34. Data Governance Basics – Ownership, Security, Privacy
Data is a powerful asset for small and medium-sized enterprises, but only if it’s well-managed, properly secured, and handled ethically. That management requires data governance—the framework that assigns roles, enforces security, and ensures privacy compliance. This article explains why data governance matters, the key pillars involved, and practical methods for SMEs to uphold robust standards. By clarifying ownership, safeguarding information, and respecting user rights, your organization protects itself from chaos or legal pitfalls and taps into data’s true potential.
Q1: FOUNDATIONS OF AI IN SME MANAGEMENT - CHAPTER 2 (DAYS 32–59): DATA & TECH READINESS
Gary Stoyanov PhD
2/3/20256 min read
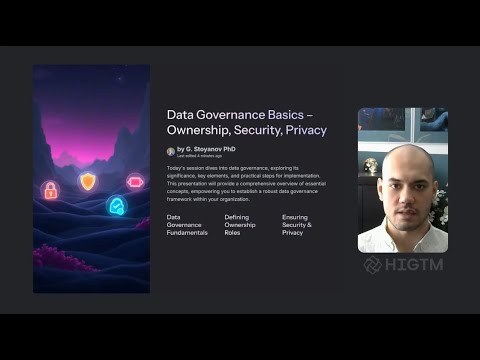
1. Understanding Data Governance
1.1 Definition
Data governance is the set of policies and processes that control how data is collected, stored, shared, and protected. It standardizes naming, enforces roles (like data owners or stewards), and secures info from misuse or breaches.
1.2 Why SMEs Need It
Some smaller companies believe governance is for big enterprises. Yet disorganized data or privacy mishaps can harm any business. A concise policy prevents confusion among teams, satisfies regulations, and builds a stable foundation for AI or analytics.
1.3 Key Outcomes
Improved Data Quality: Less duplication or conflicting records.
Clear Accountability: Everyone knows who updates each dataset.
Enhanced Security & Trust: Reduced risk of leaks, stronger customer confidence.
Better Alignment with Goals: Data remains relevant for your objectives, not random or siloed.
2. Core Elements of Data Governance
2.1 Ownership & Accountability
Data Owners: Typically managers in each department, ensuring correctness and completeness for the datasets they create or oversee.
Data Stewards: Handle daily tasks, like removing duplicates or correcting errors. This role can also monitor compliance with naming conventions.
2.2 Security & Access Control
Role-Based Access: Staff see only the data they need for their role. For instance, a marketing associate sees campaign leads, finance staff see financial statements, etc.
Encryption & Secure Storage: Tools such as SSL encryption for data in transit, and possible disk-level encryption for data at rest.
Regular Audits: Periodic checks to confirm correct permissions and watch for suspicious activity.
2.3 Privacy & Compliance
Legal Requirements: Laws like GDPR in Europe or CCPA in California demand clarity on how personal data is used.
Consent & Transparency: Users need to understand what’s collected, why, and how to opt out or request removal.
Handling Requests: Businesses must process data subject requests—like people asking to see or delete their records—within specified time frames.
2.4 Data Usage Policies
Approved vs. Prohibited: Clarify which tasks data can support (like marketing analytics) or which manipulations are off-limits (like using personal data for unrelated tracking).
Version Control: Track policy changes over time. If you allow data usage expansions (like adding a new analytics feature), update the policy so staff remains in sync.
3. Defining Ownership Roles
3.1 Importance of Clearly Assigned Owners
Without a defined owner, no one feels responsible for data quality or updates. Ambiguities cause missing fields, duplication, or incorrect data. By naming an owner (like a department head), you ensure someone stands guard.
3.2 Data Owners vs. Data Stewards
Data owners set guidelines for usage, might approve new data fields, or decide on retention periods. Data stewards handle the ongoing maintenance—like verifying entries, removing or correcting duplicates, or running monthly checks to confirm accuracy.
3.3 Cross-Departmental Collaboration
Some datasets overlap, for example: marketing and sales teams might share customer leads. The data owners coordinate to ensure consistency across systems, so each department’s version of “customer info” is the same.
4. Security Measures & Access Control
4.1 Role-Based Access
Granting each role the minimal data they need prevents accidental disclosure or internal misuse. For example, an HR manager has access to employee records but not necessarily all financial transactions.
4.2 Encryption & Data Backup
If a breach occurs, encryption ensures hackers can’t read plain text. Frequent backups guard against system failures or ransomware attacks. Storing backup copies offsite or in the cloud with secure credentials is vital.
4.3 Monitoring & Alerts
Systems logging user access or unusual data queries help catch issues early. For instance, a sudden spike in data exports from a non-technical user might signal suspicious activity or a compromised account.
5. Privacy & Regulatory Compliance
5.1 GDPR, CCPA, and More
Regulations differ by region, but the principles remain similar: collect data only with valid reasons, obtain consent where required, and be transparent about usage. Even SMEs must comply if they handle personal data from those jurisdictions.
5.2 Consent & Transparency
Tell users precisely why you’re collecting each piece of data and how you store it. Provide an easy path for them to opt out of or remove personal data. This transparency fosters trust, a huge factor in brand loyalty.
5.3 Handling Data Subject Requests
A robust governance plan includes steps for verifying someone’s identity and retrieving or deleting records if they request it. Building automation or a quick manual process helps you meet legal deadlines and maintain good user relations.
6. Avoiding Common Pitfalls in Governance
6.1 Overly Strict or Rigid Policies
Rules that hamper daily tasks may cause employees to bypass them. Aim for a balance: strong security, but no endless bureaucracy or multi-layer approvals for routine data queries.
6.2 Siloed Departmental Data
One team might run its own mini-database with little oversight or naming consistency. If each department hoards data, your business loses synergy. A central repository or at least a consistent standard helps unify efforts.
6.3 Neglecting Regular Updates
Business evolves—new product lines, expansions, or acquisitions. Unless you periodically review and tweak governance, you risk outdated policies that mismatch current operations or compliance requirements.
7. Real-World Examples
7.1 Retail Chain with Unified Customer Records
Multiple store locations meant scattered loyalty info. Once they named a data owner for customer profiles and enforced standard fields (e.g., full name, phone, email), plus set role-based access, each store read from the same master data. This approach cut double entries, improved email campaigns, and supported AI-based personalization.
7.2 Logistics SME with Secure Tracking
A logistics provider installed sensors on trucks. Initially, data was accessible to all, raising security concerns (and driver privacy issues). By introducing role-based access and setting data stewards for location logs, they restricted who could see sensitive routes. This reduced data mismanagement and aligned with driver consent forms.
7.3 Online Education Startup
This startup collected user signups globally, inadvertently storing personal details without explicit consent. After a small compliance audit, they updated consent forms, separated personal info from usage logs, and encrypted user IDs. This shift built user trust and cleared them for future expansions into AI-driven course suggestions.
8. Integrating Governance into Your AI Projects
8.1 Setting the Stage for AI
Governance ensures consistent data labeling or robust privacy rules. AI models flourish with accurate input; random or unverified data leads to subpar predictions. A well-structured approach saves time and headaches.
8.2 Minimizing Ethical & Bias Risks
Clear governance reveals how data is gathered and used in AI models. If certain fields risk discriminatory outputs (like user ethnicity or sensitive categories), data owners can exclude them or apply special checks.
8.3 Model Lifecycle Management
An MLOps engineer benefits when data pipelines remain stable and consistent. Data stewards confirm that new data fields introduced for model training abide by privacy constraints or do not compromise user rights.
9. Steps to Build or Improve Data Governance
Conduct a Data Audit
List major datasets (sales, marketing, operations, HR).
Note who’s currently in charge or whether no one is.
Assign Ownership & Stewardship
Data owners set usage rules.
Data stewards maintain daily accuracy, run checks, or respond to queries.
Draft Governance Policies
Clarify classification (public, internal, sensitive).
Outline how each classification is stored and who can view it.
Implement Security & Privacy Measures
Encrypt sensitive data.
Use role-based access.
Comply with GDPR/CCPA or relevant regional laws.
Train & Communicate
Hold short sessions ensuring staff know the new structure.
Provide simple guidelines or a data dictionary to help them follow best practices.
Monitor & Update
Schedule periodic audits.
Adjust as your enterprise or regulations evolve.
10. Measuring Success in Data Governance
10.1 Fewer Data Errors
Check monthly or quarterly error rates, duplicates, or incomplete fields. A downward trend signals better quality.
10.2 Compliance Confidence
If your SME can handle data subject requests swiftly (like retrieving or erasing personal info), you stand on solid compliance footing.
10.3 Team Feedback
Ask whether staff find it easier to locate accurate data. A positive response implies stronger governance.
10.4 Smooth AI Integrations
If data sets are consistent, your AI projects see fewer roadblocks. That success acts as a strong indicator that governance is working.
Data governance weaves ownership, security, and privacy into a coherent framework that protects information, upholds regulations, and boosts trust among customers and employees alike.
By assigning clear roles, building robust security measures, and respecting privacy mandates, your SME establishes a structured environment where data aids, rather than hinders, growth.
If your aim is to leverage AI or advanced analytics, these governance basics become nonnegotiable foundations—keeping data quality high, moral risks low, and value potential soaring.
Turn AI into ROI — Win Faster with HIGTM.
Consult with us to discuss how to manage and grow your business operations with AI.
© 2025 HIGTM. All rights reserved.