22. Budgeting for AI: A Practical Guide for Small and Medium-Sized Enterprises
Adopting Artificial Intelligence (AI) is no longer a luxury reserved for large corporations. Today, small and medium-sized enterprises (SMEs) can leverage AI to gain competitive advantage, optimize day-to-day tasks, and open new revenue streams. However, many SMEs hesitate due to cost concerns—unsure how to structure a budget that balances affordability and impact. This comprehensive guide reveals how to plan, fund, and manage AI spending so you can unlock real value without risking your bottom line.
Q1: FOUNDATIONS OF AI IN SME MANAGEMENT - CHAPTER 1 (DAYS 1–31): CORE AI CONCEPTS & VALUE PROPOSITION
Gary Stoyanov PhD
1/22/20256 min read
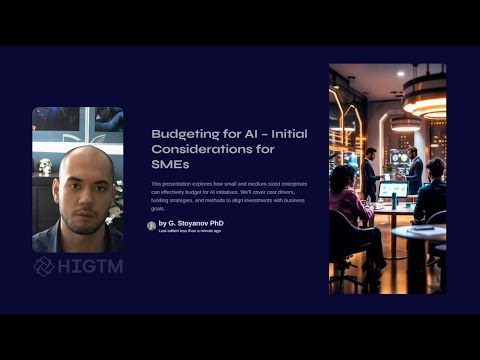
1. Why Budgeting for AI Matters
1.1 Aligning Investment with Strategic Goals
Before diving into AI, it’s crucial to clarify your broader business objectives. Are you aiming to reduce operational costs, improve customer engagement, or innovate new products? Having a strategic lens ensures that every dollar you spend on AI ties directly to measurable goals.
1.2 Avoiding the “AI Money Pit”
Without a solid budget, AI projects can spiral in scope and expense—leading to half-finished pilots or tools that never see full adoption. A clear financial plan helps you deploy resources more efficiently and minimize wasted efforts.
1.3 Building Stakeholder Confidence
For SMEs, convincing internal stakeholders or external investors that AI is a smart move often hinges on showing a well-thought-out budget. Demonstrating how and why you’ll spend funds fosters trust and underscores your commitment to ROI.
2. Identifying Key Cost Drivers
Several factors shape your AI budget. Mapping these out helps you forecast short- and long-term spending.
2.1 Software & Tooling
Cloud Services: Many AI applications run on cloud platforms like AWS, Azure, or Google Cloud. You’ll pay for usage (compute, storage, networking) which can scale up or down monthly.
Licensing Fees: Commercial AI libraries or software solutions may involve per-user or subscription charges.
Open Source vs. Proprietary: Although open-source frameworks like TensorFlow or PyTorch have no licensing costs, you still spend on hosting, maintenance, and in-house expertise.
2.2 Hardware & Infrastructure
On-Premises Servers: For SMEs opting for on-prem AI, purchasing servers or GPUs requires a significant capital outlay.
Edge Devices or Specialized Hardware: Certain use cases (e.g., computer vision at remote locations) might demand edge computing setups, adding cost for specialized hardware.
Bandwidth & Storage: As data volumes grow, you might need higher bandwidth or expanded storage capacity, especially for real-time analytics.
2.3 Talent & Training
Hiring Data Scientists & Engineers: Skilled AI professionals can command premium salaries. Alternatively, you might invest in consultants or outsourced development teams.
Upskilling Current Staff: Workshops, online courses, and certification programs can be cost-effective ways to empower existing employees.
Ongoing Team Support: Even with a talented team, AI is an iterative process—requiring model updates, debugging, and further experimentation.
2.4 Data Management & Labeling
Data Cleaning: Ensuring data accuracy often involves manual checks or specialized scripts—a time-intensive process.
Labeling Services: For supervised learning tasks like image recognition, you may need large quantities of labeled data.
Data Integration: Merging diverse data sources (e.g., ERP, CRM, spreadsheets) can involve custom connectors or specialized middleware, adding to your budget.
2.5 Maintenance & Operational Costs
Model Retraining: AI models degrade over time as real-world data changes. Allocating resources for regular model refreshes is crucial.
Bug Fixes & User Support: Post-deployment issues or user feedback often require dedicated budget lines.
Software & Security Updates: AI tools need continuous patching for security and compatibility, especially if external APIs are used.
3. Mapping Budget to Business Value
Value-based budgeting focuses on directing AI spending toward the areas with the highest potential return or strategic importance.
3.1 Prioritizing High-Impact Use Cases
Start by identifying two or three AI projects that directly tackle your biggest challenges—like reducing customer churn or automating a labor-intensive process. By aligning budget with these priorities, you establish quick wins and demonstrate ROI early.
3.2 Phased Investments
Rather than allocating a lump sum to a single, large-scale AI project, incremental spending can validate each phase. For instance:
Pilot – Low-cost proof of concept
Scale Up – Additional funds if early results look promising
Ongoing Expansion – Long-term budget line for iterative improvements
3.3 Opportunity Costs & Trade-Offs
Every dollar spent on AI is a dollar not spent elsewhere. Evaluate whether the anticipated benefits (e.g., cost savings, new products, or faster sales cycles) surpass the potential gains from other investments, such as marketing or new hires in different roles.
4. Funding Approaches for SMEs
4.1 Reallocating Existing Budgets
Check current allocations—like IT or R&D budgets—to see if you can redirect a portion toward AI. This option requires no external funding but might affect other initiatives.
4.2 Grants & Public Incentives
Many regions offer innovation grants, tax credits, or subsidies encouraging SMEs to adopt AI. Explore local economic development agencies or federal tech initiatives. These can significantly offset costs but often require structured proposals and compliance with program terms.
4.3 Investors & Venture Capital
If your AI solution has strong growth potential or a scalable market, pitching to angel investors or VCs could secure capital. Keep in mind that this route might involve equity stakes or additional pressure for rapid growth.
4.4 Partnerships or Cost-Sharing
Teaming up with a compatible SME or tech vendor might enable shared infrastructure or development costs. This approach can distribute financial risk while giving each party access to specialized expertise.
4.5 Bank Loans & Leasing
For on-premises hardware, you might lease servers or rely on small business loans. This spreads capital expenses over time, easing initial budget strain.
5. Resource Allocation and Planning
5.1 Creating a Line-Item Budget
Break down each project into line items—software licenses, hardware, staff, contingencies. A granular view ensures transparency and makes it easier to cut or add elements without derailing the entire plan.
5.2 Cost Controls & Monitoring
Usage Alerts – In the cloud, set up monitoring dashboards to watch for unexpected usage spikes.
Scheduled Reviews – Meet monthly or quarterly to compare actual spend to budgeted amounts.
Dashboard Reporting – Visualize budget vs. actual in real time, adjusting resources promptly if needed.
5.3 Building a Contingency Fund
Even small AI initiatives can encounter unexpected costs. Reserving about 10–20% of your AI budget as a contingency can keep the project afloat if you face data quality issues, need a specialized consultant, or decide to scale more rapidly.
6. Mitigating Risk and Measuring ROI
6.1 Pilot Projects with Clear KPIs
Launching a pilot with concrete metrics—like 10% cost reduction or 15% increase in leads—validates your approach before full-scale deployment. If the pilot fails to meet targets, you can pivot or reallocate funds.
6.2 Scenario Planning
Develop best-, worst-, and moderate-case scenarios. For instance, if a competitor releases a similar solution first, or if your chosen AI vendor discontinues a key feature. Scenario planning helps you prepare financially and strategically.
6.3 Transparent Stakeholder Communication
Regular updates to partners, employees, or external funders reduce misunderstandings. Show them how the budget is tracking against the original plan and highlight any course corrections.
6.4 Evaluating Post-Launch Success
After you deploy an AI model or tool, measure improvements in speed, cost, accuracy, or customer satisfaction. Compare these metrics against the initial budget to confirm you’re on track. Document lessons learned—both wins and setbacks—to refine future AI spending plans.
7. Common Budgeting Pitfalls to Avoid
Underestimating Maintenance
Even if your pilot is successful, you need ongoing funds for bug fixes, hardware refreshes, or user support.
Ignoring Data Quality
If you skip data cleaning or integration costs, you risk inaccurate model outputs and rework expenses.
Lack of ROI Tracking
Failing to define tangible KPIs can lead to inflated budgets without evidence of results.
Insufficient Training
Adopting AI requires staff readiness. Neglecting training budgets leads to underutilized tools and frustrated employees.
8. Balancing Short-Term Wins with Long-Term Strategy
8.1 Quick Returns vs. Foundational Investments
Some AI projects yield fast payoffs (e.g., automating invoice processing), while others (like advanced predictive analytics) require deeper investment and more time. Mix these approaches to maintain stakeholder enthusiasm while building a robust AI environment for the future.
8.2 Renewing the Budget Yearly
Review your AI expenditures and outcomes annually. If certain tools or vendors no longer align with your growth, reallocate those funds. Keep your budget dynamic to accommodate changes in technology or market conditions.
8.3 Building Competitive Differentiation
Successful AI initiatives can significantly differentiate SMEs from their slower-moving competitors. By consistently budgeting for improvement—enhancing customer experiences, refining operations, or expanding product lines—you set a foundation for sustained growth.
9. Real-Life SME Case Studies
9.1 Retail Startup
This small online store allocated $5,000 for a three-month AI pilot focusing on product recommendations. After proving a 12% increase in average order value, they reinvested an additional $8,000 for fully integrating these recommendations across their eCommerce platform—scoring a 20% boost in sales.
9.2 Local Manufacturer
A mid-sized plant dedicated $30,000 to predictive maintenance hardware and analytics software. Although up-front costs were steep, the manufacturer saw a 25% drop in unplanned downtime within six months, recouping the investment within a year.
9.3 Professional Services Firm
This consultancy set aside $10,000 for chatbot tech to handle routine client inquiries. By automating 60% of initial interactions, it freed up consultants to focus on high-value tasks, leading to an overall revenue increase of 15%.
10. Summary: Crafting an Effective AI Budget
Budgeting for AI is about striking the right balance between immediate feasibility and future-focused expansion. For many SMEs, the sweet spot involves:
Identifying the highest-value use cases to tackle first.
Mixing internal training with possible external expertise to build a well-rounded team.
Monitoring spending and performance in short cycles (monthly or quarterly).
Adapting quickly if metrics indicate you’re overspending or underutilizing certain tools.
By aligning each dollar with clear strategic outcomes—like cost reduction, revenue growth, or enhanced service—you ensure AI spending becomes an investment rather than an expense.
A well-planned AI budget safeguards finances and sets the stage for rapid, sustainable gains. With the right mix of phased allocation, strategic pilots, and ongoing evaluation, SMEs of any size can confidently adopt AI.
Ready to create a tailored AI budget?
Contact HIGTM for a Private Consultation.
We’ll guide you through cost evaluation, phased rollouts, and ROI-focused strategies—ensuring each dollar you spend on AI translates into meaningful business impact.
Turn AI into ROI — Win Faster with HIGTM.
Consult with us to discuss how to manage and grow your business operations with AI.
© 2025 HIGTM. All rights reserved.