26. Early Metrics to Track – Simple KPIs for AI Pilot Projects
Small and medium-sized enterprises often dive into Artificial Intelligence with enthusiasm, hoping to see fast outcomes. During these early stages, confusion can arise about what to measure and how to interpret results. Large businesses might have entire analytics teams and advanced dashboards, but SMEs may not. This article explores simple, clear metrics (Key Performance Indicators, or KPIs) that help confirm whether an AI pilot project is heading toward success, even with limited resources.
Q1: FOUNDATIONS OF AI IN SME MANAGEMENT - CHAPTER 1 (DAYS 1–31): CORE AI CONCEPTS & VALUE PROPOSITION
Gary Stoyanov PhD
1/26/20256 min read
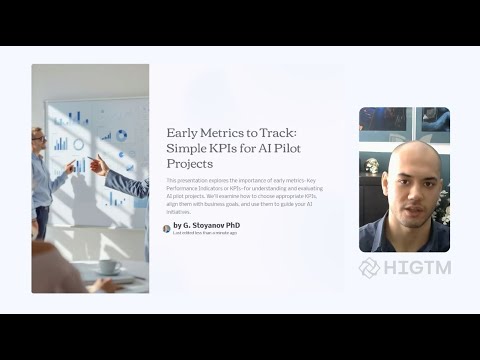
1. Why Simple KPIs Matter
1.1 Clarity in Uncertain Times
Early AI pilots involve new data streams, untested tools, and employees still learning how AI fits in. Straightforward KPIs offer a ground-level view of what’s happening.
1.2 Building Trust
Early-stage AI can provoke skepticism among managers or frontline staff. By showing immediate wins—like a small cut in processing time—teams are more likely to stay on board.
1.3 Reducing Overhead
Minimal or moderate metrics keep data collection costs down. SMEs may not afford heavy analytics software or entire business intelligence platforms. That’s fine if you track a handful of well-chosen indicators.
2. Setting Foundations for KPI Selection
2.1 Define the Pilot’s Core Objective
If the pilot aims to automate part of customer service, measure call-handling speed or satisfaction scores.
If it’s a demand forecast tool, track the difference between predicted and actual sales.
2.2 Involve the Right People
An operations manager might highlight daily throughput, while a customer support lead might emphasize satisfaction scores. Each role can bring unique KPI suggestions.
2.3 Align with Real-World Impact
Ensure the KPI you pick reflects tangible outcomes. Speeding up a process by 20% or boosting customer retention by 5% can connect directly to cost savings or revenue.
3. Examples of Simple KPIs
3.1 Accuracy Rate
What It Means: How often the AI model produces a correct prediction, classification, or recommendation.
Why It’s Important: Accuracy builds trust. A chatbot with a 30% accuracy in answering questions might frustrate customers, whereas 80% or higher could signal readiness for a broader rollout.
Caution: Don’t chase 100% right away. Early pilots often settle for partial gains.
3.2 Processing Time Reduction
What It Means: The change in how long a task takes before and after AI adoption.
Why It’s Important: Saving staff time is a direct cost or efficiency metric. If data entry tasks drop from 15 minutes to 10 minutes, you free up resources for more strategic work.
Caution: Factor in the learning curve, which might initially slow processes until employees adapt.
3.3 Adoption or Engagement Rate
What It Means: The percentage of staff or customers who use the AI solution consistently.
Why It’s Important: Even a highly accurate model fails if no one uses it. Watching user engagement reveals acceptance (or rejection) among those it aims to serve.
Caution: Low adoption could indicate missing features, poor training, or a mismatch with users’ routines.
3.4 Error Count or Defect Reduction
What It Means: How many errors happen in a workflow, such as misclassified invoices or poor product recommendations.
Why It’s Important: Mistakes carry real costs. A small dip in defects might signal big operational savings.
Caution: Confirm that the categories used to record errors remain consistent, so you don’t measure apples vs. oranges.
4. Matching KPIs to Core Business Goals
Customer Service Scenario: If your AI pilot aims to improve support, a relevant KPI might be the average time to resolve a complaint or the percentage of positive follow-up responses.
Inventory & Supply Chain: A pilot trying to forecast demand might track how many times you ended up overstocked or understocked. That difference underscores direct cost or lost sales.
Marketing & Sales: Some SMEs run a recommendation engine pilot. A relevant KPI might be the click-through rate or conversion rate on recommended items.
Aligning these metrics with a recognized cost or revenue impact cements their value.
Suppose a complaint that once took three days now takes one, boosting satisfaction or repeat sales. That improvement can encourage additional investment in AI solutions.
5. Tracking & Communicating Progress
5.1 Data Collection Frequency
Teams must decide how often to measure. Weekly might capture meaningful shifts without the overhead of daily tracking. Bi-weekly or monthly is popular too, especially in smaller businesses.
5.2 Easy-to-Understand Dashboards
Avoid complicated analytics software if you don’t have specialized staff. A well-maintained spreadsheet or a lightweight business intelligence tool can show trends for each KPI. The goal is transparency, not fancy visuals.
5.3 Celebrating Small Wins
Early AI pilots likely show modest improvements—like a 5% better accuracy or a minor drop in processing time. Highlight these gains, because they confirm you’re learning and improving. Staff or management might see the pilot as a success that leads to bigger leaps next time.
6. Common Pitfalls
Overcomplicating Metrics: Some SMEs adopt large-scale analytics suites or track 20+ KPIs. This often overwhelms the team or muddies the focus on real progress.
Ignoring the Human Element: No matter how good the system is, if employees skip it or input sloppy data, KPI readings become useless.
Setting Unreasonable Targets: A brand-new AI tool might not cut a task from days to seconds in one pilot. Realistic goals keep morale high.
7. Best Practices for Early KPI Success
Start with 2–3 Key Measures: More than that can lead to confusion. Pin down accuracy, time saved, or user engagement as your primary data points.
Define a Baseline: Know how your processes perform before the AI pilot. Measuring improvement is easier when you know the starting line.
Set a Review Schedule: Weekly or monthly sessions to examine KPI trends. This helps you respond quickly if something veers off course.
8. Real-World Mini-Case Examples
Case A: Customer Chatbot in a Retail SME
Pilot KPI: Chatbot accuracy for product inquiries
Initial Benchmark: 60% correct responses over two weeks
Outcome: Gradual rise to 75% after staff refined the knowledge base. This modest but clear improvement showed the pilot was on track.
Case B: Demand Forecasting in a Local Distributor
Pilot KPI: Over-order or under-order rate
Initial Benchmark: 10% discrepancy from actual demand
Outcome: Pilot brought the discrepancy to 7%, reflecting cost savings in warehousing. The team extended the pilot to more product categories.
9. Tools & Techniques for KPI Management
9.1 Lightweight Dashboards
Google Sheets, Microsoft Excel, or free versions of BI tools can handle simple visualizations—like line charts for accuracy or bar graphs for weekly adoption rates.
9.2 Alerts & Notifications
Setting up email alerts or Slack messages for notable changes (e.g., if accuracy dips below 50%) helps teams respond before problems escalate.
9.3 Collaboration Channels
Tools like Microsoft Teams, Slack, or Trello boards keep everyone aligned on KPI status updates, upcoming tasks, or flagged issues. This open communication fosters accountability.
10. Scaling KPIs for the Next Phase
10.1 Resource Utilization Thresholds
Set baseline metrics for CPU, memory, or I/O usage. When usage nears 80% of capacity for extended periods, investigate expansions or optimizations.
10.2 Gradual Feature Rollouts
Introducing advanced features or new analytics modules one at a time helps isolate resource spikes. Observing performance for each addition provides clarity on where to invest next.
10.3 Periodic Capacity Reviews
Schedule monthly or quarterly reviews to discuss upcoming demands, product expansions, or marketing campaigns. Align hardware, software, and staffing changes before user volumes leap.
11. Keeping KPI Discussions Inclusive
Bringing more eyes to your numbers sparks broader ownership. If the AI tool is for marketing, the finance team might see a pattern in cost savings. If it’s for manufacturing, the HR department might track how it affects staff workload and satisfaction. Each insight can uncover a new angle for improvement.
12. Balancing Realism & Ambition
Early KPIs shouldn’t discourage you from big dreams. They exist to anchor AI efforts in tangible progress. Try combining ambition with a step-by-step approach. Perhaps you aim for a major improvement, but track smaller, incremental gains that build momentum over time.
13. Looking Ahead
As your AI system matures, new use cases will appear. Over time, you might adopt more nuanced metrics—like lifetime customer value or multi-stage supply chain efficiency. Early pilots lay the groundwork, showing how you can measure AI’s influence one project at a time.
15. Conclusion: The Power of Starting Simple
Early KPIs give your AI pilot direction and transparency. They’re a guidepost, not an end in themselves. By choosing straightforward, meaningful measurements, you ensure your pilot aligns with business realities. Team morale rises when they see quick evidence of improvement, even if it’s modest at first. That’s how AI gains traction in small and medium-sized enterprises—through stepwise validation and confidence in results.
To define a set of KPIs tailored to your SME’s unique challenges, visit HIGTM.com, booking a Private KPI Alignment Session can help set the perfect metrics for your AI pilot, ensuring your team sees clear value from day one.
Turn AI into ROI — Win Faster with HIGTM.
Consult with us to discuss how to manage and grow your business operations with AI.
© 2025 HIGTM. All rights reserved.