team and measuring impact – each criterion ensures that AI delivers real value, not just hype.
In 3-6 months, a retail SME can go from zero to AI-powered hero: boosting sales, cutting costs, delighting customers, and empowering employees. The key is to stay focused on outcomes and be agile in execution. Avoid generic forays into AI; instead, tie every effort to a business result. As we’ve seen, companies doing this are already resing AI report higher revenues and efficiency gains. You can count your business among them by following these principles.
Finally, success is not the end – it’s a springboard. Meeting these criteria means you’ve built a foundation of trust and capability in AI. From here, you can confidently scale up and explore new use cases (visual AI for merchandising, advanced customer analytics, and more). The competitive edge you gain will compound over time.
So set those goals, rally your team, start that pilot, and keep your eyes on the prize. In six months, you could be looking at a very different business – one that’s smarter, faster, and ready for the future of retail. The journey to AI success starts with defining it – and now you have the map. Good luck, and may your results speak louder than any buzzwords ever could!
Turn AI into ROI — Win Faster with HIGTM.
Consult with us to discuss how to manage and grow your business operations with AI.
© 2025 HIGTM. All rights reserved.