19. Data Collection Essentials: How SMEs Can Gather Accurate, Relevant Data for AI and Analytics
While large organizations often have extensive infrastructure for data gathering, small and medium-sized enterprises (SMEs) can find it challenging to establish robust processes. This article offers a comprehensive roadmap for SMEs to collect high-quality information that truly serves their strategic goals. We’ll explore why data collection matters, common pitfalls, and step-by-step practices to ensure your data fuels valuable insights rather than misleading reports.
Q1: FOUNDATIONS OF AI IN SME MANAGEMENT - CHAPTER 1 (DAYS 1–31): CORE AI CONCEPTS & VALUE PROPOSITION
Gary Stoyanov PhD
1/19/20255 min read
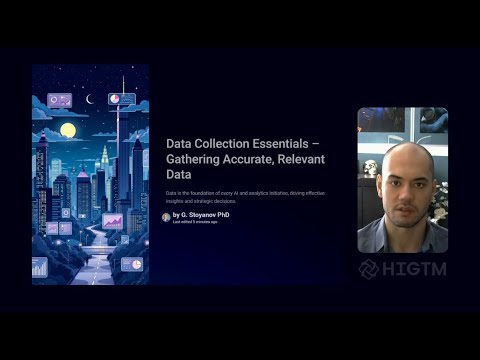
1. Why Data Collection Matters
1.1 The Foundation of AI and Analytics
At its core, any analytics or AI model is only as strong as the data it’s built upon. A predictive model without reliable historical data is like a puzzle missing key pieces—conclusions become guesswork rather than facts.
1.2 Reducing Risk
Poor data can lead to skewed forecasts, misguided investment decisions, or inaccurate customer segmentation. For SMEs with limited resources, even a single bad decision can have a lasting impact on profitability and brand reputation.
1.3 Enabling Scalability
When data collection processes are well-structured and standardized, it’s easier to integrate new tools or scale existing AI solutions. This adaptability is crucial as your business matures and faces more complex challenges.
2. Common Pitfalls in Data Gathering
2.1 Inconsistent Data Entry
Different departments often use various naming conventions or data input methods. For example, one team might label customers by ID, while another labels them by name or email. This inconsistency leads to duplicates, confusion, and alignment issues.
2.2 Legacy Systems
Many SMEs rely on outdated software—like legacy ERP or CRM systems—that lack modern integration capabilities. These older systems can be prone to data duplication or data that isn’t refreshed in real-time.
2.3 Collecting Excess Data
The lure of “collect everything” can cause businesses to hoard information they don’t need. Storing massive amounts of data without clarity on its use raises storage costs, complicates compliance, and makes analysis more difficult.
2.4 Skipping Validation & Cleaning
Data should be checked for errors, missing fields, or outdated entries. Skipping this step means your reports or AI models might rely on inaccurate or stale information.
3. The Principles of Accurate, Relevant Data
3.1 Define Clear Objectives
Before gathering data, align your collection efforts with specific business outcomes. Examples include:
Market Expansion: Collect data on customer buying behaviors or potential new regions.
Operational Efficiency: Gather metrics on production line outputs, supply chain logistics, or staffing hours.
Customer Retention: Track user activity, support interactions, and satisfaction scores.
By defining your objectives, you ensure that each piece of data serves a practical purpose.
3.2 Consistency in Standards
Consistency starts with setting standardized naming conventions, data formats (e.g., CSV vs. Excel), and metadata rules. Document these guidelines in a data dictionary accessible to all relevant teams.
3.3 Quality Control Processes
The phrase “garbage in, garbage out” rings especially true for data. Regular audits, validation scripts, and real-time checks can pinpoint anomalies before they contaminate larger datasets.
3.4 Compliance & Privacy
From GDPR in the EU to CCPA in California, data protection laws are stricter than ever. Even if your SME isn’t based in these regions, having a privacy-first approach builds trust with customers and future-proofs your operations.
3.5 Timely Updates
Data ages quickly. Inventory levels, customer preferences, and competitor moves can shift in days or even hours. A robust data pipeline updates information frequently, enabling more agile decision-making.
4. Practical Strategies & Best Practices
4.1 Start with a Data Inventory
Identify Data Sources: List every place your data comes from—online forms, POS systems, social media analytics, etc.
Ownership & Accountability: Assign a data owner for each source, ensuring someone is responsible for its accuracy and timeliness.
4.2 Implement Automated Collection Tools
APIs & Integrations: Whenever possible, integrate software through APIs to reduce manual data entry.
IoT Sensors for Physical Environments: If you’re in manufacturing or logistics, sensor data can deliver real-time updates on machine performance or inventory levels.
Web Scraping & Bots: In regulated contexts, you can gather market data through web scraping—just be mindful of legal guidelines.
4.3 Set Data Quality KPIs
Accuracy Rate: What percentage of records are error-free?
Completeness: How many records lack required fields?
Duplicate Records: Track and minimize duplicates in your database.
Timeliness: Monitor how frequently critical data points are refreshed.
Reward teams when they meet or exceed these KPIs, promoting a culture that values data integrity.
4.4 Establish a Governance Framework
A formal governance framework clarifies rules around data accessibility, modification rights, and approval processes. This may include:
Role-Based Access Control (RBAC): Limit data access based on roles—finance, marketing, or HR—so employees only see what they need.
Cross-Functional Committee: A small group representing different departments to oversee governance policies.
Ongoing Audits: Regularly scheduled reviews to ensure compliance and address new data streams.
4.5 Ongoing Training & Culture
Technology is only half the battle. Employees must understand the significance of data quality. By highlighting how accurate data drives better decisions—such as accurate sales forecasts or customer sentiment analysis—you foster an environment where everyone cares about integrity.
5. Illustrative Use Cases for SMEs
5.1 Retail Scenario
An online boutique collects customer data mainly from social media ads and email sign-ups. Without consistent naming conventions, the marketing team ends up with duplicate leads. After implementing standardized forms and data-cleaning scripts, they reduce duplicates by 40%, leading to more precise marketing campaigns and a 15% increase in conversions.
5.2 Manufacturing Context
A small auto parts manufacturer automates data gathering via IoT sensors on its assembly line. This real-time feed alerts them to equipment inefficiencies. By correlating sensor data with output logs, they identify a mechanical flaw affecting 5% of products. Correcting it boosts yield and reduces waste, saving thousands of dollars monthly.
5.3 Service-Based Business
A consulting firm uses multiple CRMs inherited from past mergers. Consolidating client records into one integrated CRM ensures each consultant sees the same up-to-date client data. Cross-selling opportunities improve, and the firm estimates a 10% revenue uplift from reduced miscommunication.
6. Overcoming Common Challenges
Resistance to Change: Employees accustomed to ad-hoc data entry may resent new systems. Provide training, explain the benefits, and collect feedback.
Budget Constraints: SMEs can’t always invest in enterprise-level data tools. Focus on cost-effective or open-source solutions, prioritizing the most critical data needs.
Ongoing Maintenance: Data collection is not a one-off project. Allocate time and resources for periodic updates, staff training, and system upgrades.
7. Future-Proofing Your Data Collection
7.1 Scaling with AI and Automation
As you grow, advanced AI applications like predictive analytics or natural language processing (NLP) might become relevant. By laying a data-first foundation now, you streamline future integration efforts.
7.2 Cloud-Based Solutions
Migrating data to secure, scalable cloud platforms can solve storage limits and provide easy global access. Reputable providers also help with compliance and data encryption.
7.3 Continuous Improvement
Schedule regular check-ins—monthly or quarterly—where your data committee reviews the status of data quality KPIs, new data sources, and any required training updates.
8. Overcoming Organizational Resistance
Executive Alignment: Present a clear ROI for ethical AI—such as safeguarding brand reputation or reducing compliance costs—to leadership.
Employee Buy-In: Involve staff early. Demonstrate that ethical AI can reduce mundane tasks and let them focus on creative or strategic work.
Cultural Shift: Celebrate small successes, like detecting a potential bias in a pilot test. Publicly recognizing these efforts shows the organization values responsible innovation.
10. Conclusion: Data collection is often overlooked
Yet, gathering accurate and relevant data is what ultimately determines whether those technologies deliver real-world value or fall flat. Whether you’re facing inconsistent data entry, planning to integrate IoT sensors, or struggling to unify multiple CRMs, a strategic approach to data collection can unlock transformative results.
Ready to refine your data collection strategy?
Schedule a Private Data Assessment at HIGTM.com.
Our team will help you establish a targeted, scalable approach to collecting the data that matters—ensuring you’re well-equipped for advanced analytics and AI-driven innovation.
9. Putting It All Together
Define Objectives: Align data collection with your top business goals.
Standardize & Automate: Use consistent formats and leverage tools to reduce manual errors.
Implement Governance: Establish committees, policies, and role-based access.
Invest in Culture: Train employees to recognize how better data drives better outcomes.
Iterate & Scale: Review metrics and adapt processes as your business evolves.
By following these steps, SMEs can build a data ecosystem robust enough to support AI initiatives, adapt to changing market conditions, and unlock new growth avenues.
Turn AI into ROI — Win Faster with HIGTM.
Consult with us to discuss how to manage and grow your business operations with AI.
© 2025 HIGTM. All rights reserved.