33. Creating a Data Strategy – Aligning with Business Goals
Data can illuminate hidden patterns, drive AI solutions, and boost revenue—but only if it’s managed with specific aims in mind. A random approach to data leads to wasted resources and frustrated teams. By creating a focused data strategy that aligns with your business goals, you transform raw information into actionable insights. This article walks through each step: from defining goals and mapping sources to implementing governance and measuring outcomes.
Q1: FOUNDATIONS OF AI IN SME MANAGEMENT - CHAPTER 2 (DAYS 32–59): DATA & TECH READINESS
Gary Stoyanov PhD
2/2/20255 min read
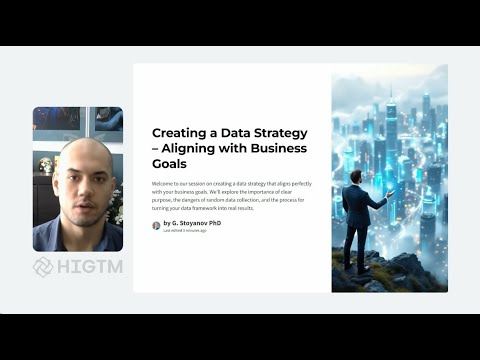
1. Why Align Data with Business Goals?
1.1 Avoiding Data Overload
Information overload is real. Many companies collect every variable or user click they can, hoping something yields insights. But data lacking purpose becomes noise. Instead, align data-gathering efforts with goals—like improving customer retention or identifying high-margin products—so each piece of data you collect has a reason.
1.2 Transforming Insights into Action
When data matches your strategic aims, gleaned insights quickly tie back to revenue or cost-reduction measures. If an analysis reveals that weekday promotions don’t lift sales, you can respond by shifting resources to weekends. That direct link from data to decision-making ensures you see tangible results.
1.3 Building Trust & Efficiency
If teams see data leading to real improvements—like faster shipping or personalized offers—they’ll trust the data strategy and contribute more. Misaligned data sets that produce irrelevant dashboards drain morale. By tying data tasks to key performance indicators (KPIs), employees view them as purposeful.
2. Components of a Well-Aligned Data Strategy
Clear Business Objectives
Examples: “Reduce average support call time by 20%” or “Increase average cart value by 10%.”
Each objective becomes the guiding star that dictates which data you gather.
Data Source Identification
Know where relevant info exists: CRM logs, website analytics, offline records, or third-party APIs.
Evaluate consistency and reliability (are these sources up-to-date?).
Data Governance & Ownership
Define policies for data collection, usage, and security.
Assign owners for each dataset—like a marketing manager for campaign data or an operations lead for supply chain records.
Infrastructure & Tools
Depending on your scale, choose data storage (cloud vs. on-prem) and analytics frameworks that match your staff’s skill set.
For smaller teams, a robust spreadsheet system or a lightweight database might suffice if well-structured.
Implementation Roadmap
Outline phases for rolling out data-driven processes or AI projects.
Start with small pilots to show viability, then scale once you see stable gains.
3. Steps to Link Data Strategy to Specific Goals
3.1 Spell Out the Question
Frame your main question: “How can we improve product recommendations to boost cart size?” or “Which marketing channel yields highest net profit?” This question drives data collection (like user browsing histories or cost-of-acquisition logs).
3.2 Map Needed Datasets
Identify each relevant dataset, whether it’s from your e-commerce platform or finance system. Decide if you must unify them in a single repository or can analyze them in separate but coordinated systems.
3.3 Assess Data Quality & Gaps
Clean up duplicates or incomplete records. If essential data is missing—like user location—decide if it’s truly needed. Overfilling forms or forcing data collection might hamper user experiences.
3.4 Evaluate Feasibility
Check staff skills, tools, and budgets to confirm you can handle the data tasks. If you lack certain capabilities, plan to upskill or hire a consultant.
3.5 Implement & Review
Execute your plan in sprints or phases, updating stakeholders regularly on findings. Each outcome or insight should directly tie back to the initial goal.
4. Avoiding Scattershot Data Collection
4.1 The Problem with “Collect Everything”
A data lake that holds every possible point—like user screen resolutions, times of day, random device stats—often leads to confusion. You end up paying for storage but rarely using those fields. It also raises compliance risks: more data means more potential for breaches or regulatory fines.
4.2 Prioritize Relevance
Focus on fields that relate to core metrics. If you want to reduce delivery times, gather shipping logs, driver routes, and possibly traffic data if relevant. Let go of the rest.
4.3 Balancing Depth & Simplicity
Some metrics, like “clicks per product page,” are easy to collect. Others, like “detailed user sentiment,” can be trickier, requiring advanced text analysis. Start with simpler, high-impact metrics before adding complexity.
5. Challenges & How to Overcome Them
5.1 Inconsistent Data Definitions
One department calls a product “SKU #123,” another calls it “Blue T-shirt.” Align naming conventions to avoid confusion. A data dictionary listing each field and meaning fosters clarity.
5.2 Frequent Changes in Business Goals
Corporate priorities shift—maybe you pivot to a new product line mid-project. Keep your data strategy flexible. Make sure your infrastructure can adapt to new data types or expansions.
5.3 Lack of Stakeholder Involvement
If data initiatives run in isolation, you risk building analytics that no one uses. Include key stakeholders from finance, sales, or marketing to help shape KPI definitions and ensure the data approach suits real needs.
6. Real-World Illustrations
6.1 Online Retailer
They aimed to reduce cart abandonment. The data strategy centered on capturing browsing history, cart additions, and exit pages. Analyzing these details, they found certain shipping rate structures caused most drop-offs—leading them to simplify shipping tiers. The result: higher conversion rates.
6.2 Regional Logistics Company
Sought to cut late deliveries by 25%. They mapped route data, driver schedules, and traffic patterns, storing them in a central system for quick analysis. AI pilots used this data to optimize routes. Clear alignment with the main goal prevented random data bloat.
6.3 Local Healthcare Clinic
Wanted to raise patient satisfaction scores. The data approach collected wait times, staff availability, and appointment patterns. By analyzing these trends, the clinic adjusted staffing hours, trimming average wait times—improving both patient contentment and service flow.
7. Infrastructure & Tool Selection
7.1 Matching Tools to Team Skills
Choose analytics software your staff can operate. A highly complex data warehouse might prove overkill if your staff just needs moderate reporting. Alternatively, advanced AI solutions could be worth it if you aim for big gains or have staff/consultants ready to manage them.
7.2 Cloud vs. On-Prem
Cloud solutions often suit SMEs wanting scalable, pay-as-you-go options. On-prem might be chosen for data security or compliance. Hybrid can merge both worlds—keeping sensitive data local while hosting less critical data in the cloud for easy analytics.
7.3 Handling Real-Time vs. Batch Updates
If immediate data (like live e-commerce events) is vital, pick systems that refresh frequently. For slower processes—like monthly financial statements—batch updates suffice. Match refresh speeds to actual business rhythms to keep overhead low.
8. Cultural Considerations
8.1 Gaining Cross-Team Input
Data strategies grow stronger when each department shares input on which metrics matter. Marketing might want lead conversion data, while operations need accurate supply chain logs. Integrate these needs early.
8.2 Training & Accessibility
User-friendly dashboards or data exploration tools let more employees leverage insights. Provide short training sessions showing how to read or interpret the data, fostering broad data literacy.
8.3 Feedback Loops
Encourage staff to highlight data inconsistencies or request new fields if they see missing links. This feedback loop ensures continuous improvement and engagement.
9. Measuring Success of Your Data Strategy
Goal Achievement: If your main objective was boosting repeated purchases by 10%, check if that happened after implementing your new data approach.
Data Quality Metrics: Look at data completeness, error rates, or duplication levels. Lowering these indicates better governance.
System Adoption: Are staff using dashboards? Are managers referencing data in planning meetings? High usage shows your strategy resonates.
Project Timeline: Evaluate if data tasks run on schedule. If constant rework or confusion arises, revisit your plan.
10. Maintaining & Evolving Your Strategy
10.1 Regular Audits
Check data sources every quarter or year. Confirm they remain accurate, relevant, and well-structured. If your business pivoted to new products, add or remove fields accordingly.
10.2 Ongoing Staff Development
As your data grows, your team’s skill set must evolve. Offer advanced workshops on BI tools, or provide data best practice refreshers. This ensures each new wave of employees or updated systems integrates smoothly.
10.3 Tying Strategy to Future AI
Your data roadmap might eventually feed AI pilots—for example, predictive analytics or personalized marketing. Continual refinement keeps data “AI-ready” whenever new opportunities arise.
A sound data strategy locks onto genuine business needs—boosting revenue, refining operations, or delighting customers. By defining objectives, cleaning data sources, installing governance, and selecting suitable tools, you pave the way for meaningful analytics or AI outcomes.
The real power of data emerges when each data point has a purpose, each system fits into a bigger plan, and each employee knows how to leverage these insights.
Let data become your currency—spent wisely for tangible returns.
Turn AI into ROI — Win Faster with HIGTM.
Consult with us to discuss how to manage and grow your business operations with AI.
© 2025 HIGTM. All rights reserved.