46. Selecting an AI Partner in Finance: Deep Dive into Criteria and Due Diligence
Banks, investment firms, and fintechs are racing to adopt artificial intelligence – from automating compliance to enhancing customer experiences – but C-suite executives know that not all that glitters is gold. This deep-dive article cuts through the noise and provides a pragmatic guide to selecting an AI vendor, focusing on the hard-nosed realities of AI adoption in finance. We’ll explore six key evaluation criteria, industry context with top players, and strategic insights to ensure your AI partnership delivers real-world impact (and not just hype).
Q1: FOUNDATIONS OF AI IN SME MANAGEMENT - CHAPTER 2 (DAYS 32–59): DATA & TECH READINESS
Gary Stoyanov PhD
2/15/202524 min read
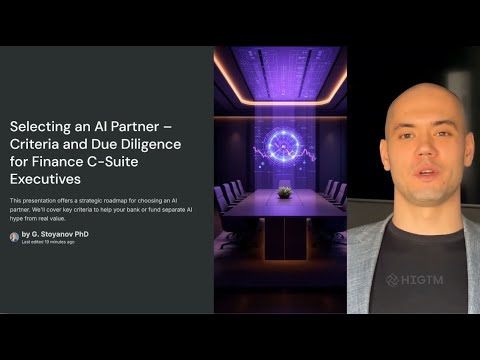
1. Technical Expertise & Capabilities: Finding Substance behind the Sales Pitch
Financial leaders must first assess an AI vendor’s technical prowess and ability to deliver high-performing AI models tailored to financial use cases. It’s easy for a supplier to throw around buzzwords like “machine learning” and “predictive analytics,” but can they actually build and deploy models that meet your institution’s needs?
1.1 Domain-Specific Experience
Look for AI partners with demonstrated experience in the finance domain. This might include use cases such as credit risk scoring, fraud detection in banking, algorithmic trading, portfolio optimization, or customer churn prediction for fintech apps. A vendor steeped in financial industry projects will understand the unique data types, workflows, and pain points of your sector. As one AI consultancy advises, evaluate the partner’s ability to understand your industry and adapt their models accordingly.
For instance, if you’re a bank aiming to implement AI for loan underwriting, a vendor who has successfully deployed similar solutions at other banks will hit the ground running.
1.2 Proven Model Performance
Insist on evidence of model quality. What accuracy or performance metrics can the vendor share from past deployments? Reputable AI firms should provide benchmarks or case studies – e.g. “our AI reduced fraudulent transaction losses by 60% for XYZ Bank” – and even be open to running a pilot using your data to prove their technology’s effectiveness. Because finance is data-intensive, check that their algorithms can handle large volumes of data and complex variables without degrading performance. A high-performing AI model in the lab means little if it chokes on real-world data feeds or produces too many false positives/negatives for practical use.
1.3 Customization & Flexibility
Avoid one-size-fits-all solutions. Your institution likely has proprietary data, unique processes, and specific objectives. An ideal AI partner will custom-tailor models or features to fit these needs. During due diligence, ask how much the solution can be configured or trained on your own data. Will you need data scientists in-house to tweak it, or does the vendor offer that service? The ability to fine-tune and the vendor’s willingness to co-develop solutions is a strong sign of technical partnership rather than just a product sale.
1.4 Tech Stack and Integration Capabilities
Another aspect of technical due diligence is examining the underlying technology stack. Does the vendor use modern, widely-supported programming languages and frameworks (like Python, TensorFlow/PyTorch, etc.), or obscure proprietary tech? A modern stack not only indicates the solution is up-to-date, but also that you’ll find it easier to hire talent to work with it or integrate it with other systems. Compatibility with your existing infrastructure (databases, core banking system, CRM, etc.) is crucial – the best AI model provides no value if it can’t plug into your workflows.
In summary, separating AI hype from reality starts with a hard look at technical competence. Ask for the technical documentation, talk to their engineers if possible, and ensure the partner truly has the “brainpower” (skilled talent and proven tech) to deliver results for a financial institution.
2. Regulatory Compliance & Security: No Compromises in a Regulated Industry
The finance sector operates under some of the most stringent regulatory regimes on the planet. Any AI partner must be fully aligned with regulatory compliance requirements and best-in-class security practices. This is not just a checkbox – it’s a make-or-break aspect of adopting AI in finance.
2.1 Navigating a Web of Regulations
Depending on your geography and sub-sector, you’re looking at compliance with laws and guidelines such as GDPR (data privacy for any EU customer data), GLBA (Gramm-Leach-Bliley Act for privacy in financial institutions), industry-specific rules from FINRA (for broker-dealers), SEC regulations (for investment and trading activities), OCC guidelines (for national banks), and others. In Europe, guidelines from the EBA (European Banking Authority) and upcoming EU AI Act also come into play. Your AI vendor doesn’t have to be a law firm, but they absolutely should demonstrate knowledge of these regulations and show how their product or service complies. In fact, regulators themselves are emphasizing this – FINRA’s 2025 report highlighted that firms must consider vendor risk and understand the type of AI usage in a vendor’s product to manage compliance.
If a prospective AI partner gives you a blank stare when you mention model governance under OCC Bulletin 2011-12/SR 11-7 (which outlines model risk management for banks), that’s a red flag.
2.2 Data Privacy and GDPR
Privacy regulations like GDPR in Europe and CCPA in California enforce strict rules on personal data handling. An AI solution often needs to consume large datasets, which may include sensitive customer information. Ensure the vendor follows “privacy by design” principles – meaning data is anonymized or pseudonymized where possible, usage is transparent and with consent, and they conduct impact assessments for data processing. GDPR non-compliance can lead to fines up to €20 million or 4% of global annual turnover, so any AI partner should treat customer data with the highest care.
Ask if they support data deletion requests, how they handle data ownership (your data should remain yours), and if they will use your data to train other clients’ models (ideally, no, unless properly anonymized and agreed upon).
2.3 Security Posture
Financial institutions are prime targets for cyber attacks, and introducing an AI vendor means a new potential attack surface. Evaluate the vendor’s cybersecurity measures. Are they compliant with standards like ISO 27001 or SOC 2 Type II? Do they encrypt data at rest and in transit? What is their breach history, if any, and how have they responded? Inquire about their access controls: who on their team can access your data or models? A top-tier AI provider should have clear answers, perhaps even offer to show you their pen-test results or certifications. Also consider location and cloud infrastructure – if using cloud AI services (e.g., on AWS, Azure, GCP), are data centers located in approved regions? Some banks require data residency (e.g., EU data stays in EU). Security and compliance go hand in hand: a breach not only causes operational damage but can quickly become a compliance violation too.
2.4 Model Governance and Transparency
Beyond IT security, there’s the concept of model risk management. Finance regulators expect that models (including AI/ML algorithms) that impact decision-making are subject to validation, documentation, and periodic review. Your AI partner should assist in this by providing documentation of model design, underlying assumptions, and testing results. Executives should ask: Does the vendor offer explainability tools so we can audit why the AI made a given prediction or recommendation? If the AI will, say, recommend investments to clients or approve loans, how can we ensure those decisions can be explained to satisfy compliance and consumer protection rules? The OCC, for example, has stressed that using AI doesn’t absolve a bank from understanding and managing the model’s output. Transparency from your vendor, via white-box models or explainability modules (like AI “X-ray” tools), is crucial.
In essence, choose an AI partner who treats compliance and security as first-class priorities, not afterthoughts. The vendor’s ability to enable safe and lawful AI deployment in your institution is as important as the features they promise.
3. Scalability & Future-Proofing: Go Big or Go Home (Safely)
When a financial institution invests in AI, the expectation is that successful projects will scale up across the enterprise and deliver value for years to come. Thus, an AI partner must offer solutions that are scalable and future-proof.
3.1 Enterprise Scalability
Start by assessing whether the vendor’s solution has been deployed at scale elsewhere. Can their platform handle the volume of data you have and the speed required? For example, if you are a retail bank considering an AI fraud detection system, can it instantly analyze millions of transactions per day without performance lags? If you’re a trading firm, can the AI model operate in real-time with low latency? Scalability isn’t only about computing power; it’s also about integration. The AI should integrate with multiple systems (core banking, risk management systems, CRMs, data lakes) across different departments. A scalable AI partner will have a robust API or middleware strategy to plug into your existing enterprise architecture.
3.2 Infrastructure and Cloud Strategy
Many AI solutions leverage cloud infrastructure for scalability. Clarify if the vendor uses a cloud-based SaaS, on-premises deployment, or a hybrid model. Each has implications: cloud can scale more easily and shift burdens away from your IT, but you must be comfortable with data in the cloud and vendor reliance on third-party cloud providers. Some banks opt for on-prem or private cloud AI deployments for sensitive use cases. Ensure the vendor’s offering aligns with your IT strategy and can scale accordingly – e.g., can it leverage distributed computing, add nodes or containers under high load, etc. Also verify that their tech stack is compatible with modern deployment methods (Dockers, Kubernetes, etc., for containerization if needed).
3.3 Future-Proofing through Innovation
The AI field evolves rapidly – what’s cutting-edge today might be table stakes next year. A future-proof partner should demonstrate that they keep pace with AI innovation. In practice, this could mean their platform is model-agnostic (you can update or swap out the machine learning algorithms without a complete overhaul) and they regularly update their software with improvements.
For instance, with the rise of Generative AI, if that becomes relevant for, say, generating personalized reports or doing advanced NLP on earnings calls, will your vendor be able to integrate those capabilities? In 2024 and beyond, we expect a wave of new AI techniques; your vendor’s R&D roadmap should show they won’t fall behind. One positive sign is if the vendor actively publishes research or contributes to the AI community, indicating they’re not just riding a single product but evolving with the tech.
3.4 Avoiding Vendor Lock-In
Future-proofing isn’t just technical – it’s also contractual and architectural. Many executives worry about vendor lock-in, where switching away from a chosen AI provider becomes prohibitively costly or technically impossible. To mitigate this, ensure the partnership gives you sufficient control. Do you retain ownership of the models or at least the data used and outputs generated? Can you export your data in a standard format easily? Is the AI built on open-source frameworks that your team could continue to use independently if needed? If the vendor runs the model as a black box and you have no artifacts if you part ways, you’re essentially handcuffed. Insist on contractual clauses that address portability (for example, the right to get all your trained model weights and code if the contract ends). A future-proof AI strategy maintains flexibility so that you can adapt suppliers in the future or even bring the capability in-house if that makes sense down the line.
3.5 Pilot to Production Path
Another angle of scalability is the vendor’s capability to support you from pilot to full production. Many AI engagements start as limited scope proofs-of-concept. But the transition to production at scale is where a lot of projects stumble (indeed, Gartner predicts that by 2025, 30% of generative AI projects will be abandoned at the pilot stage due to issues like poor data quality or inadequate risk management).
Choose a partner who has a clear methodology for scaling up – they should help define success criteria for the pilot and have a deployment plan that includes knowledge transfer, user training, and change management for your staff. Their experience in scaling AI in organizations will be invaluable to navigate common pitfalls (like model drift, performance issues, or user adoption challenges) that occur when moving from a lab environment to enterprise operations.
In short, think long-term: the AI partner should be able to grow with you. The solution must handle the size and complexity of your business not just now, but as you and the AI technology both evolve over the next 5+ years.
4. Cost Transparency & ROI: Counting the Cost of AI (Before It Counts You Out)
AI projects in finance can be expensive undertakings, so cost clarity and return on investment (ROI) are front-of-mind for any C-suite. A prudent executive will demand transparent pricing from AI vendors and have a realistic plan for ROI – and a good AI partner will actively help on both fronts.
4.1 Understand the Pricing Model
AI solution pricing can vary widely. It might be a subscription model, pay-per-use (e.g. per API call or per transaction analyzed), license fees for software, or even outcome-based pricing in some cases. During vendor evaluation, get a detailed breakdown of costs. For example, if a vendor offers an AI analytics platform, is the pricing tiered by number of users or data volume? Are there extra fees for premium features, additional data connectors, or support hours? Hidden fees are common gotchas – perhaps basic model deployment is included but each additional model or retraining cycle costs extra, or the price covers development but not maintenance or updates. Make sure everything is spelled out. It can help to ask for a sample total cost of ownership (TCO) for a scenario similar to your intended use. This should include one-time implementation fees, custom development costs, and recurring fees over, say, a 3-5 year period.
4.2 Budget for the Full Lifecycle
Often, the price quoted by a vendor is just the tip of the iceberg. Internally, you might need to budget for data preparation (cleaning and labeling data for the AI), new hardware or cloud resources, training your employees to work with the AI system, and integrating the AI outputs into your processes. There may also be costs for compliance (e.g., if you need to hire a consultant to validate the AI model or invest in an external audit). A savvy AI partner will acknowledge these and perhaps provide guidance or services to help (for instance, some vendors offer data onboarding as part of their package). When calculating ROI, include these costs to get a true picture of the investment.
4.3 Demand ROI Evidence and Tools
Executives should ask each vendor: “How will your solution drive ROI for us, and how soon?” While the specifics will depend on your use case (reducing manual labor, increasing revenue via better decisions, cutting losses via fraud detection, etc.), a credible vendor should offer a hypothesis and ideally evidence from other deployments. If they’ve served similar clients, what ROI did those clients see?
Was it in cost savings (like automating 30% of compliance checks) or revenue lift (like 15% more cross-sell to existing customers thanks to AI recommendations)? ROI can also come in non-tangible forms like improved customer satisfaction or faster reporting – these are valuable but try to quantify them (Net Promoter Score increase, report generation time cut by hours which frees staff for other tasks, etc.). Notably, according to a global CIO study, proving ROI remains one of the greatest barriers to AI adoption despite increased investment.
Knowing this, treat lofty ROI promises with healthy skepticism but also push vendors to help you make the business case. Some might have ROI calculators or be willing to do a pilot project where success criteria are tied to business outcomes.
4.4 Pilot Projects and Phased Investment
One best practice to manage cost and demonstrate ROI is to start with a pilot project or phased rollout. Many AI partners will support a paid pilot or a limited-scope implementation for a few months. Define clear metrics for success in this pilot (e.g., model accuracy above X%, processing time cut by Y%, or a specific dollar savings achieved). If the pilot meets targets, you then proceed to full deployment (and full payment). If not, you’ve limited your financial exposure. Ensure the vendor’s contract structure allows this kind of phased commitment. Avoid paying 100% upfront for an unproven solution; negotiate stage gates aligned with deliverables.
4.5 Transparency in Ongoing Costs
Once in production, what will it cost to keep the AI running? Ask about things like: Do we need to pay for model retraining as data drifts or new data comes in? How about support and maintenance fees after year 1? If the AI is delivered “as a service,” are costs predictable or could a spike in usage blow out our budget (common with cloud-based usage pricing)? Get clarity on how they will charge for upgrades or adding more users/use cases later. A truly transparent vendor will layout a multi-year cost expectation, not just the initial sale.
Finally, always tie cost discussions back to business value. It’s easy to get enamored by AI’s potential, but as an executive, keep asking “What’s the ROI? When do we break even?” A responsible AI partner will appreciate these questions and work with you to answer them, rather than dodge them. If they seem uncomfortable discussing ROI or TCO, take it as a warning sign – either they haven’t been around long enough to prove value, or they assume someone else in your organization will worry about the returns.
5. Vendor Reliability & Market Presence: Safeguarding Your Choice with a Stable Partner
When you choose an AI vendor, you’re entrusting them with critical aspects of your business – it’s akin to adding a new wing to your building. You want that wing built by a dependable architect, not someone who might skip town. Vendor reliability, reputation, and market presence are therefore vital components of due diligence.
5.1 Reputation and References
Start with the vendor’s reputation in the market. This can include formal evaluations (like Gartner Magic Quadrants, Forrester Waves, industry awards) and informal ones (word of mouth, media coverage). Have they been recognized as leaders in AI or fintech? A company that regularly publishes success stories or is featured in reputable industry publications likely has a record to back their promises. Client testimonials and case studies are especially telling – as noted, checking these can give insight into a vendor’s real-world performance.
Don’t hesitate to ask the vendor for references. Speaking directly to a current customer of the vendor (with similar scale or in a related industry) can yield candid insights: Are they satisfied with the support? Did the implementation meet expectations? Any hidden challenges?
5.2 Financial Stability
Assess the vendor’s financial health. If it’s a large established firm (think Microsoft, Google, IBM, or even big financial core technology providers like FIS, Fiserv, SAS), they’re likely stable – but confirm the AI product line you’re interested in is strategic for them (sometimes big companies kill off smaller product lines). If it’s a startup or mid-size company, do they have solid funding or revenue? Research their investors, profitability, and growth trend. The risk with smaller vendors is that they might get acquired by another company (and your project’s direction could change), or worse, run out of funds. Interestingly, sometimes an acquisition can be a positive (if a larger firm will continue the service), but it can also lead to discontinuation if the product is absorbed. Consider including contract clauses about what happens if they are acquired or if key personnel leave.
5.3 Expertise of Team
The caliber of the vendor’s team contributes to reliability. Who are the leaders and engineers? Do they have experienced data scientists, ideally with backgrounds in both AI and finance? If the vendor has a dedicated team for financial services clients, that’s a plus. Also, gauge their capacity – do they have enough staff to support your project in the timeline needed? A small vendor might have great tech but could be overloaded if they take on multiple big bank clients at once.
5.4 Market Presence and Ecosystem
It can be revealing to see what partnerships or ecosystem the AI vendor is part of. For example, are they partnering with major consulting firms (Accenture, Deloitte, etc.) to implement their solutions? Are they on the marketplace of major cloud providers (AWS, Azure) as a certified solution? A strong presence in the ecosystem means more resources and community knowledge around their product. It also means if issues arise, there may be third-party expertise available to help (e.g., consultants familiar with that tool). Conversely, an isolated player might leave you reliant solely on them for all support and enhancements.
5.5 Cultural Fit and Support
Don’t overlook softer aspects like cultural fit and support reliability. If you’re a highly regulated bank that moves carefully, a move-fast-and-break-things startup vendor might frustrate you (and vice versa). During the vetting process, note how responsive and transparent the vendor is. Are they willing to adapt to your needs or do they push a standard approach? How they behave in pre-contract discussions can indicate what service will be like post-contract. Ensure they offer a support structure that meets your needs (dedicated account manager, 24/7 support if your operations are around the clock, etc.). Especially in finance, if an AI system is critical (say it’s flagging fraudulent transactions or ensuring compliance), you need the vendor to be available immediately if something goes wrong.
In short, do your homework on the vendor as rigorously as you’d do on a major investment or a new hire. You want a partner with a solid past, a stable present, and a promising future.
6. Risk Mitigation & Ethical AI: Ensuring a Responsible AI Deployment
Adopting AI in finance comes with a set of risks – some known and quantifiable, others emerging and reputational. A responsible AI partner should not only excel when things go right, but also have your back when things go wrong. This means a focus on risk mitigation, ethical AI practices, and a plan to handle the unexpected.
6.1 Bias and Fairness Checks
One of the biggest ethical concerns with AI, especially in finance, is bias. AI models trained on historical financial data might inadvertently perpetuate or even amplify biases – for example, in lending, if historical data is biased against a certain group, a naive AI model could end up systematically denying credit to that group, leading to discrimination. The consequences are not just ethical; they are legal (violating fair lending laws, for instance) and reputational. During due diligence, probe the vendor’s approach to mitigating bias. Do they conduct bias audits on their models? Are they knowledgeable about techniques like bias correction, diverse training data sets, or algorithmic fairness metrics? “Because AI models make predictions based on datasets and assumptions, their results carry a risk of being skewed due to error and bias,” as risk experts note.
A vendor that acknowledges this will have strategies to address bias – such as including explainable AI that can highlight potential bias, or allowing you to set thresholds to override model decisions that look unfair.
6.2 Explainability and Transparency
Hand-in-hand with fairness is the need for explainable AI. In finance, you’ll often need to explain decisions to regulators, customers, or internal audit. If an AI model is a complete “black box”, it might be unacceptable for high-stakes decisions (imagine trying to explain to the SEC why your trading AI made a certain trade that caused market impact, if you yourself don’t know). Many AI vendors now offer tools for explainability – for example, feature importance charts, natural language explanations of decisions, or the ability to trace which data influenced an outcome. Ensure any vendor you consider has some level of transparency in their solution. Some newer AI techniques like deep learning are inherently less interpretable, so if they use them, ask how they mitigate that (sometimes through surrogate models or frameworks like LIME/SHAP for local explanations). Regulators are increasingly skeptical of unchecked AI, and having interpretability built in is a strong sign of an ethical, risk-conscious AI partner.
6.3 Robust Testing & Model Risk Management
Financial firms are familiar with stress testing and scenario analysis – your AI models should undergo similar rigor. What testing does the vendor do before deploying a model? Do they simulate edge cases or rare events? For example, if you’re implementing an AI for market risk, has it been tested on extreme market swings (e.g., 2008 crisis data)? If it’s a fraud detection AI, does it update quickly when new fraud patterns emerge? Moreover, regulators like the OCC and Federal Reserve (in the US) expect that banks treat third-party models like their own in terms of model risk management – meaning initial validation, ongoing monitoring, and periodic validation. The vendor should provide you with documentation and access to the model necessary for your risk teams to validate it. If a vendor has been through a bank’s model validation process before, that’s a big plus (they’ll know the drill, like providing confusion matrices, performance on different segments, etc.).
6.4 Incident Response and Accountability
Discuss what happens if the AI makes a mistake or fails. How does the vendor define and handle “incidents”? For instance, if an AI-driven trading system goes awry, or an AI credit model mistakenly declines a set of applications it shouldn’t have, what support will the vendor provide? Do they have a 24/7 crisis team? Will they help in forensic analysis of the model’s behavior? While the financial institution ultimately bears the regulatory responsibility, a good partner stands with you in resolving issues. Some advanced AI firms even implement kill switches or fallbacks – if the model’s output seems outside normal bounds, the system can automatically revert to a safe default or human review. Understand if such features exist and how you can control them.
6.5 Ethical AI Principles
It’s worth checking if the vendor publicly subscribes to any ethical AI principles. Many companies have developed AI ethics charters (e.g., Google’s AI principles, Microsoft’s responsible AI framework). While those are sometimes broad, they at least indicate the company culture prioritizes doing the right thing. For example, principles might include commitments to transparency, privacy, fairness, and accountability. If your organization has its own AI ethics guidelines, even better – share them and see if the vendor can comply. Ethics also extend to use cases: ensure the vendor’s technology isn’t being repurposed in ways contrary to your values (for instance, you wouldn’t want your vendor using your partnership as an excuse to develop AI for something unethical elsewhere).
6.6 Regulatory and Legal Risk Mitigation
Because this is finance, consider legal terms that mitigate risk: indemnities for data breaches caused by the vendor, intellectual property assurances (who owns the developed models or any derivatives?), and compliance guarantees where possible. It may not always be possible to get a vendor to take on regulatory liability, but you can often include clauses that require them to adhere to all applicable laws and assist you in compliance efforts. Also, ensure they will accommodate regulatory exams – e.g., if the SEC or OCC wants to examine the model or how it’s governed, the vendor should cooperate.
In summary, look for an AI partner who proactively addresses risks and ethics rather than one who says “we just provide the tech, it’s up to you how it’s used.” In a tightly regulated space, you need a partner who is as cautious and prepared as you are.
7. Industry Context: The Competitive Landscape of AI Vendors in Finance
The pool of AI vendors and partners is vast and diverse. Understanding the landscape will provide context to your evaluation and help differentiate between providers.
7.1 Big Tech & Cloud Providers
Companies like Google, Amazon, Microsoft, and IBM have robust AI offerings tailored to industry, including finance. Google Cloud’s AI tools, Amazon Web Services (AWS) machine learning services, Microsoft Azure’s AI suite, and IBM’s Watson and financial services cloud offerings are all examples. These vendors bring massive scalability, strong R&D, and often built-in compliance and security features. They are reliable in the sense of stability, but one consideration is that you may be a smaller fish in a big pond to them. Ensure you will get sufficient attention and customization – often these giants work through integration partners or consultants to deliver solutions to end clients. One advantage though is these companies are often leaders in AI research, so their products may incorporate the latest techniques (and they likely won’t go out of business).
7.2 Finance-Focused AI Startups and Specialists
The last decade has seen many fintech AI startups emerge, targeting niches like algorithmic trading, credit scoring, fraud prevention, wealth management robo-advisors, or customer service chatbots for banks. Examples include companies like Zest AI (for underwriting), Ayasdi (now Symphony AyasdiAI, for AML and fraud), DataRobot (automated machine learning, used in some financial contexts), Onfido or Jumio (for AI-powered identity verification in fintech), etc. These specialized vendors often deeply understand a particular problem and can have very tailored solutions. They might offer faster deployment and more hand-holding. The trade-off is their size and longevity – you need to vet their stability and possibly their interoperability (e.g., a niche product that doesn’t play well with others can be a silo).
7.3 Consulting and System Integrators
Major consulting firms (Accenture, Deloitte, PwC, EY, McKinsey, BCG) and IT integrators (Infosys, TCS, Capgemini, etc.) also play a role. While they may not sell a proprietary AI product (though some have frameworks and tools), they often partner with AI technology providers to deliver end-to-end solutions. For a large bank, sometimes the “AI partner” is actually a combination of a technology vendor and a consulting firm implementing it. The benefit of involving such firms is their broad experience in transformation and risk management; they can help you evaluate vendors impartially and integrate the chosen solution smoothly. If you lack internal expertise to manage an AI project, bringing in an external consultant can de-risk the implementation. However, this adds to cost, and you need to ensure clarity of responsibility (the consulting firm might push their preferred vendor, so be sure that choice is indeed best for you).
7.4 Competition and Innovation
The competitive environment among vendors can be used to your advantage. If you’re evaluating multiple providers (which you should – never single-source such a critical component without alternatives), let them know it’s a competitive process. You might find they offer better pricing or terms to win your business. Also, ask each what differentiates them from others – their answers can illuminate strengths or reveal weaknesses. For instance, one might emphasize their model’s superior accuracy, another their ease of integration, another their compliance credentials. Align those with what matters most to you.
7.5 Staying Informed
The market is continually changing – new startups appear, and sometimes yesterday’s leaders fall behind. Use resources like industry analyst reports, fintech accelerators, and networking with peers at other institutions to keep updated on who’s delivering real results. It’s a good idea to attend finance and AI conferences or webinars where banks share case studies; you may hear which vendors they partnered with and lessons learned. Being informed helps you cut through vendor marketing – you’ll know, for example, if a certain AI platform has a reputation for being user-friendly or if another is known for strong regulatory compliance features.
8. Strategic Recommendations for Executives
Selecting and onboarding an AI partner in a financial enterprise is a strategic initiative. Here are some high-level recommendations and best practices to ensure success:
Adopt a Cross-Functional Approach: As mentioned in the presentation section, bring together stakeholders from IT, data science, compliance, legal, risk, and business units when evaluating vendors. This ensures all angles are covered – technology fit, regulatory issues, user needs, etc. It also builds buy-in across the organization for the chosen solution.
Define Clear Objectives and KPIs: Before or during vendor selection, get specific on what you want to achieve. “Improve fraud detection” is a goal, but a clearer objective would be “detect 50% more fraud cases in the credit card portfolio within 6 months of implementation while reducing false positives.” Having this clarity not only guides vendor selection (you’ll pick the one that can meet that), but also sets the stage for measuring success and ROI.
Beware of the PoC Trap (Proof of Concept vs. Production): Many financial firms spin up impressive proofs of concept with AI that never see the light of day in production (recall the Gartner stat that a large chunk of AI projects stall at pilot
). To avoid this, treat the PoC as if it were an initial deployment: involve end-users early, test integration with real data streams, and plan how you’d deploy at scale if it works. Choose a vendor who is enthusiastic and capable about getting you to production, not just winning a PoC then leaving the hard scaling work to you.
Contractual Safeguards: Negotiate a contract that protects you. Key things to consider: a) Service level agreements (SLAs) for performance and uptime if it’s a service (you might need near-100% uptime for critical systems); b) Data ownership clearly spelled out; c) clauses on exit and transition assistance (as discussed, to avoid lock-in); d) requirements around compliance (perhaps the vendor must undergo annual audits or compliance reviews); e) Liability and indemnification (if their tool causes a big issue, do they share any liability? Often vendors limit this heavily, but get as much accountability as you can). It’s worth getting your legal counsel involved early to identify any red flags in vendor contracts, especially with newer AI firms that might not have experience with banking contracts.
Governance and Monitoring: Set up a governance structure for the AI initiative. Perhaps a steering committee that includes C-suite sponsors (CIO/CTO, CFO, Chief Risk Officer, etc.) meets regularly with the project team and vendor to review progress and risks. Also plan for post-implementation monitoring: define who will watch model performance metrics, how often you retrain or update the model, and how you’ll handle model validation annually. The idea is to treat the AI system as a living part of your operations that requires oversight – much like you’d monitor and maintain any critical system (or even more so, given AI can change as data changes).
Change Management & Training: Even the best AI solution can fail if your people don’t use it or trust it. Ensure the vendor provides (or you invest in) training programs for end-users and administrators. For example, if branch managers will get AI-driven insights for cross-selling, train them on how to interpret and use those insights. C-suite should champion the adoption, communicating the strategic importance of the AI partnership and setting the tone that it’s here to augment staff, not replace or confuse them. Encourage an organizational culture that sees AI as a tool to leverage, but also empower employees to question or override the AI when it doesn’t make sense – that balance is key to building trust in the system.
10. Conclusion
Selecting an AI partner in the finance sector is one of those high-stakes decisions that requires both strategic vision and diligent scrutiny. The right choice can empower your organization with transformative capabilities – from hyper-efficient operations to data-driven insights that drive competitive advantage. The wrong choice, however, can lead to wasted resources, regulatory troubles, and reputational harm.
As a C-suite leader, approach this decision with a balanced mindset: enthusiastic about AI’s potential, yet grounded in the practical realities of your industry’s demands. Use the criteria discussed – technical excellence, compliance, scalability, cost clarity, vendor reliability, and ethical risk management – as your compass. Engage your teams and perhaps external experts to evaluate vendors against these benchmarks thoroughly. In doing so, you will differentiate between mere AI hype and solutions that truly deliver value in the real world of finance.
Ultimately, a successful AI partnership in finance is one where technology and business strategy align. It’s a partnership where both parties are committed to innovation, compliant growth, and constant learning. With careful due diligence and a clear focus on your strategic goals, you can forge an AI alliance that propels your financial institution into the future, confidently and responsibly.
9. Key Takeaways for Finance Executives
Substance Over Hype: In a landscape flooded with AI buzzwords, focus on partners who demonstrate real-world impact in finance. Remember that as many as 85% of AI projects can fail to deliver if not properly executed, so choose a vendor with a track record of success, not just promises.
Regulatory Compliance is Mandatory, Not Optional: Ensure any AI partner is prepared to meet all relevant financial regulations and privacy laws, and has robust security and risk controls in place. Regulatory missteps can result in fines or project shutdowns, so compliance and AI governance must be baked into the solution from day one.
Avoid Lock-In – Keep Control of Your Destiny: Be wary of becoming too dependent on one vendor’s proprietary systems. Opt for solutions that allow you to retain ownership of data and models, export your data easily, and switch providers if needed. This flexibility safeguards your control over AI implementations and prevents being stuck with a partner that isn’t meeting expectations.
Long-Term Value and Impact: Evaluate AI partnerships on their long-term financial and operational impact. This means looking beyond initial costs to total cost of ownership, ensuring the solution can scale with your growth, and that it will deliver sustainable ROI. Also consider organizational readiness – the best AI partnership includes a plan for your team to effectively use and maintain the AI system over time.
Turn AI into ROI — Win Faster with HIGTM.
Consult with us to discuss how to manage and grow your business operations with AI.
© 2025 HIGTM. All rights reserved.